How Machine Learning is Advancing Precision Agriculture
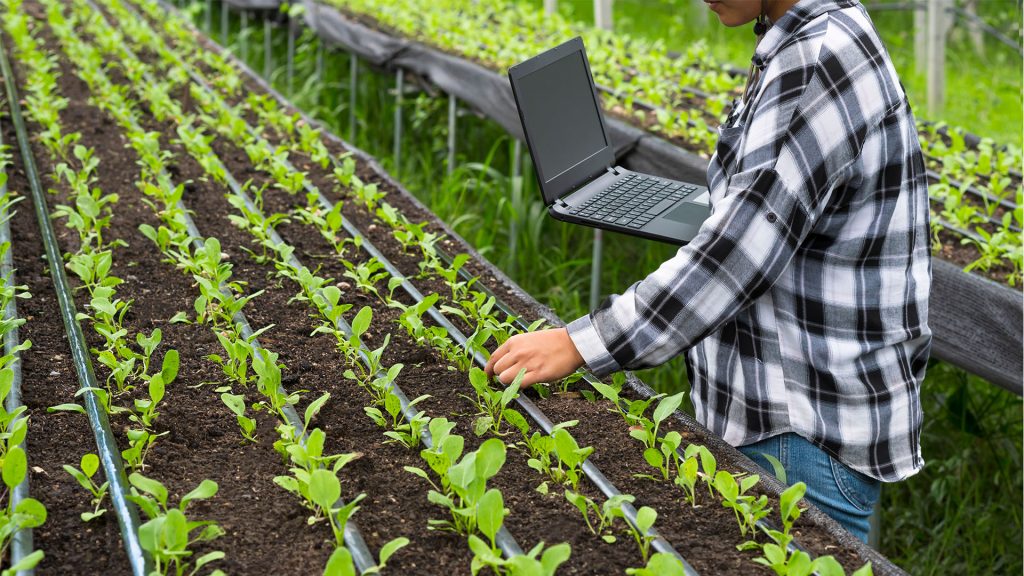
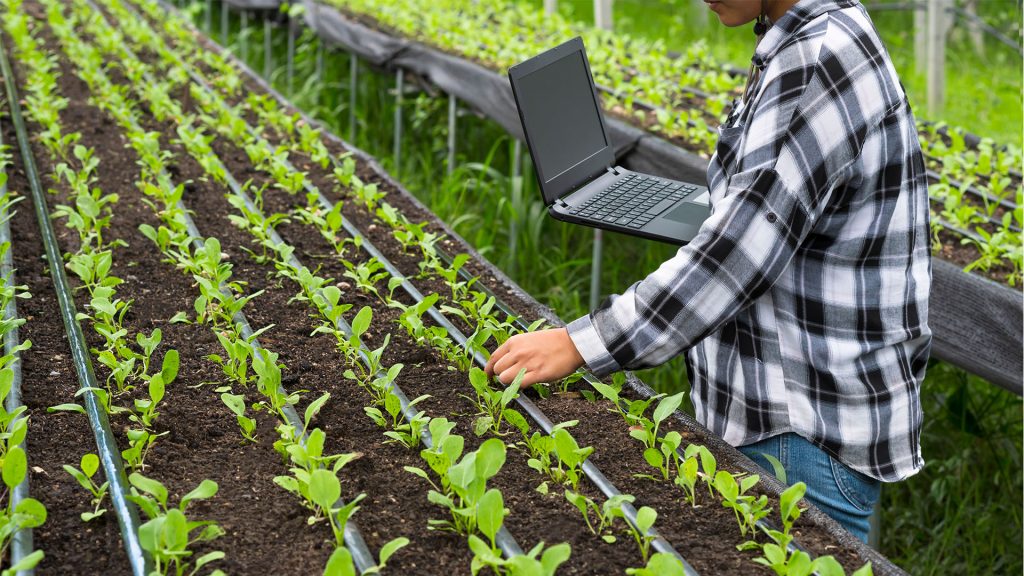
Agriculture has always been the backbone of human civilization, but the industry faces numerous challenges today, including climate change, soil degradation, and increasing demand for food. To tackle these issues, precision agriculture has emerged as a revolutionary approach, leveraging advanced technologies like Machine Learning (ML) to optimize farming practices and improve sustainability. This article explores how ML is transforming precision agriculture, providing real-world examples and highlighting its benefits.
Introduction
Precision agriculture, also known as smart farming, integrates technology to monitor and manage crops at a micro level. This approach enables farmers to optimize their inputs and improve crop yields while minimizing environmental impact. Machine Learning (ML), a subset of artificial intelligence, plays a crucial role in this transformation by analyzing vast amounts of data to provide actionable insights.
Understanding Precision Agriculture
Precision agriculture involves the use of technology to collect and analyze data on various aspects of farming, including soil conditions, weather patterns, and crop health. This data-driven approach allows farmers to make informed decisions, leading to increased efficiency and productivity.
Key Components of Precision Agriculture
- Remote Sensing: Using satellites and drones to capture images and data from fields.
- IoT Devices: Sensors placed in fields to monitor soil moisture, temperature, and other conditions.
- Data Analytics: Analyzing collected data to identify patterns and trends.
- Automation: Using automated machinery and systems to perform tasks such as planting, watering, and harvesting.
The Role of Machine Learning in Precision Agriculture
Machine Learning enhances precision agriculture by processing large datasets to identify patterns and make predictions. These capabilities help farmers optimize their practices and respond proactively to potential issues.
Key ML Techniques in Agriculture
- Supervised Learning: Training models on labeled data to make predictions.
- Unsupervised Learning: Identifying patterns in data without predefined labels.
- Reinforcement Learning: Learning optimal actions through trial and error.
- Deep Learning: Using neural networks to analyze complex data and make predictions.
Key Applications of ML in Agriculture
Machine Learning has numerous applications in precision agriculture, including:
Crop Monitoring and Disease Detection
ML algorithms analyze data from remote sensing and IoT devices to monitor crop health and detect diseases early. This enables farmers to take preventive measures and reduce crop losses.
Yield Prediction
By analyzing historical data and current conditions, ML models can predict crop yields with high accuracy. This helps farmers plan their harvests and manage resources more effectively.
Soil Health Analysis
ML models analyze soil data to determine nutrient levels and recommend appropriate fertilizers and treatments. This ensures optimal soil health and improves crop productivity.
Precision Irrigation
ML algorithms use data from soil moisture sensors and weather forecasts to optimize irrigation schedules. This reduces water usage and ensures crops receive the right amount of water at the right time.
Pest and Weed Management
ML models analyze data to identify pest and weed infestations early. This allows farmers to implement targeted treatments and minimize the use of pesticides.
Real-World Examples
Here are 20 real-world examples of how Machine Learning is advancing precision agriculture:
1. Blue River Technology
Blue River Technology, a subsidiary of John Deere, uses ML to develop smart machines for precision agriculture. Their “See & Spray” technology uses computer vision and ML to identify and target weeds, reducing herbicide usage. Read more
2. FarmLogs
FarmLogs leverages ML to provide farmers with insights on crop health, weather conditions, and soil quality. Their platform helps farmers make data-driven decisions to optimize their practices. Read more
3. Descartes Labs
Descartes Labs uses satellite imagery and ML to monitor crop health and predict yields. Their platform provides real-time insights to help farmers manage their fields more effectively. Read more
4. Climate FieldView
Climate FieldView, developed by The Climate Corporation, uses ML to analyze field data and provide insights on crop performance. Their platform helps farmers optimize planting, irrigation, and fertilization. Read more
5. Granular
Granular uses ML to provide farmers with insights on field operations, financials, and crop performance. Their platform helps farmers increase efficiency and profitability. Read more
6. Taranis
Taranis uses ML and aerial imagery to monitor crop health and detect diseases early. Their platform provides high-resolution images and actionable insights to help farmers protect their crops. Read more
7. CropX
CropX uses ML to optimize irrigation by analyzing soil moisture data. Their platform provides recommendations on when and how much to water, helping farmers save water and improve yields. Read more
8. Prospera
Prospera uses ML to analyze data from field sensors and drones, providing insights on crop health and growth. Their platform helps farmers optimize their practices and increase productivity. Read more
9. aWhere
aWhere uses ML to provide weather data and agricultural insights to farmers. Their platform helps farmers make informed decisions on planting, irrigation, and pest management. Read more
10. Gamaya
Gamaya uses hyperspectral imaging and ML to monitor crop health and detect diseases early. Their platform provides detailed insights to help farmers protect their crops. Read more
11. Arable
Arable uses ML to analyze data from weather stations and sensors, providing insights on crop health and growth. Their platform helps farmers optimize irrigation and fertilization. Read more
12. OneSoil
OneSoil uses ML to analyze satellite imagery and provide insights on field performance. Their platform helps farmers monitor crop health and optimize their practices. Read more
13. Plantix
Plantix uses ML to identify plant diseases and pests through a mobile app. Farmers can take pictures of their crops, and the app provides diagnoses and treatment recommendations. Read more
14. AgEagle
AgEagle uses drones and ML to monitor crop health and detect issues early. Their platform provides high-resolution images and actionable insights to help farmers manage their fields. Read more
15. DroneDeploy
DroneDeploy uses ML to analyze aerial imagery and provide insights on crop health and field conditions. Their platform helps farmers optimize planting, irrigation, and pest management. Read more
16. Trace Genomics
Trace Genomics uses ML to analyze soil samples and provide insights on soil health. Their platform helps farmers optimize fertilization and improve crop yields. Read more
17. xFarm
xFarm uses ML to provide farmers with insights on field operations, crop health, and weather conditions. Their platform helps farmers increase efficiency and productivity. Read more
18. PEAT
PEAT uses ML to identify plant diseases and pests through a mobile app called Plantix. Farmers can take pictures of their crops, and the app provides diagnoses and treatment recommendations. Read more
19. SkySquirrel Technologies
SkySquirrel Technologies uses drones and ML to monitor vineyard health and detect diseases early. Their platform provides high-resolution images and actionable insights to help vineyard managers protect their crops. Read more
20. Agremo
Agremo uses ML to analyze aerial imagery and provide insights on crop health and field conditions. Their platform helps farmers optimize planting, irrigation, and pest management. Read more
Implementing ML in Precision Agriculture
Step 1: Define Objectives
Before implementing ML, it’s crucial to define your agricultural objectives. Whether it’s improving crop yields, reducing water usage, or detecting diseases early, clear objectives will guide the implementation process.
Step 2: Collect and Analyze Data
Data is the foundation of ML. Collect data from various sources, including sensors, satellite imagery, and historical records. Use ML algorithms to analyze this data and derive actionable insights.
Step 3: Develop Predictive Models
Build predictive models to forecast trends and scenarios. These models will help farmers anticipate future needs and make informed decisions.
Step 4: Optimize Farming Practices
Leverage ML to optimize farming practices, such as irrigation, fertilization, and pest management. Automation and real-time monitoring can enhance efficiency and effectiveness.
Step 5: Monitor and Adjust
Regularly monitor the performance of ML-powered farming strategies. Use analytics tools to measure success and make data-driven adjustments to optimize practices.
Challenges and Considerations
Data Privacy and Security
The use of ML in agriculture raises concerns about data privacy and security. Ensure compliance with data protection regulations and implement robust security measures to safeguard data.
Algorithm Bias
ML algorithms can exhibit bias, leading to unfair or inaccurate outcomes. Regularly audit and refine algorithms to mitigate bias and ensure fairness.
Integration with Existing Systems
Integrating ML with existing agricultural systems can be challenging. Ensure seamless integration to maximize the benefits of these technologies.
Continuous Learning
ML models require continuous learning and updates to remain effective. Regularly update models with new data to ensure accuracy and relevance.
The Future of ML in Precision Agriculture
The future of ML in precision agriculture is promising. As technology advances, these tools will become even more sophisticated, offering deeper insights and more precise solutions. The integration of ML will lead to smarter, more sustainable farming practices that can adapt to changing needs and challenges.
Emerging Trends
- AI-Driven Sustainability: ML will play a crucial role in promoting sustainability and environmental protection.
- Autonomous Farming: The development of autonomous machinery and robots will enhance farming efficiency.
- Advanced Crop Monitoring: Improved sensors and imaging technologies will provide more detailed insights into crop health.
Conclusion
Machine Learning is transforming precision agriculture, offering powerful tools to optimize farming practices and improve sustainability. By leveraging ML, farmers can address complex challenges, enhance productivity, and reduce environmental impact. As ML continues to evolve, its impact on agriculture will only grow, paving the way for innovative and sustainable farming solutions.
For more insights and resources on Machine Learning in precision agriculture, visit TGI.