Explore ML’s Impact on Improving Personalized Financial Planning
Explore ML’s Impact on Improving Personalized Financial Planning
#ML #Finance #Innovation
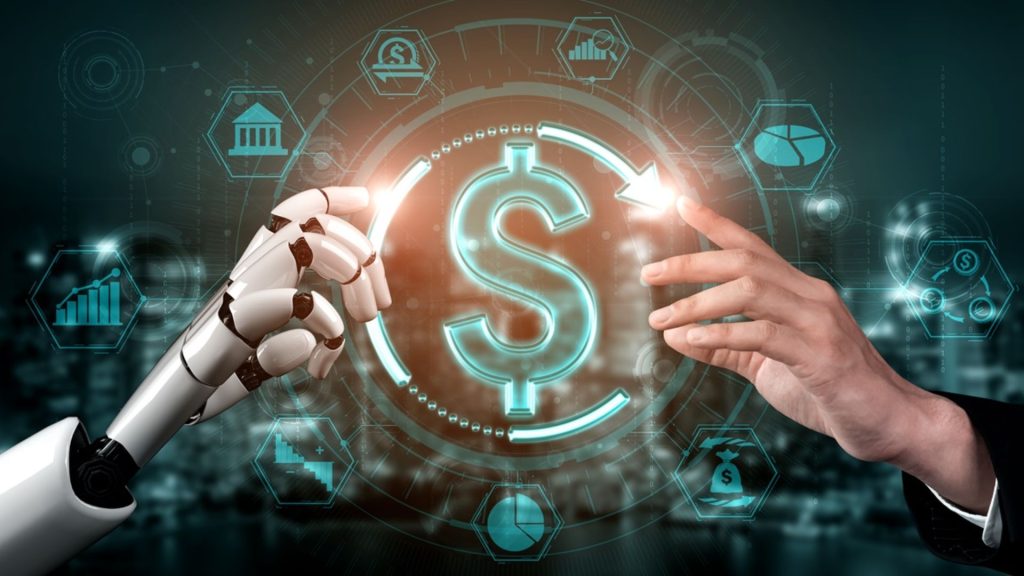
Introduction
In an increasingly complex financial landscape, individuals and organizations require guidance tailored to their unique goals, risk appetites, and life stages. Machine Learning (ML) is stepping up to meet this demand by providing data-driven, personalized financial planning solutions. By analyzing a wealth of data—ranging from spending habits and income patterns to market trends and economic indicators—ML-powered tools can craft financial strategies that align with personal objectives, helping users optimize investments, manage debts, and prepare for future needs.
This article explores how ML is revolutionizing personalized financial planning, the benefits it offers, and the future trends shaping this innovative field.
Applications of ML in Personalized Financial Planning
ML technologies integrate into multiple facets of financial planning to deliver tailored advice and actionable insights:
Goal-Based Investment Recommendations
ML models assess user profiles, financial targets, and time horizons to suggest diversified investment portfolios. This ensures that recommendations align with specific objectives—such as buying a home, saving for education, or planning retirement.
Real-Time Budgeting and Expense Tracking
Intelligent budgeting tools analyze spending patterns to identify areas of improvement. Users receive personalized tips for cutting unnecessary expenses, reallocating funds, or capitalizing on savings opportunities.
Dynamic Risk Assessment
By evaluating historical data, market volatility, and user behavior, ML-driven risk models adjust financial plans in response to economic shifts, helping clients maintain stable portfolios even in turbulent conditions.
Debt and Credit Management
ML algorithms guide users in managing credit card debts, loans, and mortgages. Personalized repayment plans, refinancing suggestions, and alerts on credit utilization keep individuals on track toward financial stability.
Predictive Retirement Planning
ML-based forecasting tools project retirement income, expenses, and shortfalls. Users can test various scenarios—like changes in savings rates or retirement ages—to find the most robust strategies for long-term security.
Benefits of ML in Personalized Financial Planning
Integrating ML into financial planning processes yields several key advantages:
- Customized Advice: ML ensures that recommendations are relevant to each user’s financial situation, goals, and preferences.
- Continuous Optimization: As personal and market conditions evolve, ML models update strategies in real-time, maintaining optimal outcomes.
- Enhanced Accessibility: Automated insights lower barriers to financial expertise, making personalized guidance available to a broader audience.
- Informed Decision-Making: Data-driven analysis reduces guesswork and emotional biases, empowering users to make more confident financial decisions.
- Cost-Effectiveness: ML-driven platforms often operate at lower costs than traditional advisors, offering affordable solutions without compromising quality.
Key ML Technologies in Financial Planning
Several ML techniques and tools drive advancements in personalized financial planning:
Regression and Forecasting Models
Regression analysis helps predict future income, expenses, and asset growth, while time-series models handle seasonal trends and economic cycles for accurate financial projections.
Classification Algorithms
Classification methods identify customer segments, categorizing users based on their risk profiles, investment knowledge, and financial behaviors. This segmentation enables targeted guidance and product recommendations.
Clustering and Unsupervised Learning
Unsupervised techniques group similar customers or expenses, revealing hidden patterns. Identifying these clusters informs more nuanced budgeting advice and investment plans.
Natural Language Processing (NLP)
NLP analyzes user interactions and queries—like questions asked to virtual assistants—to provide context-aware answers and education on financial topics, improving user engagement and trust.
Case Studies
Financial App A: Tailored Savings Plans
App A employed ML models to track user spending habits and personal goals. Users who followed the recommended savings plans saw a 20% increase in emergency fund balances and improved financial resilience.
Wealth Manager B: Personalized Investment Portfolios
Wealth Manager B integrated ML-driven asset allocation strategies, creating portfolios aligned with each client’s risk tolerance and target returns. Clients experienced more stable performance and higher satisfaction ratings.
Robo-Advisor C: Automated Retirement Planning
Robo-Advisor C’s platform leveraged ML to simulate various retirement scenarios. Customers received tailored recommendations on contribution increases, asset rebalancing, and retirement age adjustments, leading to better-prepared retirements.
Challenges and Considerations
While ML enhances personalized financial planning, several challenges must be addressed:
Data Privacy and Security
Handling sensitive financial data requires strict compliance with privacy laws, encryption standards, and secure data storage practices to maintain user trust.
Bias and Fairness
ML models trained on skewed data may produce biased recommendations. Regular audits and bias mitigation strategies ensure fairness and equitable access to financial advice.
Regulatory Compliance
The financial industry is heavily regulated. ML-driven platforms must comply with legal requirements and provide transparent explanations of their recommendations.
User Education and Trust
Encouraging users to understand the rationale behind recommendations and maintaining a human touch where needed builds confidence and fosters long-term adoption.
Future Trends in ML-Driven Financial Planning
ML’s role in personal finance is poised for further expansion and refinement:
Integration with Holistic Financial Wellness
Beyond investments and budgeting, ML solutions will encompass insurance coverage, tax strategies, and estate planning, providing comprehensive financial well-being support.
Voice and Conversational Interfaces
NLP-driven chatbots and voice assistants will offer instant guidance, making it easier for users to access insights and take action without navigating complex menus.
Personalized Financial Education
AI-driven tools will suggest educational content—such as articles, videos, or courses—based on a user’s knowledge gaps, fostering financial literacy and informed decision-making.
Predictive Impact Analysis
ML models will forecast how life events—like career changes, relocation, or inheritance—may influence financial plans, allowing users to adapt preemptively.
Conclusion
Machine Learning is reshaping personalized financial planning by delivering timely, relevant, and adaptable advice. As data grows more abundant and models become more sophisticated, these solutions will only improve, making financial expertise accessible to a broader audience and helping individuals navigate their financial journeys with confidence.
Embracing ML-driven personalized financial planning positions both individuals and financial institutions at the forefront of innovation, driving better outcomes and empowering customers to achieve their long-term financial objectives.