The Future of AI in Customer Experience: What You Need to Know
The Future of AI in Customer Experience: What You Need to Know
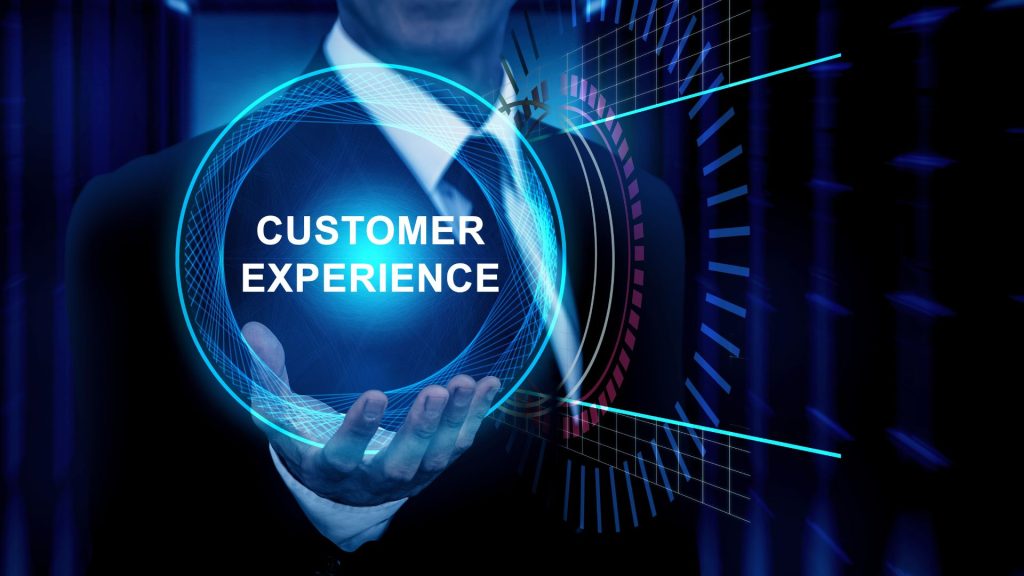
The integration of artificial intelligence (AI) into customer experience strategies is redefining the boundaries of customer interaction across various industries. As AI technology evolves, its influence in shaping and enhancing customer experiences is becoming increasingly significant. This article delves into the transformative power of AI in customer experience and what businesses need to know to stay ahead.
The Impact of AI on Customer Experience
Personalization at Scale
AI excels in analyzing large datasets to derive insights about individual customer preferences and behaviors. This capability enables businesses to deliver personalized experiences at scale, offering products, services, and interactions that are uniquely tailored to each customer. From personalized marketing messages to custom product recommendations, AI makes it possible to meet the unique needs of millions of customers simultaneously.
Automated Customer Support
AI-powered chatbots and virtual assistants are becoming the first point of contact in customer service interactions. These AI systems can handle a wide range of tasks from answering FAQs to solving complex issues, providing quick and efficient service. As these tools learn from each interaction, they become better at delivering accurate and helpful responses, improving customer satisfaction.
Seamless Omnichannel Experiences
AI helps in unifying customer experiences across multiple channels. By tracking and analyzing customer interactions across web, mobile, and in-store platforms, AI enables a seamless transition that feels consistent and integrated to the customer. This not only enhances the customer experience but also builds stronger brand loyalty.
Technological Innovations Driving AI in Customer Experience
Machine Learning and Data Analytics
At the core of AI’s impact are machine learning algorithms and advanced data analytics. These technologies enable the processing and interpretation of vast amounts of customer data, transforming it into actionable insights that can drive decision-making and strategic improvements in customer service.
Natural Language Processing (NLP)
NLP technologies are crucial in understanding and generating human language, allowing AI systems to communicate effectively with customers. This is evident in chatbots and AI customer service agents that interact with customers in a natural, conversational manner.
Predictive Analytics
AI’s ability to predict future customer behaviors and preferences by analyzing past data is revolutionary. This not only helps in anticipating customer needs before they arise but also in proactively addressing potential issues, thus enhancing the overall customer experience.
Challenges and Considerations
Privacy and Data Security
As AI systems rely heavily on customer data to function effectively, ensuring the privacy and security of this data is paramount. Businesses must adhere to stringent data protection regulations and ethical standards to maintain trust and protect their customers’ information.
Integration and Implementation Challenges
Integrating AI into existing customer experience strategies can be complex. It requires significant investment in technology and training, as well as a cultural shift towards data-driven decision making.
Maintaining Human Touch
Balancing AI automation with the irreplaceable human element of customer service is crucial. While AI can handle routine tasks effectively, human empathy and understanding are essential for dealing with complex customer needs and emotions.
The Future Outlook
As AI technology continues to advance, its role in customer experience will only grow deeper and more integral. Future trends may include more sophisticated AI personal assistants, greater use of AI in customer behavior prediction, and more immersive virtual reality experiences. These advancements will further redefine the standards of customer interaction and satisfaction.
Businesses that understand and leverage the capabilities of AI in enhancing customer experience will not only stay competitive but will also set new benchmarks in customer engagement and satisfaction.