Learn How AI is Revolutionizing User Interface Design
Learn How AI is Revolutionizing User Interface Design
#AI #UIDesign #Tech
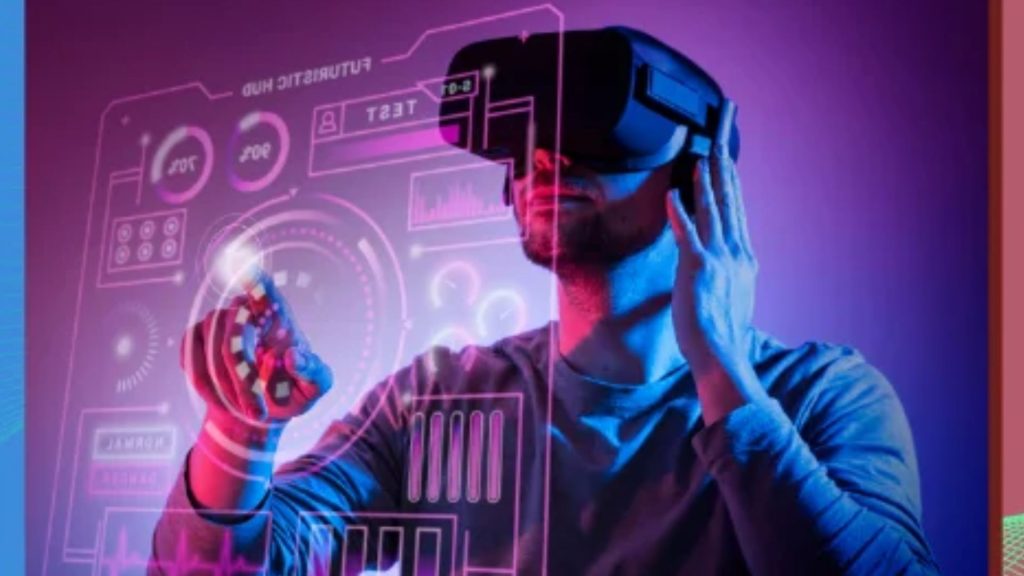
Introduction
User Interface (UI) design plays a crucial role in how people interact with technology. As digital experiences become more complex and personalized, the need for intuitive and adaptive interfaces has grown. Artificial Intelligence (AI) is stepping in to transform the UI landscape by streamlining design processes, enhancing user experiences, and making interfaces more responsive to individual needs.
This article explores how AI is revolutionizing UI design, the benefits it brings, and the future trends shaping this evolving field.
AI Integration in UI Design
AI technologies are being integrated into the design process and UI elements to improve efficiency, consistency, and personalization:
Automated Design Systems
AI-driven tools can generate layouts, color schemes, and typography suggestions based on brand guidelines, user preferences, or industry best practices. This automates routine tasks, allowing designers to focus on creativity and innovation.
Personalized Interfaces
By analyzing user behavior, AI tailors UI elements—such as navigation menus, content placement, and calls-to-action—to individual user preferences. This leads to more engaging and relevant experiences.
Predictive User Flows
AI models anticipate user actions and guide them through interfaces. Predictive UI elements suggest relevant content, shortcuts, or form fields, reducing friction and improving task completion rates.
Voice and Gesture Recognition
AI-driven interfaces support natural language processing and computer vision for voice commands and gesture controls. This expands the range of inputs users can rely on, making interfaces more accessible and intuitive.
Benefits of AI in UI Design
Integrating AI into UI design offers several advantages:
- Improved Efficiency: Automated design processes save time and effort, accelerating product development cycles.
- Enhanced User Satisfaction: Personalization and predictive elements create experiences that feel tailored and user-centric.
- Increased Accessibility: Voice and gesture controls break down barriers for users with disabilities, making interfaces more inclusive.
- Consistency in Branding: AI-driven style guides maintain brand coherence across multiple interfaces and platforms.
- Data-Driven Decisions: Continuous user feedback and analytics inform iterative improvements in design quality.
Key AI Technologies in UI Design
Several AI technologies are shaping the future of UI design:
Machine Learning
ML algorithms analyze user behavior patterns and feedback, identifying trends that inform design improvements and personalization strategies.
Natural Language Processing (NLP)
NLP powers voice-based interfaces and chatbots, enabling users to interact with systems through natural language commands or queries.
Computer Vision
Computer vision allows interfaces to understand visual inputs, such as gestures or facial expressions, expanding how users control digital experiences.
Reinforcement Learning
Reinforcement learning adapts UI elements in real-time, refining layouts and navigation based on user interactions and outcomes, optimizing for user satisfaction.
Case Studies
Company A: Automated Design Suggestions
Company A implemented an AI-driven tool that suggested layout variations and color palettes for their mobile app UI. This reduced design iteration time by 30% and improved user engagement rates.
Platform B: Personalized Dashboards
Platform B used ML models to learn user preferences and rearrange dashboard widgets. Users reported a 20% increase in productivity after the interface adapted to their usage patterns.
Startup C: Voice-Enabled Navigation
Startup C integrated NLP and speech recognition to create a voice-driven UI for their web application. This approach improved accessibility and attracted a broader user base.
Challenges and Considerations
While AI brings notable benefits, there are challenges to address:
Data Privacy and Security
Collecting user data to inform AI-driven interfaces must be done ethically and securely, complying with privacy regulations and user consent.
Bias and Fairness
AI models may inadvertently reflect societal biases present in their training data. Ensuring equitable and inclusive UI design requires careful model evaluation and refinement.
Complexity of Implementation
Integrating AI into UI design workflows can be complex. Designers and developers must collaborate closely and continuously update their skills.
User Acceptance
Some users may be wary of highly adaptive interfaces. Transparency about data usage and the option to customize AI-driven features can build trust.
Future Trends in AI and UI Design
The future of UI design with AI is filled with exciting possibilities:
Context-Aware Interfaces
UIs will adapt dynamically to a user’s context—such as location, device type, or time of day—providing seamless experiences regardless of environment.
Multimodal Interactions
Combining voice, gesture, and even haptic feedback will create richer interactions, accommodating diverse user preferences and abilities.
Generative Design
AI-powered generative design tools will create endless UI variations, testing and refining them at scale to find the most effective solutions.
Collaborative AI-Designer Workflows
Designers will work alongside AI, guiding the creative process while the AI handles repetitive tasks and data-driven refinements.
Conclusion
AI is revolutionizing UI design by automating routine tasks, personalizing experiences, and expanding the ways users interact with technology. As AI-driven interfaces become more context-aware and inclusive, designers and developers must navigate the challenges of privacy, bias, and complexity to ensure these solutions benefit all users.
Embracing AI in UI design opens the door to innovative, efficient, and highly adaptable digital experiences, setting a new standard for how we engage with the digital world.