Explore the Benefits of ML in Improving Data Security
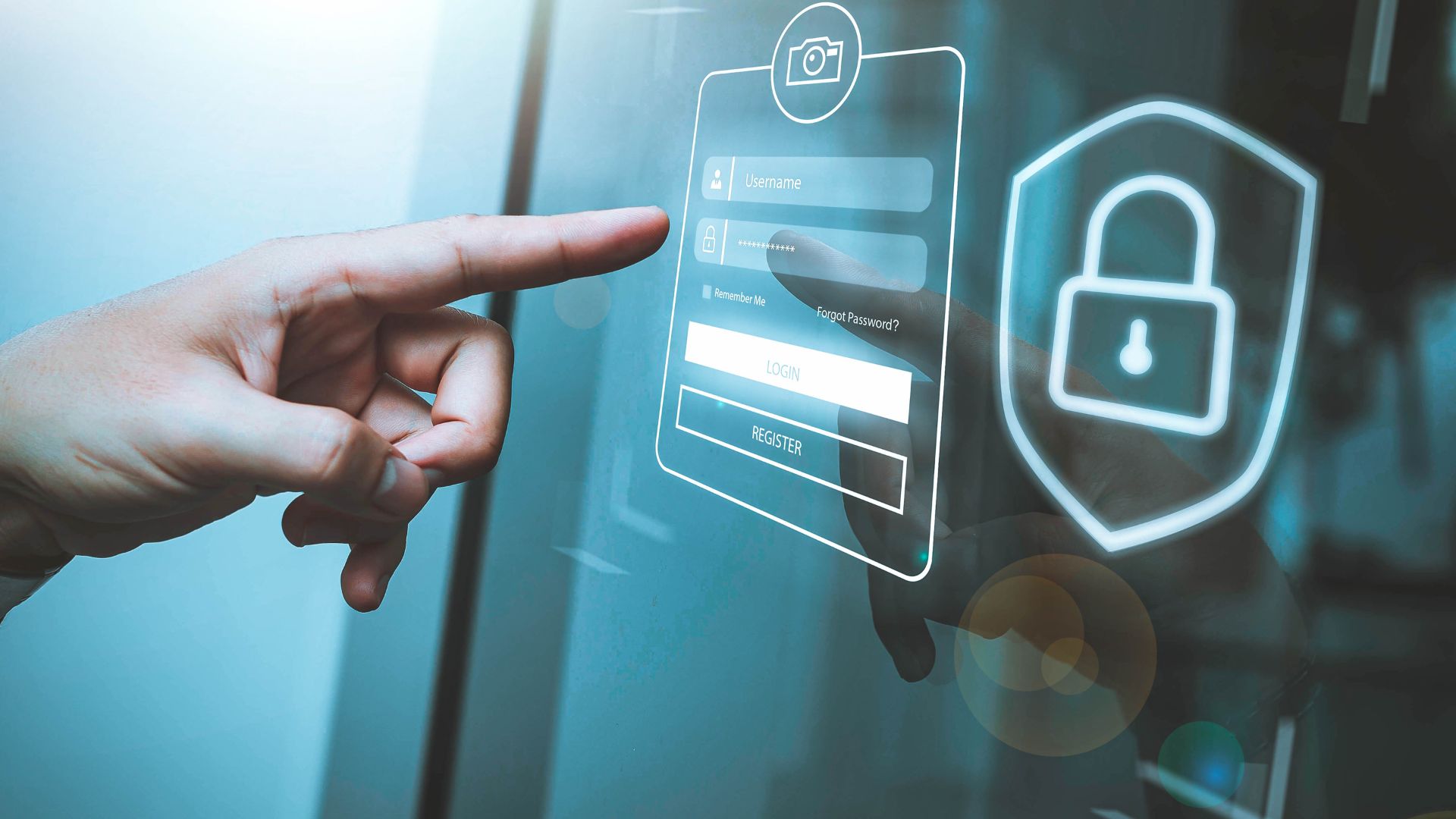
Explore the Benefits of ML in Improving Data Security
#ML #DataSecurity #Tech
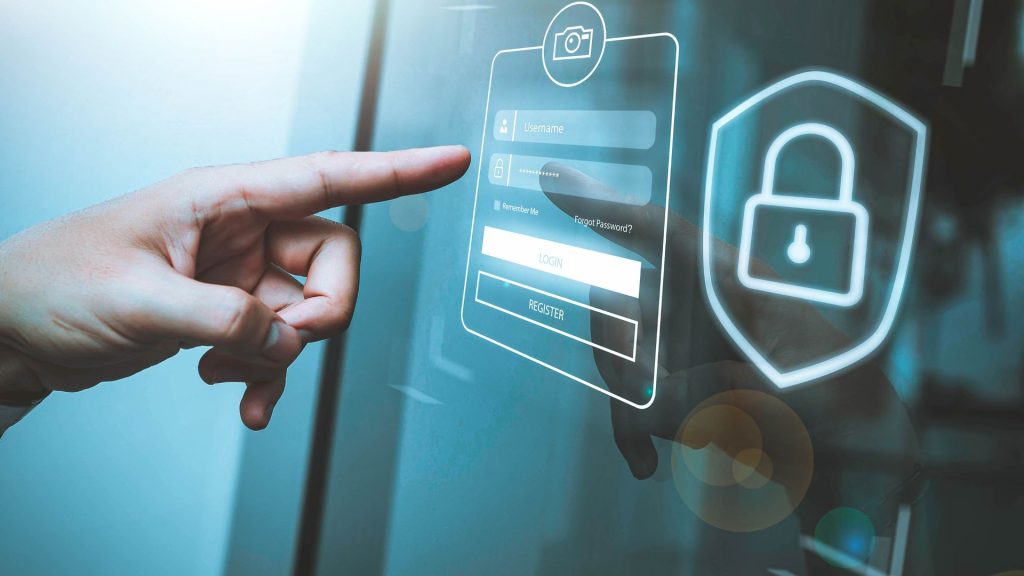
Introduction
In the digital age, data security has become a paramount concern for individuals and organizations alike. With the increasing frequency and sophistication of cyber attacks, traditional security measures are often insufficient to protect sensitive information. Machine Learning (ML), a subset of artificial intelligence, offers innovative solutions to bolster data security. By analyzing patterns and learning from vast amounts of data, ML algorithms can detect anomalies, predict threats, and automate responses to security incidents.
This article delves into how Machine Learning enhances data security, the benefits it brings, and the future of ML in the cybersecurity landscape.
The Evolving Threat Landscape
Cyber threats are constantly evolving, with attackers employing advanced techniques to bypass security defenses. Key challenges include:
- Advanced Persistent Threats (APTs): Long-term targeted attacks aimed at stealing data or surveilling systems.
- Zero-Day Exploits: Attacks that exploit previously unknown vulnerabilities before patches are available.
- Ransomware: Malware that encrypts data, demanding payment for decryption keys.
- Phishing Attacks: Deceptive emails or messages designed to trick users into revealing sensitive information.
Traditional security measures struggle to keep pace with these threats, necessitating more intelligent and adaptive solutions.
Role of Machine Learning in Data Security
Machine Learning enhances data security through various applications:
Anomaly Detection
ML algorithms can identify unusual patterns or behaviors that may indicate a security breach. By learning what constitutes ‘normal’ activity, they can detect deviations in real-time.
Predictive Analytics
ML models analyze historical data to predict future threats, allowing organizations to proactively address vulnerabilities before they are exploited.
Automated Threat Response
Upon detecting a threat, ML systems can automatically initiate responses, such as isolating affected systems or blocking malicious traffic, minimizing damage.
Fraud Detection
In financial services, ML is used to detect fraudulent transactions by recognizing patterns associated with fraudulent activity.
Spam and Malware Filtering
ML enhances email security by improving spam filters and detecting emails that contain malware or phishing attempts.
Benefits of ML in Data Security
Integrating ML into data security strategies offers several advantages:
- Real-Time Threat Detection: ML systems can identify and respond to threats as they occur, reducing response times.
- Adaptive Learning: ML models continuously learn from new data, improving over time and adapting to emerging threats.
- Reduced False Positives: By accurately distinguishing between legitimate and malicious activity, ML reduces the number of false alarms.
- Scalability: ML can handle large volumes of data, making it suitable for organizations of all sizes.
- Resource Optimization: Automation of security processes allows IT staff to focus on strategic initiatives rather than routine monitoring tasks.
ML Techniques in Data Security
Various ML techniques are employed to enhance data security:
Supervised Learning
Algorithms are trained on labeled datasets to recognize known threats. Useful in detecting specific types of malware or intrusion attempts.
Unsupervised Learning
Identifies patterns and anomalies in data without prior labeling. Effective in detecting novel threats and unusual activities.
Reinforcement Learning
Systems learn optimal responses through trial and error, improving automated threat response strategies.
Deep Learning
Utilizes neural networks with multiple layers to model complex data structures. Applied in image and speech recognition for advanced threat detection.
Case Studies
Company G: Enhancing Network Security
Company G implemented ML-based anomaly detection, resulting in a 50% reduction in undetected cyber attacks and a significant decrease in response times.
Company H: Fraud Prevention in Banking
By employing ML algorithms for transaction monitoring, Company H reduced fraudulent activities by 40%, saving millions in potential losses.
Company I: Email Security Enhancement
Company I used ML to improve spam filters, blocking 99% of phishing emails and reducing successful phishing attacks dramatically.
Challenges and Considerations
While ML offers significant benefits, there are challenges to consider:
Data Privacy Concerns
ML systems require access to large datasets, which may contain sensitive information. Ensuring data privacy and compliance with regulations is critical.
Quality of Data
The effectiveness of ML depends on the quality of the data. Incomplete or biased data can lead to inaccurate predictions.
Adversarial Attacks
Attackers may attempt to deceive ML models by feeding them malicious inputs designed to bypass detection.
Complexity and Cost
Implementing ML solutions can be complex and resource-intensive, requiring specialized expertise and infrastructure.
Future Trends in ML and Data Security
The intersection of ML and data security is evolving, with emerging trends including:
Federated Learning
Allows ML models to learn from data across multiple devices or servers without centralized data storage, enhancing privacy.
Explainable AI (XAI)
Develops ML models that are transparent and interpretable, aiding in understanding decision-making processes and ensuring compliance.
Integration with Blockchain
Combining ML with blockchain technology can enhance data integrity and security through decentralized and tamper-proof records.
AI-Driven Security Orchestration
Automating the coordination of multiple security tools and responses to create a unified defense strategy.
Conclusion
Machine Learning is playing an increasingly vital role in improving data security. By providing intelligent, adaptive, and proactive defenses against cyber threats, ML enhances an organization’s ability to protect sensitive information. While challenges exist, the benefits of integrating ML into data security strategies are substantial. As cyber threats continue to evolve, leveraging ML will be essential for organizations seeking to safeguard their data and maintain trust with their stakeholders.
Embracing ML technologies not only strengthens security posture but also positions organizations at the forefront of technological innovation in the cybersecurity field.