Discover How ML is Advancing Natural Language Understanding
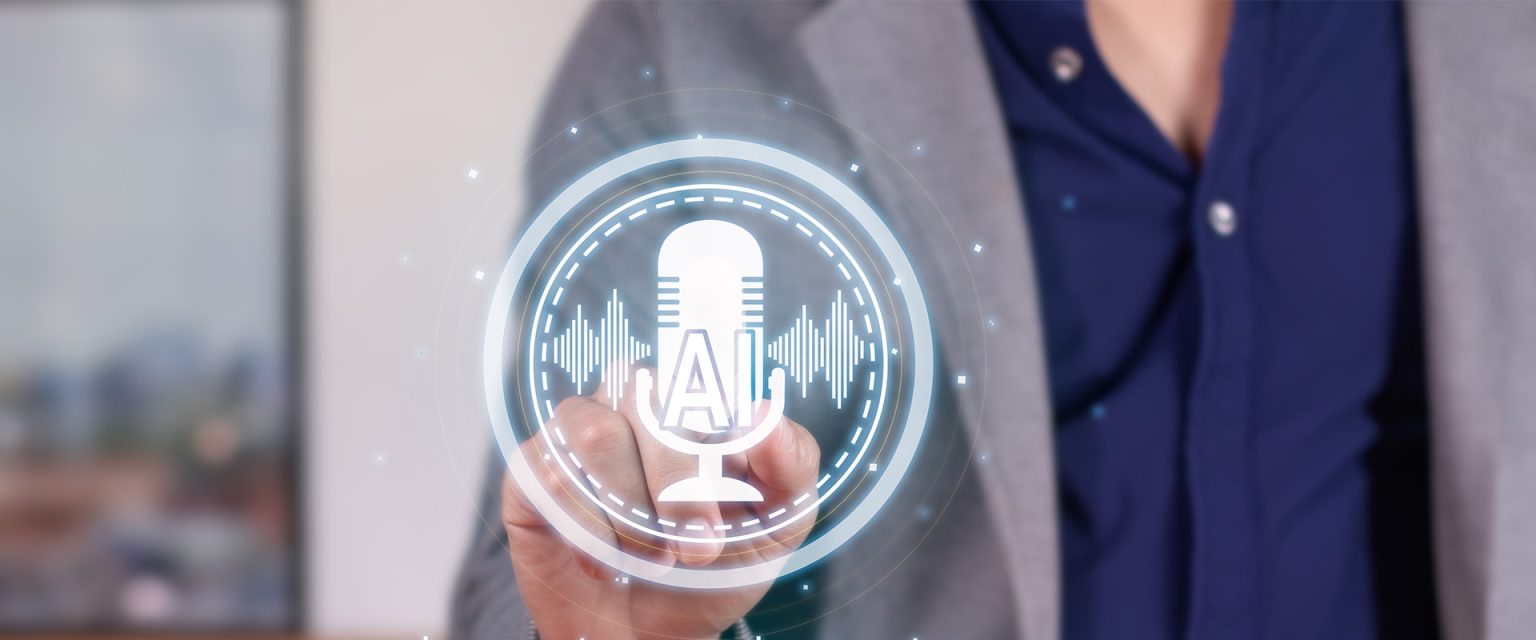
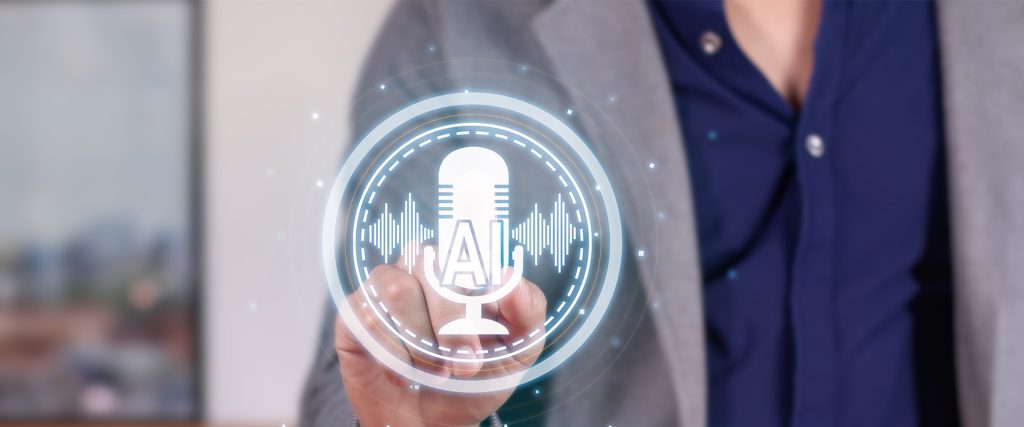
How Machine Learning is Advancing Natural Language Understanding
Machine Learning (ML) is a cornerstone of advancements in Natural Language Processing (NLP), driving innovations that allow computers to understand, interpret, and generate human language with unprecedented accuracy. From sentiment analysis to machine translation, ML is reshaping how we interact with technology. This article explores the profound impact of ML on natural language understanding (NLU), supported by real-world examples and detailed insights.
Table of Contents
- Introduction to Natural Language Understanding
- The Role of Machine Learning in NLP
- Key ML Techniques in NLU
- Applications of NLU
- Sentiment Analysis
- Chatbots and Virtual Assistants
- Machine Translation
- Text Summarization
- Speech Recognition
- Real-World Examples of ML in NLU
- Challenges and Limitations
- Future Trends and Developments
- Conclusion
Introduction to Natural Language Understanding
Natural Language Understanding (NLU) is a subfield of NLP that focuses on enabling machines to comprehend and interpret human language in a meaningful way. NLU involves the ability to understand context, disambiguate meaning, and generate appropriate responses. As technology progresses, the integration of ML in NLU is becoming increasingly sophisticated, making it possible for machines to interact with humans in more natural and intuitive ways.
The Role of Machine Learning in NLP
Machine Learning algorithms are integral to the development of NLP applications. By learning from large datasets, ML models can identify patterns and make predictions, enhancing the machine’s ability to understand and generate human language. These models continuously improve as they are exposed to more data, enabling more accurate and contextually relevant language processing.
Key ML Techniques in NLU
Deep Learning
Deep learning, a subset of ML, involves neural networks with multiple layers that can learn hierarchical representations of data. In NLU, deep learning models, such as recurrent neural networks (RNNs) and transformers, have been highly effective in processing sequential data and understanding context.
Google’s BERT (Bidirectional Encoder Representations from Transformers) is a deep learning model that has significantly improved the accuracy of various NLP tasks, such as question answering and sentiment analysis. Read more.
Transfer Learning
Transfer learning involves pre-training a model on a large dataset and then fine-tuning it on a smaller, task-specific dataset. This technique has revolutionized NLP by allowing models to leverage knowledge from general language understanding to perform specific tasks more effectively.
OpenAI’s GPT-3 (Generative Pre-trained Transformer 3) uses transfer learning to generate human-like text, powering applications such as chatbots and content creation tools. Read more.
Reinforcement Learning
Reinforcement learning involves training models to make a sequence of decisions by rewarding desired behaviors. In NLU, reinforcement learning is used to optimize dialogue systems and improve the interaction between machines and humans.
DeepMind’s AlphaStar uses reinforcement learning to improve real-time strategy game play, demonstrating how these techniques can be adapted for complex decision-making tasks in NLU. Read more.
Applications of NLU
Sentiment Analysis
Sentiment analysis involves determining the emotional tone behind a piece of text. ML models can classify text as positive, negative, or neutral, providing valuable insights for businesses and organizations.
Amazon uses sentiment analysis to analyze customer reviews and improve product recommendations. Read more.
Chatbots and Virtual Assistants
AI-powered chatbots and virtual assistants rely on NLU to understand user queries and provide relevant responses. These systems are used in customer service, personal assistance, and information retrieval.
Apple’s Siri uses NLU to interpret voice commands and perform tasks such as setting reminders and answering questions. Read more.
Machine Translation
Machine translation involves converting text from one language to another. ML models improve the accuracy and fluency of translations by learning from vast multilingual datasets.
Google Translate uses neural machine translation to provide high-quality translations for over 100 languages. Read more.
Text Summarization
Text summarization algorithms condense long documents into shorter summaries, making it easier to digest large volumes of information. ML models can generate extractive or abstractive summaries based on the context and content.
Summa uses ML to provide automatic text summarization services, helping users quickly understand the main points of lengthy documents. Read more.
Speech Recognition
Speech recognition technology converts spoken language into text. NLU models enhance the accuracy of transcription by understanding context and differentiating between similar-sounding words.
Google’s Speech-to-Text API uses ML to provide real-time speech recognition with high accuracy. Read more.
Real-World Examples of ML in NLU
1. IBM Watson – Cognitive Computing
IBM Watson uses ML to understand natural language queries and provide accurate answers across various domains, including healthcare and finance. Read more.
2. Grammarly – Writing Enhancement
Grammarly uses ML to provide real-time grammar and style suggestions, helping users improve their writing. The platform’s NLU capabilities enable it to understand context and provide relevant recommendations. Read more.
3. Microsoft Azure – Cognitive Services
Microsoft Azure’s Cognitive Services offer a suite of NLU tools for tasks such as sentiment analysis, language detection, and key phrase extraction. Read more.
4. Amazon Comprehend – Text Analysis
Amazon Comprehend uses ML to analyze text and extract insights such as sentiment, entities, and key phrases. The service helps businesses gain a deeper understanding of their data. Read more.
5. Facebook AI – Deep Text
Facebook’s Deep Text is an AI system that uses ML to understand the context and meaning of text in over 20 languages, enhancing user experience on the platform. Read more.
6. Salesforce Einstein – AI for CRM
Salesforce Einstein uses ML to analyze customer interactions and provide insights for improving sales and customer service. The platform’s NLU capabilities enable it to understand and respond to customer queries effectively. Read more.
7. Baidu – DuerOS
Baidu’s DuerOS is an AI-powered platform that uses NLU to provide voice-activated services across various smart devices. Read more.
8. Alibaba – AliMe
Alibaba’s AliMe uses ML to power its virtual assistant, handling customer service queries and providing personalized recommendations. Read more.
9. SAP – Conversational AI
SAP’s Conversational AI platform uses ML to create chatbots that understand and respond to natural language queries, enhancing business automation. Read more.
10. Zoom – Real-Time Transcription
Zoom uses ML to provide real-time transcription services during video conferences, enhancing accessibility and record-keeping. Read more.
Challenges and Limitations
Ambiguity and Context
Understanding context and resolving ambiguity in language remain significant challenges for NLU models. Words can have multiple meanings, and interpreting the correct meaning requires understanding the context.
Bias in Training Data
ML models can inherit biases present in training data, leading to unfair or inaccurate predictions. Ensuring diversity and fairness in training datasets is crucial for developing unbiased NLU systems.
Computational Resources
Training and deploying advanced NLU models require substantial computational resources, which can be a barrier for smaller organizations. Optimizing these models for efficiency is an ongoing challenge.
Future Trends and Developments
As ML technology continues to evolve, its impact on natural language understanding will only grow. Future trends may include greater integration of contextual understanding, real-time processing capabilities, and a focus on ethical AI practices.
Improved Contextual Understanding
Advancements in contextual understanding will enable NLU models to interpret language more accurately, considering nuances and subtleties in human communication.
Real-Time Language Processing
Future NLU systems will focus on real-time processing capabilities, allowing for instantaneous understanding and response in interactive applications.
Ethical AI and Fairness
Ensuring ethical AI practices and addressing biases in NLU models will be a priority, promoting fairness and equity in language understanding technologies.
Conclusion
Machine Learning is advancing natural language understanding by providing powerful tools to interpret and generate human language. From sentiment analysis and chatbots to machine translation and speech recognition, ML is transforming how we interact with technology. As the technology continues to evolve, its impact on NLU will only grow, paving the way for more innovative and effective language understanding solutions.
For more insights and updates on the latest in ML and NLP, visit TGI.