How Machine Learning Enhances Supply Chain Transparency
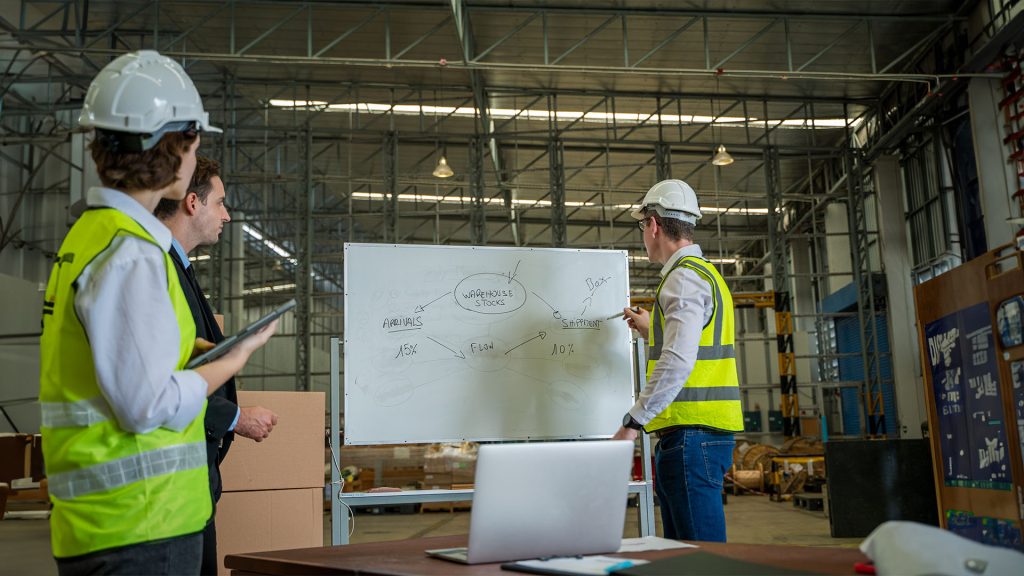
How Machine Learning Enhances Supply Chain Transparency
Supply chain transparency has become a critical focus for businesses worldwide. With increasing consumer demand for ethical sourcing, environmental sustainability, and efficient logistics, companies are turning to advanced technologies to improve visibility across their supply chains. Machine Learning (ML) is at the forefront of this transformation, offering powerful tools to enhance transparency, optimize operations, and foster trust among stakeholders. This article explores the various ways ML is revolutionizing supply chain transparency, supported by real-world examples and detailed insights.
Table of Contents
- Introduction to Supply Chain Transparency
- The Role of Machine Learning in Supply Chain Management
- Enhancing Data Visibility and Traceability
- Predictive Analytics for Demand Forecasting
- Optimizing Inventory Management
- Enhancing Supplier Risk Management
- Improving Logistics and Transportation
- Real-World Examples of ML in Supply Chain Transparency
- Ethical Considerations and Challenges
- Future Trends and Developments
- Conclusion
Introduction to Supply Chain Transparency
Supply chain transparency refers to the ability of a business to track and report on the origins, movements, and handling of products throughout the supply chain. Transparent supply chains allow companies to ensure ethical sourcing, maintain product quality, and comply with regulatory requirements. With increasing pressure from consumers and regulatory bodies, businesses are seeking innovative solutions to enhance transparency and build trust.
The Role of Machine Learning in Supply Chain Management
Machine Learning, a subset of artificial intelligence, involves the use of algorithms and statistical models to analyze and interpret complex data. In supply chain management, ML enables businesses to gain deeper insights, predict future trends, and optimize processes. By leveraging ML, companies can achieve greater transparency, improve decision-making, and enhance overall efficiency.
Enhancing Data Visibility and Traceability
Real-Time Tracking and Monitoring
ML-powered systems can provide real-time tracking and monitoring of products throughout the supply chain. By analyzing data from IoT devices, sensors, and RFID tags, ML algorithms can offer detailed insights into the location, condition, and status of goods. This real-time visibility helps businesses ensure product integrity, prevent losses, and respond quickly to any issues.
Blockchain Integration
Integrating ML with blockchain technology enhances supply chain transparency by providing a secure and immutable record of transactions. ML algorithms can analyze blockchain data to verify the authenticity of products, trace their origins, and ensure compliance with regulations. This combination of technologies offers a robust solution for maintaining transparency and trust.
IBM’s Food Trust platform uses ML and blockchain to track food products from farm to table, ensuring food safety and authenticity. Read more.
Predictive Analytics for Demand Forecasting
Accurate Demand Predictions
ML algorithms can analyze historical sales data, market trends, and external factors to generate accurate demand forecasts. These predictions help businesses optimize production schedules, reduce excess inventory, and meet customer demands more effectively.
Adaptive Forecasting Models
Adaptive forecasting models continuously learn from new data and adjust predictions in real-time. This dynamic approach ensures that businesses can respond to changing market conditions, seasonal trends, and unexpected disruptions, enhancing supply chain agility and transparency.
Amazon uses ML-driven predictive analytics to forecast demand for millions of products, optimizing inventory levels and ensuring timely delivery. Read more.
Optimizing Inventory Management
Inventory Optimization
ML algorithms can optimize inventory levels by analyzing sales patterns, lead times, and demand variability. This helps businesses maintain optimal stock levels, reduce holding costs, and minimize stockouts.
Automated Replenishment
Automated replenishment systems use ML to predict when inventory needs to be replenished, triggering orders automatically. This ensures that businesses always have the right amount of stock on hand, enhancing supply chain efficiency and transparency.
Walmart uses ML to manage its vast inventory, automating replenishment and optimizing stock levels across thousands of stores. Read more.
Enhancing Supplier Risk Management
Supplier Performance Analysis
ML algorithms can analyze supplier performance data, identifying patterns and trends that indicate potential risks. This helps businesses proactively manage supplier relationships, ensure compliance with standards, and mitigate risks.
Risk Prediction and Mitigation
By analyzing external data sources, such as news reports and social media, ML can predict potential disruptions in the supply chain, such as natural disasters or political instability. This enables businesses to develop contingency plans and maintain supply chain continuity.
Jaguar Land Rover uses ML to monitor supplier risks and ensure the reliability of its supply chain. Read more.
Improving Logistics and Transportation
Route Optimization
ML algorithms can optimize transportation routes by analyzing traffic patterns, weather conditions, and delivery schedules. This reduces transportation costs, improves delivery times, and minimizes the environmental impact of logistics operations.
Predictive Maintenance
Predictive maintenance systems use ML to analyze data from vehicles and equipment, predicting when maintenance is needed. This proactive approach prevents breakdowns, reduces downtime, and ensures the smooth operation of logistics networks.
DHL uses ML to optimize delivery routes and predict maintenance needs, improving efficiency and customer satisfaction. Read more.
Real-World Examples of ML in Supply Chain Transparency
1. UPS – Predictive Analytics for Delivery Efficiency
UPS uses ML to analyze delivery data and optimize routes, reducing fuel consumption and improving delivery times. The company’s ORION system leverages predictive analytics to make real-time routing decisions. Read more
2. Coca-Cola – Inventory Optimization
Coca-Cola uses ML to optimize its inventory levels, ensuring that products are available when and where they are needed. The company’s AI-driven systems analyze sales data and forecast demand, reducing waste and improving efficiency. Read more
3. Procter & Gamble – Supplier Risk Management
Procter & Gamble employs ML to monitor supplier performance and predict potential disruptions. The company’s risk management systems analyze a wide range of data sources to ensure a reliable supply chain. Read more
4. PepsiCo – Demand Forecasting
PepsiCo uses ML to forecast demand for its products, optimizing production schedules and reducing excess inventory. The company’s predictive analytics systems provide accurate and timely demand predictions. Read more
5. Unilever – Sustainable Sourcing
Unilever leverages ML to enhance transparency in its supply chain, ensuring sustainable sourcing of raw materials. The company’s AI-driven systems track the origins of products and verify compliance with sustainability standards. Read more
6. Shell – Predictive Maintenance
Shell uses ML for predictive maintenance of its equipment and facilities. The company’s AI systems analyze sensor data to predict maintenance needs, reducing downtime and ensuring operational efficiency. Read more
7. Maersk – Route Optimization
Maersk employs ML to optimize shipping routes, reducing fuel consumption and improving delivery times. The company’s AI-driven systems analyze a wide range of data to determine the most efficient routes for its fleet. Read more
8. Nestlé – Quality Control
Nestlé uses ML to enhance quality control in its supply chain. The company’s AI systems analyze data from production processes to detect anomalies and ensure product quality. Read more
9. Nike – Inventory Management
Nike leverages ML to optimize its inventory management, ensuring that products are available to meet customer demand. The company’s AI-driven systems analyze sales data and forecast demand, reducing stockouts and excess inventory. Read more
10. Starbucks – Supply Chain Optimization
Starbucks uses ML to optimize its supply chain, from sourcing raw materials to delivering finished products. The company’s AI systems analyze data from across the supply chain to improve efficiency and ensure product quality. Read more
11. Tesla – Manufacturing Efficiency
Tesla employs ML to enhance manufacturing efficiency and ensure supply chain transparency. The company’s AI-driven systems monitor production processes and predict maintenance needs, reducing downtime and improving quality. Read more
12. Airbus – Supplier Performance
Airbus uses ML to monitor supplier performance and ensure the reliability of its supply chain. The company’s AI systems analyze data from suppliers to detect potential issues and mitigate risks. Read more
13. Microsoft – AI in Logistics
Microsoft leverages ML to optimize logistics and transportation, improving delivery times and reducing costs. The company’s AI-driven systems analyze a wide range of data to optimize routes and ensure efficient operations. Read more
14. Johnson & Johnson – Supply Chain Resilience
Johnson & Johnson uses ML to enhance supply chain resilience, ensuring the availability of critical products. The company’s AI systems analyze data from across the supply chain to predict and mitigate potential disruptions. Read more
15. FedEx – Real-Time Tracking
FedEx employs ML to provide real-time tracking and visibility of shipments. The company’s AI-driven systems analyze data from sensors and IoT devices to ensure timely and accurate delivery. Read more
16. Apple – Supplier Transparency
Apple uses ML to enhance transparency in its supply chain, ensuring ethical sourcing and compliance with labor standards. The company’s AI systems track the origins of materials and verify supplier practices. Read more
17. Adidas – Demand Forecasting
Adidas leverages ML to forecast demand for its products, optimizing production schedules and reducing excess inventory. The company’s AI-driven systems provide accurate and timely demand predictions. Read more
18. GE – Predictive Analytics
General Electric uses ML for predictive analytics in its supply chain, optimizing inventory levels and reducing costs. The company’s AI systems analyze data from across the supply chain to make informed decisions. Read more
19. Intel – Supply Chain Visibility
Intel employs ML to enhance visibility in its supply chain, ensuring the availability of critical components. The company’s AI-driven systems monitor supplier performance and predict potential disruptions. Read more
20. Alibaba – Logistics Optimization
Alibaba uses ML to optimize its logistics network, improving delivery times and reducing costs. The company’s AI systems analyze data from warehouses, transportation, and customer orders to ensure efficient operations. Read more
Ethical Considerations and Challenges
Data Privacy and Security
The use of ML in supply chain transparency involves handling vast amounts of sensitive data, raising concerns about data privacy and security. Businesses must implement robust measures to protect this data and comply with relevant regulations.
Bias and Fairness in ML Algorithms
ML algorithms can inadvertently introduce biases, leading to unfair treatment of certain suppliers or regions. Ensuring fairness and transparency in ML systems is crucial for maintaining trust and equity in the supply chain.
Integration with Existing Systems
Integrating ML with existing supply chain management systems can be challenging. Businesses must ensure that their ML solutions are compatible with current technologies and processes to achieve seamless integration.
Future Trends and Developments
As ML technology continues to evolve, its impact on supply chain transparency will only grow. Future trends may include greater integration of IoT devices, advancements in predictive analytics, and the development of more sophisticated ML algorithms tailored to specific supply chain challenges.
Greater Integration of IoT Devices
The integration of IoT devices with ML will provide even greater visibility and control over the supply chain. These devices will generate vast amounts of data, which ML algorithms can analyze to provide real-time insights and predictive analytics.
Advancements in Predictive Analytics
Future advancements in predictive analytics will enable businesses to forecast demand, manage inventory, and optimize logistics with even greater accuracy. These improvements will enhance supply chain transparency and efficiency.
Development of Sophisticated ML Algorithms
The development of more sophisticated ML algorithms will allow businesses to address specific supply chain challenges, such as managing complex logistics networks or ensuring ethical sourcing. These advanced algorithms will provide deeper insights and more effective solutions.
Conclusion
Machine Learning is revolutionizing supply chain transparency, offering powerful tools to enhance visibility, optimize operations, and build trust among stakeholders. From real-time tracking and predictive analytics to supplier risk management and logistics optimization, ML is transforming the way businesses manage their supply chains. As the technology continues to evolve, its impact on supply chain transparency will only grow, paving the way for more innovative and efficient supply chain solutions.
For more insights and updates on the latest in ML and supply chain management, visit TGI.