Explore ML’s Impact on Predictive Maintenance in Utilities
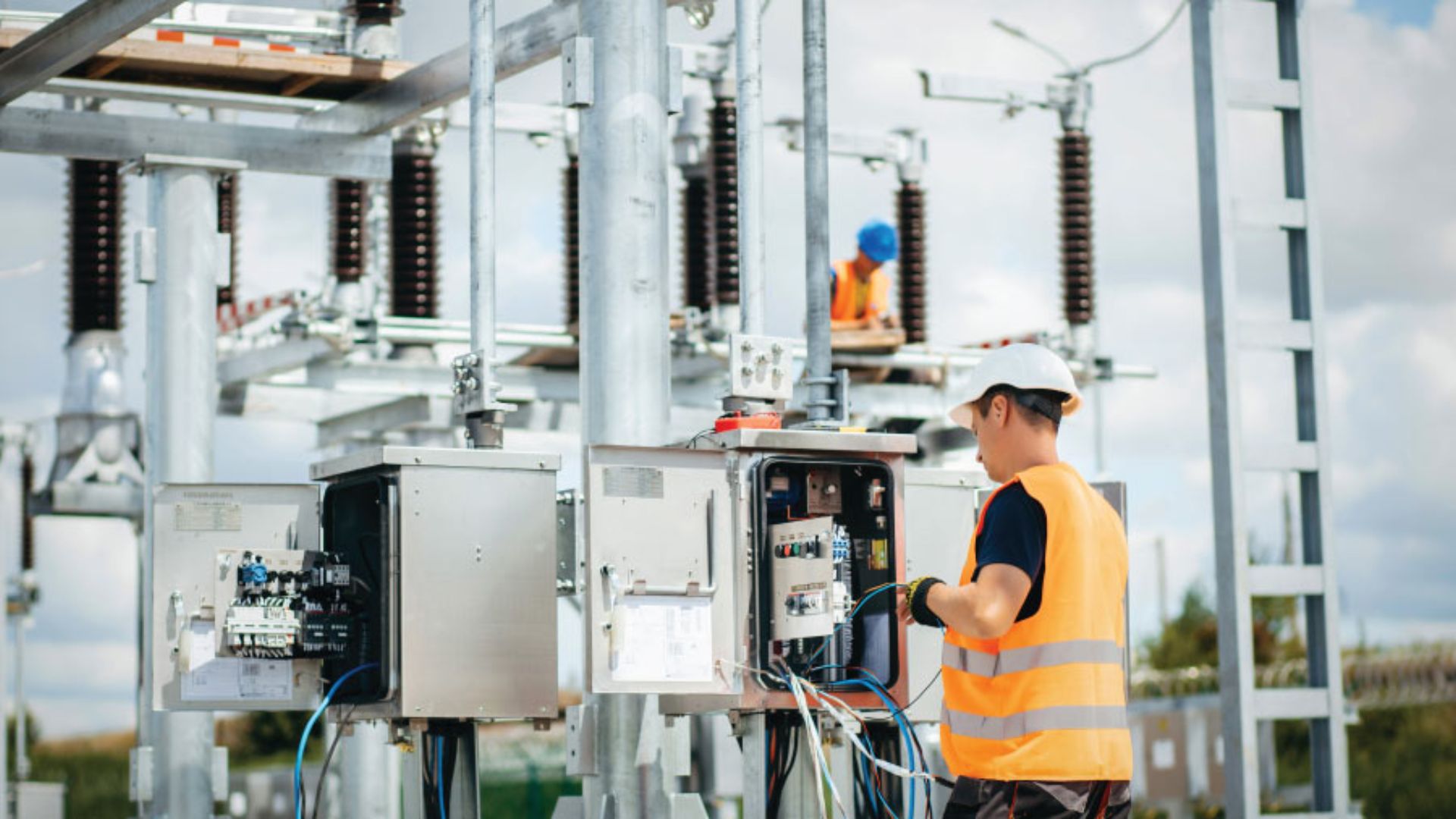
Explore ML’s Impact on Predictive Maintenance in Utilities
#ML #Utilities #Tech
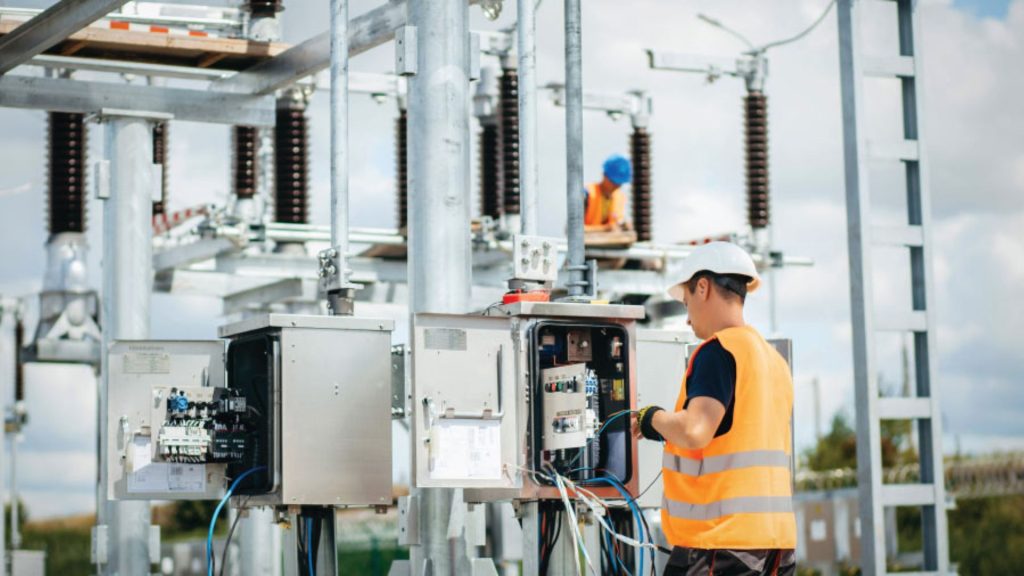
Introduction
The utility sector is essential for modern society, providing critical services such as electricity, water, and gas. Maintaining the vast infrastructure required to deliver these services poses significant challenges. Equipment failures can lead to service disruptions, safety hazards, and costly repairs. Traditional maintenance strategies, like reactive maintenance (fixing equipment after failure) or scheduled preventive maintenance (servicing equipment at regular intervals), are often inefficient and costly.
Machine Learning (ML) is transforming predictive maintenance in utilities by enabling data-driven insights that predict equipment failures before they happen. This proactive approach optimizes maintenance schedules, reduces downtime, extends asset lifespans, and enhances operational efficiency.
This article explores the impact of ML on predictive maintenance in the utilities sector, examining its benefits, applications, challenges, and future trends.
Role of Machine Learning in Predictive Maintenance
Machine Learning leverages data from sensors, historical maintenance records, and operational logs to identify patterns and anomalies indicative of potential equipment failures. Key ML techniques used in predictive maintenance include:
Anomaly Detection
ML models detect deviations from normal operating conditions by analyzing real-time data. This helps in identifying issues before they escalate into failures.
Regression Analysis
Regression models predict continuous outcomes, such as the remaining useful life (RUL) of equipment, allowing for timely maintenance interventions.
Classification Algorithms
These algorithms classify equipment status into categories (e.g., ‘normal’, ‘needs maintenance’, ‘failure imminent’) based on input data.
Time Series Analysis
ML models analyze time-dependent data to forecast future equipment behavior and identify trends that precede failures.
Benefits of ML in Predictive Maintenance for Utilities
Integrating Machine Learning into predictive maintenance offers numerous advantages for utility companies:
- Reduced Downtime: Predicting failures allows for maintenance to be scheduled proactively, minimizing unplanned outages.
- Cost Savings: Optimizing maintenance schedules reduces unnecessary maintenance and extends equipment life, leading to significant cost reductions.
- Improved Safety: Early detection of equipment issues prevents accidents and enhances safety for workers and the public.
- Enhanced Reliability: Consistent service delivery strengthens customer trust and satisfaction.
- Data-Driven Decision Making: ML provides actionable insights, supporting strategic planning and operational efficiency.
Key Applications in the Utilities Sector
Machine Learning-driven predictive maintenance is applied across various utility services:
Electric Power Generation and Distribution
ML models monitor equipment like turbines, transformers, and power lines to predict failures, optimize load distribution, and prevent blackouts.
Water Treatment and Distribution
Predictive maintenance ensures pumps, valves, and filtration systems operate efficiently, preventing leaks and contamination.
Gas Pipeline Monitoring
ML algorithms detect anomalies in pipeline pressure and flow rates, identifying leaks or weaknesses before they lead to hazardous situations.
Renewable Energy Systems
For wind turbines and solar panels, ML predicts component degradation, optimizing maintenance and maximizing energy production.
Case Studies
Utility Company A: Reducing Transformer Failures
Utility Company A implemented ML-based predictive maintenance for its transformers. By analyzing temperature, load, and vibration data, they reduced transformer failures by 30%, saving millions in repair costs and reducing service disruptions.
Water Authority B: Preventing Pipeline Leaks
Water Authority B used ML algorithms to monitor pressure and flow data in their distribution network. Early leak detection reduced water loss by 25% and minimized the environmental impact.
Energy Provider C: Optimizing Wind Turbine Maintenance
By applying ML models to predict component wear in wind turbines, Energy Provider C scheduled maintenance more effectively, increasing energy production by 15% and reducing maintenance costs.
Challenges and Considerations
While ML offers significant benefits, implementing predictive maintenance in utilities presents challenges:
Data Quality and Quantity
ML models require large amounts of high-quality data. Collecting and processing this data can be complex and resource-intensive.
Integration with Legacy Systems
Utilities often rely on legacy infrastructure. Integrating ML solutions requires compatibility with existing systems, which may necessitate upgrades.
Cybersecurity Risks
Increased connectivity and data sharing introduce cybersecurity vulnerabilities. Protecting sensitive operational data is crucial.
Cost of Implementation
Initial investment in ML technologies and skilled personnel can be substantial. Utilities must consider the long-term return on investment.
Regulatory Compliance
Compliance with industry regulations and standards must be maintained when implementing new technologies.
Future Trends in ML and Predictive Maintenance
The integration of ML in predictive maintenance is evolving, with emerging trends including:
Internet of Things (IoT) Integration
Increased use of IoT devices provides richer real-time data for ML models, enhancing predictive capabilities.
Edge Computing
Processing data at the edge (near the data source) reduces latency and bandwidth usage, enabling faster decision-making.
Advanced Analytics and AI
Combining ML with other AI technologies, such as deep learning, can improve the accuracy and scope of predictive maintenance models.
Digital Twins
Creating virtual replicas of physical assets allows for simulations and testing of maintenance strategies without impacting real-world operations.
Conclusion
Machine Learning is significantly impacting predictive maintenance in the utilities sector by providing intelligent, data-driven insights that optimize maintenance schedules, reduce costs, and enhance reliability. As the utility infrastructure continues to age and demands increase, ML-driven predictive maintenance becomes increasingly essential for ensuring uninterrupted service delivery and operational efficiency.
Embracing ML technologies enables utilities to proactively address maintenance challenges, improve safety, and position themselves at the forefront of technological innovation in the industry.