How Machine Learning Optimizes Supply Chain Logistics in E-Commerce
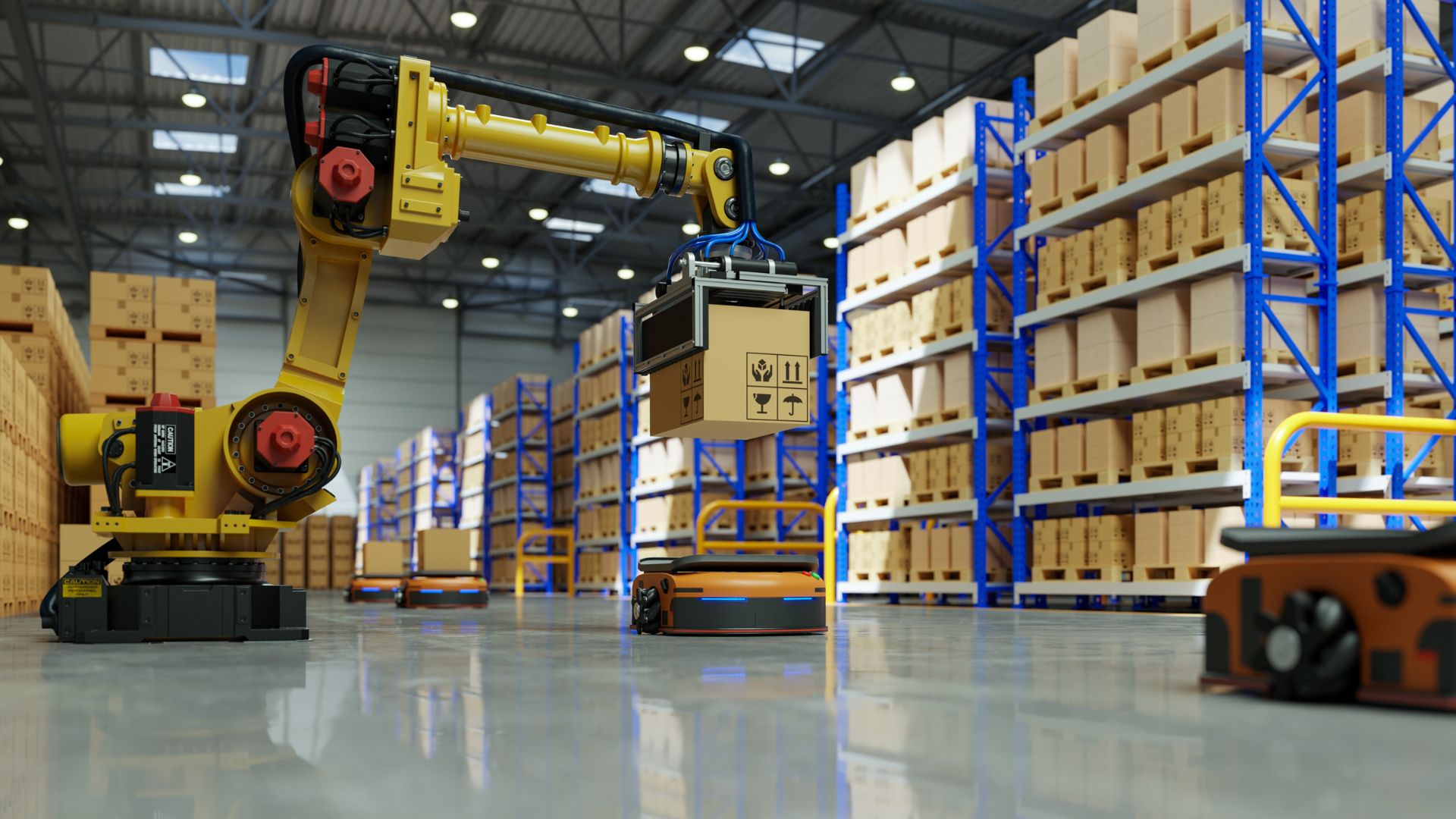
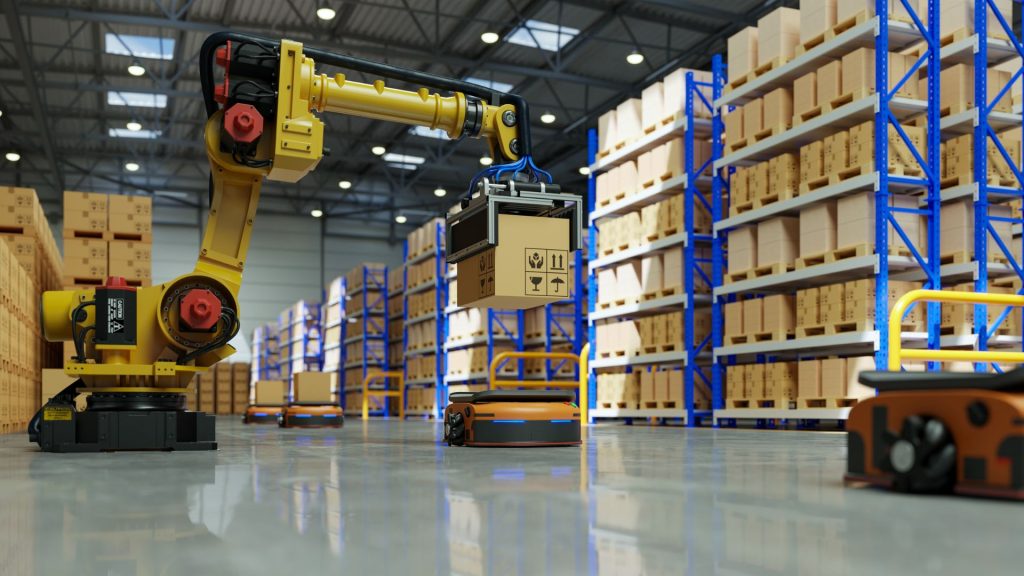
The rapid growth of e-commerce has revolutionized the global retail landscape, providing consumers with unprecedented access to products from all over the world. However, this transformation also brings significant challenges in managing the complex supply chain logistics required to meet customer expectations. Machine learning (ML), a branch of artificial intelligence (AI), has emerged as a powerful tool for optimizing various aspects of supply chain logistics, from demand forecasting to route optimization and inventory management. This article explores how ML is being utilized to enhance efficiency, reduce costs, and improve customer satisfaction in the e-commerce supply chain.
1. Demand Forecasting and Inventory Management
Accurate demand forecasting is crucial for efficient supply chain management. Overestimating demand can lead to overstocking, tying up capital in unsold products, while underestimating demand can result in stockouts and lost sales. Traditional forecasting methods often rely on historical data and may fail to account for new trends or external factors like economic shifts or seasonal events.
Machine learning algorithms, on the other hand, can analyze large datasets from multiple sources—such as sales trends, social media sentiment, weather patterns, and economic indicators—to provide more accurate demand forecasts. These algorithms continuously learn and improve as new data becomes available, allowing e-commerce businesses to better anticipate demand fluctuations and adjust their inventory levels accordingly.
By optimizing inventory management, ML reduces excess stock and minimizes the risk of stockouts, helping businesses maintain a balance between supply and demand. This leads to lower holding costs and improved customer satisfaction, as products are more likely to be available when needed.
2. Supplier Selection and Procurement Optimization
E-commerce companies often work with a diverse network of suppliers to source products. Selecting the right supplier involves evaluating factors such as price, quality, reliability, and lead times. Machine learning can assist in this process by analyzing historical supplier performance data, as well as external factors like geopolitical risks and market conditions.
ML algorithms can identify patterns and trends in supplier behavior, enabling e-commerce companies to make data-driven decisions about which suppliers to work with. These algorithms can also predict potential disruptions in the supply chain, such as delays or quality issues, and recommend alternative suppliers or strategies to mitigate risks.
Furthermore, ML can optimize procurement by automating purchase order generation and tracking, reducing the time and effort required to manage supplier relationships. By streamlining the procurement process, e-commerce companies can ensure a steady flow of products while minimizing costs.
3. Warehouse Management and Automation
Efficient warehouse management is critical to the success of e-commerce businesses, particularly those that handle a large volume of orders. Machine learning plays a key role in optimizing warehouse operations by improving inventory organization, order picking, and storage utilization.
ML-powered warehouse management systems (WMS) can analyze order patterns and inventory turnover rates to recommend the best storage locations for products, reducing the time and distance required for order picking. This not only speeds up the fulfillment process but also minimizes labor costs.
In addition to optimizing storage, ML algorithms can be used to automate repetitive tasks within the warehouse. For example, autonomous robots equipped with ML capabilities can handle tasks such as sorting, packing, and transporting goods within the facility. These robots learn from their environment and improve their efficiency over time, leading to faster and more accurate order fulfillment.
4. Route Optimization and Last-Mile Delivery
One of the most challenging aspects of e-commerce logistics is the “last mile”—the final leg of the delivery journey from a distribution center to the customer’s doorstep. Last-mile delivery is often the most expensive and time-consuming part of the supply chain, accounting for a significant portion of overall shipping costs.
Machine learning can significantly improve last-mile delivery by optimizing delivery routes and reducing delivery times. ML algorithms analyze data such as traffic conditions, weather forecasts, delivery time windows, and customer preferences to determine the most efficient routes for delivery drivers. These algorithms continuously learn from real-time data, allowing them to adjust routes dynamically in response to changing conditions.
For example, if traffic congestion or a road closure is detected, the ML system can automatically reroute the driver to avoid delays. This not only ensures faster deliveries but also reduces fuel consumption and transportation costs. In addition, ML can help allocate delivery resources more efficiently, such as determining the optimal number of delivery vehicles and drivers needed to meet demand.
5. Predictive Maintenance and Fleet Management
E-commerce companies that manage their own delivery fleets can benefit from machine learning through predictive maintenance. Predictive maintenance involves using ML algorithms to monitor the health of delivery vehicles and predict when maintenance is needed before a breakdown occurs. This helps prevent unexpected downtime and reduces repair costs.
ML algorithms analyze data from sensors embedded in vehicles—such as engine performance, fuel efficiency, and wear-and-tear indicators—to detect early signs of potential issues. By identifying patterns that indicate an impending failure, the system can recommend proactive maintenance actions, such as replacing a part or scheduling a service, before the vehicle experiences a breakdown.
In addition to predictive maintenance, ML can optimize fleet management by analyzing data such as driver behavior, fuel consumption, and delivery routes. This helps e-commerce companies improve fuel efficiency, reduce vehicle wear and tear, and enhance overall fleet performance.
6. Customer Service and Order Tracking
In the highly competitive e-commerce industry, providing exceptional customer service is essential for retaining customers and building brand loyalty. Machine learning plays a key role in enhancing the customer experience by enabling real-time order tracking, personalized recommendations, and automated customer support.
ML-powered systems can analyze customer data—such as purchase history, browsing behavior, and feedback—to provide personalized product recommendations, increasing the likelihood of repeat purchases. Additionally, ML algorithms can predict delivery times based on factors such as order volume, warehouse location, and transportation conditions, allowing customers to track their orders more accurately.
Automated customer support, powered by natural language processing (NLP) and ML, can handle common customer inquiries, such as order status, return policies, and shipping issues. This reduces the workload for human customer service representatives and provides customers with instant, accurate responses to their questions.
7. Fraud Detection and Risk Management
E-commerce businesses are often targets for fraud, such as payment fraud, account takeovers, and return fraud. Machine learning can help detect and prevent fraudulent activities by analyzing transaction data and identifying suspicious patterns.
ML algorithms can flag potentially fraudulent transactions based on factors such as unusual spending patterns, location discrepancies, and multiple failed payment attempts. By continuously learning from new data, these algorithms become more accurate over time, reducing the number of false positives and minimizing the risk of fraud.
Additionally, ML can be used to assess risks in the supply chain, such as potential disruptions due to supplier issues, transportation delays, or geopolitical factors. By proactively identifying and mitigating risks, e-commerce companies can ensure smoother operations and maintain customer trust.
Conclusion
Machine learning is transforming supply chain logistics in e-commerce by enabling businesses to optimize operations, reduce costs, and improve customer satisfaction. From demand forecasting and inventory management to last-mile delivery and fraud detection, ML provides data-driven insights that help e-commerce companies stay competitive in a rapidly evolving market.
As ML technology continues to advance, its role in optimizing supply chain logistics will only grow, offering new opportunities for innovation and efficiency. E-commerce companies that embrace ML and integrate it into their supply chain strategies will be better positioned to meet customer expectations, reduce operational costs, and succeed in the digital economy.