Explore ML’s Impact on Optimizing Retail Pricing Strategies
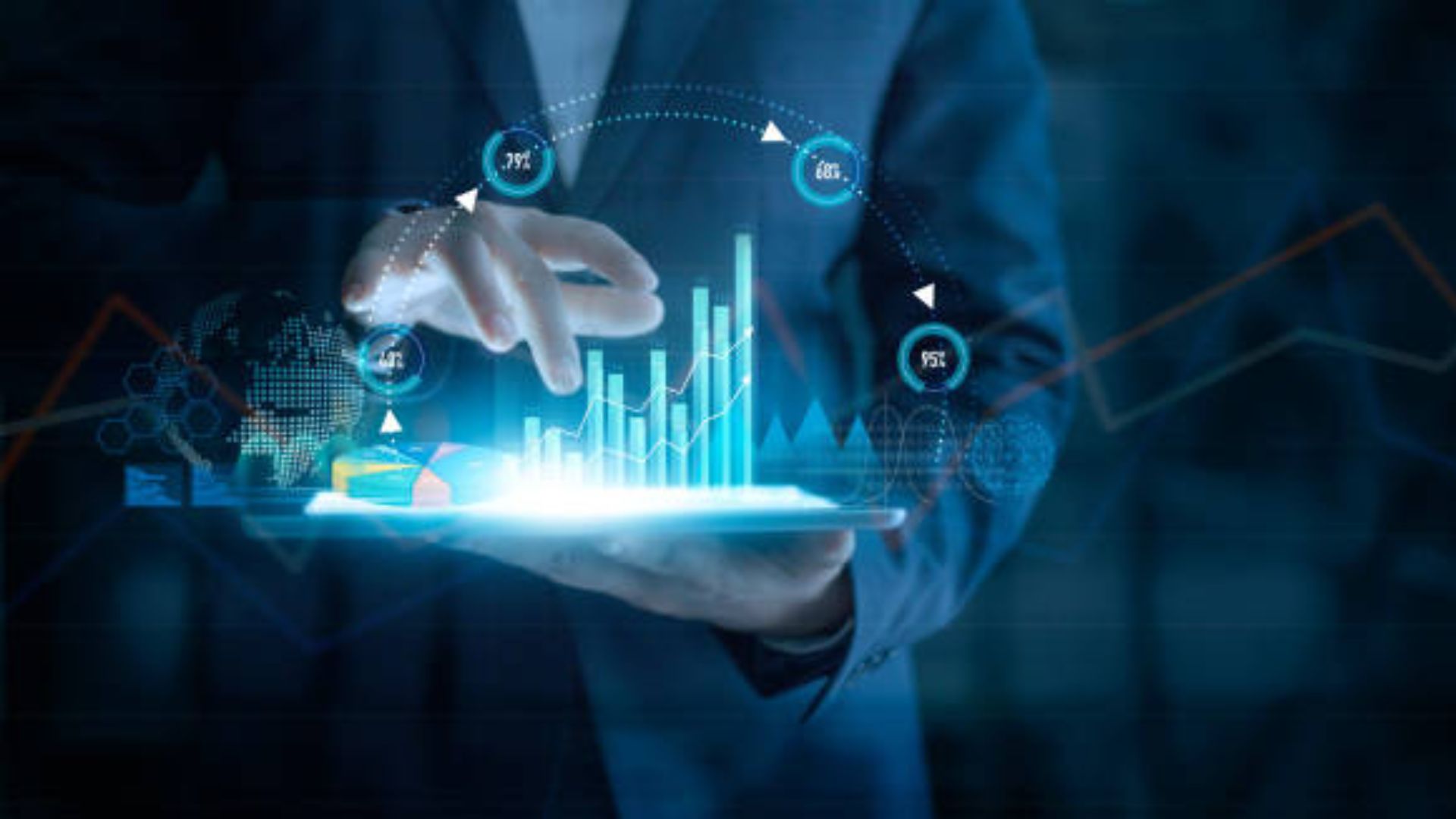
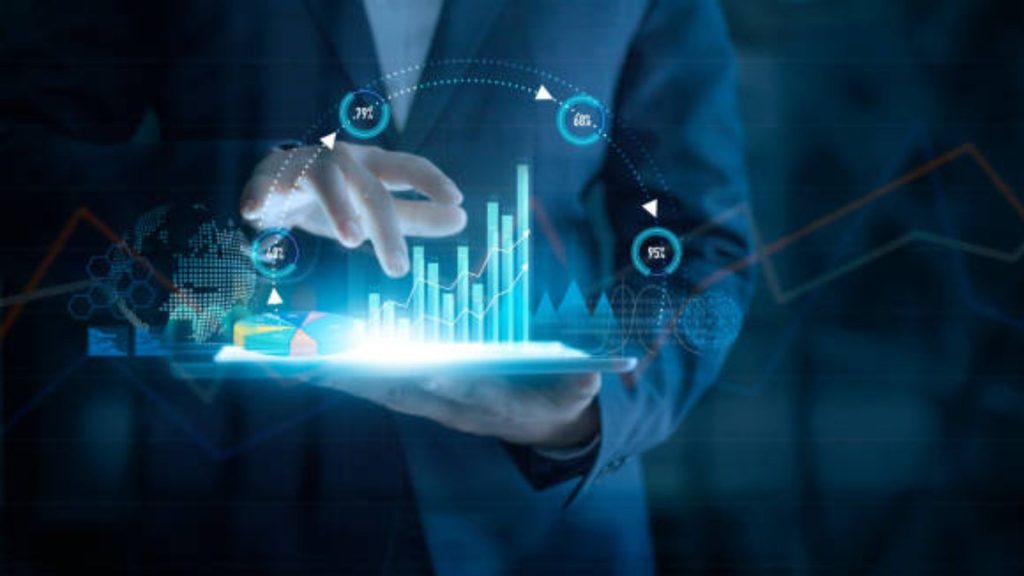
Explore ML’s Impact on Optimizing Retail Pricing Strategies
#ML #Retail #Business
Introduction
In a fast-paced retail landscape characterized by dynamic competition and ever-evolving consumer demands, pricing can be the key lever that determines profitability and market share. Traditional pricing strategies often rely on historical data, intuition, and manual adjustments. Today, Machine Learning (ML) is changing the game by enabling retailers to analyze vast datasets, understand consumer behaviors, and set prices that balance profitability with customer satisfaction.
This article examines how ML is revolutionizing retail pricing strategies, the benefits it brings, and the future trends that will shape the industry’s pricing playbook.
Key Applications of ML in Retail Pricing
ML-driven pricing solutions address numerous aspects of the retail value chain, from forecasting demand to adjusting prices in real-time:
Dynamic Pricing
ML algorithms analyze real-time data—such as current demand, competitor prices, and inventory levels—to automatically adjust prices. This ensures that retailers remain competitive while optimizing margins and capturing value from time-sensitive market conditions.
Demand Forecasting and Price Elasticity
By examining historical sales, seasonal patterns, and external factors, ML models predict how changes in price affect demand. Understanding price elasticity helps retailers find the ideal price points that maximize revenue without alienating customers.
Promotional Optimization
ML-driven insights guide promotional strategies, identifying which discounts, bundles, or loyalty offers will yield the greatest return. Retailers can test various scenarios and adopt promotions that effectively boost sales and customer engagement.
Competitor Benchmarking
Scraping competitor prices and comparing them against internal data helps retailers understand market positioning. ML algorithms process these findings to recommend price adjustments that maintain competitiveness while preserving margins.
Inventory and Markdown Management
ML models anticipate when to mark down slow-moving inventory, ensuring products sell before they become obsolete. This reduces waste, frees up storage space, and keeps assortments fresh and appealing.
Benefits of ML in Retail Pricing
Implementing ML-driven pricing strategies delivers tangible advantages for retailers:
- Increased Profitability: Optimal price points capture more value from each transaction without sacrificing sales volume.
- Enhanced Customer Satisfaction: Well-calibrated prices and promotions align with customer expectations, improving loyalty and brand perception.
- Faster Response to Market Changes: Automated, real-time pricing adjustments help retailers stay agile in volatile market conditions.
- Data-Driven Decision-Making: ML models turn raw data into actionable insights, guiding strategic pricing decisions and reducing guesswork.
- Scalability: ML solutions grow with the business, handling larger assortments, more locations, and increasingly diverse customer segments.
Key ML Technologies in Retail Pricing
Various ML techniques enable retailers to refine their pricing approaches:
Regression Models
Linear and nonlinear regression models help establish relationships between price changes and sales volumes, identifying price sensitivity and revenue-maximizing points.
Time-Series Forecasting
ML-driven time-series forecasting accounts for seasonality, holidays, and market cycles, improving demand predictions and guiding price adjustments accordingly.
Reinforcement Learning
Reinforcement learning algorithms adjust prices continuously based on real-time outcomes. The model “learns” which pricing actions yield the highest returns, refining its strategy over time.
Clustering and Segmentation
Unsupervised ML techniques group similar products or customer segments. Tailored pricing strategies for each cluster ensure that product categories and customer groups receive the most effective price points.
Case Studies
Retailer A: Dynamic Pricing Implementation
Retailer A adopted ML-driven dynamic pricing, adjusting prices based on real-time market conditions. They achieved a 15% increase in average margins while maintaining competitive positioning.
E-Commerce Platform B: Promotional Effectiveness
By leveraging ML to identify high-impact promotions, Platform B increased promotional ROI by 20%. Customers responded positively to targeted offers, raising satisfaction levels.
Brick-and-Mortar Chain C: Optimal Markdown Strategies
Chain C integrated ML models to predict when to apply discounts on surplus inventory. This approach reduced waste by 25% and improved store-level profitability.
Challenges and Considerations
Despite the benefits, deploying ML in retail pricing comes with hurdles:
Data Quality and Integration
Ensuring accurate, timely data from multiple sources is critical. Poor data quality or siloed systems can distort ML insights and undermine confidence in recommendations.
Ethical and Competitive Concerns
ML-driven pricing must comply with antitrust laws and avoid practices that harm consumers. Retailers should maintain transparency and fairness in their pricing strategies.
Model Interpretability
Understanding how ML models arrive at certain price recommendations is vital. Interpretability builds trust with stakeholders and ensures data-driven decisions are well-grounded.
Adoption and Change Management
Transitioning from manual pricing approaches to ML-driven methods requires training, communication, and a cultural shift to embrace data-driven decision-making.
Future Trends in ML-Driven Retail Pricing
The evolution of ML in retail pricing continues, with emerging trends promising further innovation:
Contextual Pricing
Future ML models will consider factors like location, weather, or consumer sentiment, adapting prices to dynamic contexts and delivering hyper-personalized offers.
Voice and Visual Commerce Integration
As voice and visual search gain traction, ML-driven pricing engines will respond to new input modes, ensuring seamless shopping experiences across platforms.
Real-Time Collaboration with Suppliers
Sharing ML-driven forecasts and recommendations with suppliers can align production and delivery schedules, minimizing stockouts and optimizing pricing opportunities.
Sustainability and Ethical Pricing
ML models will incorporate sustainability metrics, encouraging retailers to set prices that support ethical sourcing, responsible production, and transparent supply chains.
Conclusion
Machine Learning is reshaping how retailers set and manage prices, providing them with nuanced insights, dynamic adjustments, and predictive capabilities that drive profitability and customer satisfaction. By harnessing ML for pricing strategies, businesses can respond more effectively to market changes, tailor their offerings to consumer preferences, and stay competitive in an increasingly complex retail environment.
As ML technologies evolve, retailers that embrace these innovations will lead the way in delivering value, enhancing trust, and fostering lasting customer relationships through smarter pricing decisions.