Harnessing Machine Learning to Revolutionize Inventory Management in Logistics
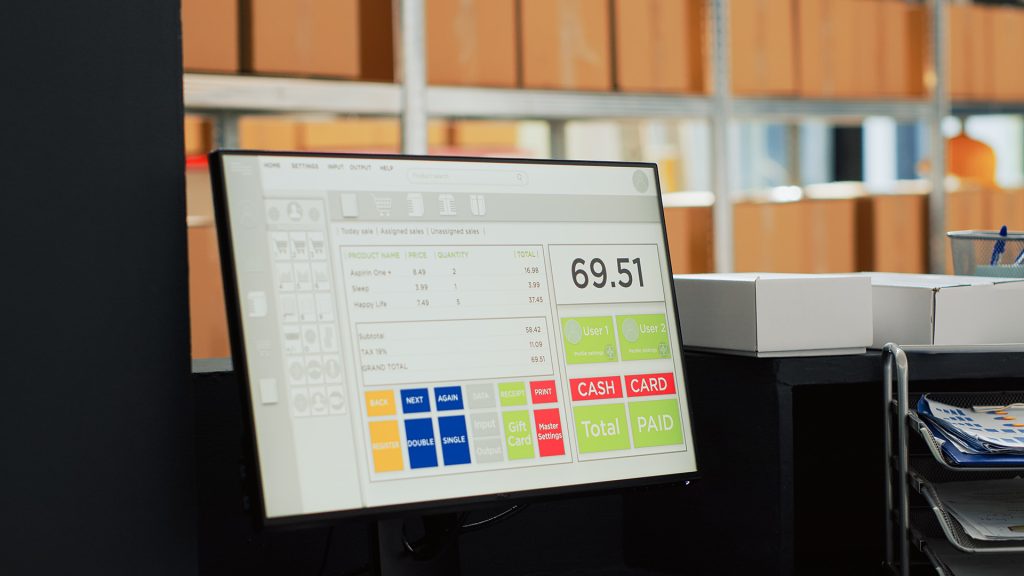
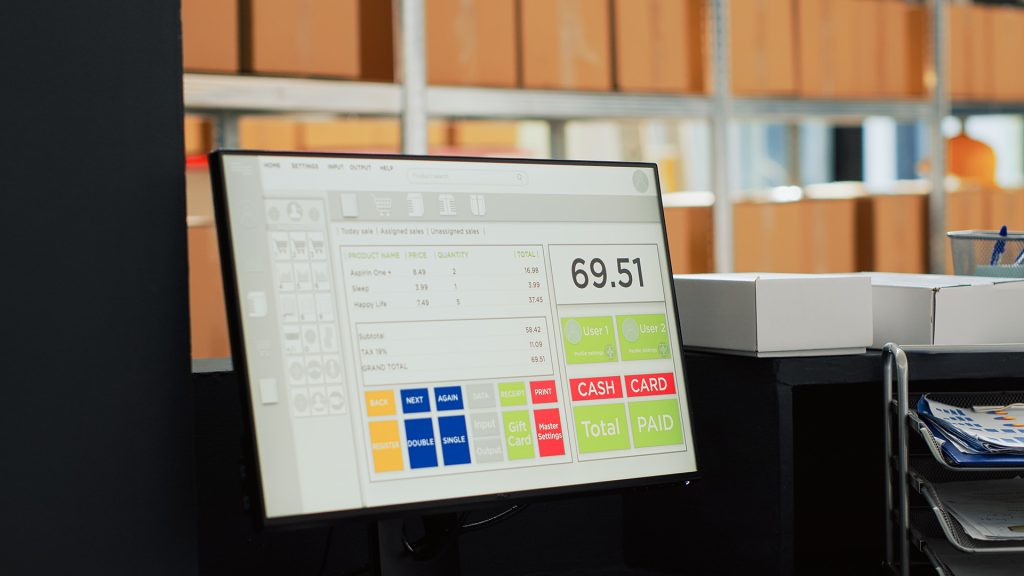
Machine Learning (ML) is revolutionizing the logistics industry by optimizing inventory management, enhancing supply chain efficiency, and reducing operational costs. From predicting demand and automating stock replenishment to identifying bottlenecks and minimizing waste, ML is transforming the way companies manage their inventory. This article explores twenty real-world examples showcasing how ML is optimizing inventory management in logistics, highlighting the significant impacts and future potential of this technology.
Real-World Examples of ML in Inventory Management
1. Walmart: Demand Forecasting
Walmart uses ML algorithms to forecast demand accurately, helping to optimize inventory levels across its vast network of stores. By analyzing historical sales data, customer behavior, and external factors like weather, Walmart ensures that products are always in stock, reducing both overstock and stockouts.
Read more2. Amazon: Automated Warehousing
Amazon leverages ML in its automated warehouses to manage inventory efficiently. Robots powered by ML algorithms move goods within the warehouse, optimizing storage space and picking routes, which increases operational efficiency and reduces fulfillment times.
Read more3. Zara: Real-Time Inventory Management
Zara employs ML to manage its inventory in real-time, allowing the company to respond quickly to changing fashion trends. By analyzing sales data and customer feedback, Zara ensures that the right products are available in the right stores at the right time.
Read more4. Alibaba: Smart Logistics
Alibaba’s Cainiao Network uses ML to optimize its logistics operations. The system analyzes data from various sources to forecast demand, optimize delivery routes, and manage inventory levels, ensuring efficient and timely deliveries.
Read more5. UPS: Predictive Analytics
UPS utilizes ML-driven predictive analytics to manage inventory and optimize delivery routes. By forecasting demand and identifying potential disruptions, UPS can make proactive decisions to ensure timely deliveries and efficient inventory management.
Read more6. Nestlé: Supply Chain Optimization
Nestlé uses ML to optimize its supply chain and inventory management. The system analyzes sales data, market trends, and external factors to predict demand and manage inventory levels, ensuring that products are always available when needed.
Read more7. Coca-Cola: Inventory Forecasting
Coca-Cola leverages ML for inventory forecasting, helping to manage its vast network of distributors and retailers. By analyzing sales data and market trends, Coca-Cola can optimize stock levels and reduce waste.
Read more8. Procter & Gamble: Supply Chain Resilience
Procter & Gamble uses ML to enhance the resilience of its supply chain. The company’s ML algorithms analyze data from various sources to predict demand, optimize inventory levels, and ensure a steady supply of products to retailers.
Read more9. Unilever: Dynamic Inventory Management
Unilever employs ML to manage its inventory dynamically. The system analyzes sales data, market conditions, and supply chain factors to optimize stock levels and ensure product availability.
Read more10. Home Depot: Inventory Optimization
Home Depot uses ML to optimize inventory across its network of stores and distribution centers. By forecasting demand and optimizing stock levels, Home Depot ensures that products are available when and where customers need them.
Read more11. PepsiCo: Demand Sensing
PepsiCo leverages ML for demand sensing, allowing the company to anticipate changes in consumer demand and adjust inventory levels accordingly. This helps PepsiCo to reduce waste and improve supply chain efficiency.
Read more12. Nike: Inventory Optimization
Nike uses ML to optimize inventory levels across its supply chain. By analyzing sales data and market trends, Nike can forecast demand and ensure that the right products are available in the right locations.
Read more13. Target: Automated Replenishment
Target employs ML to automate the replenishment of inventory in its stores. The system forecasts demand and automatically orders stock, ensuring that shelves are always stocked with the right products.
Read more14. H&M: Real-Time Inventory Tracking
H&M uses ML to track inventory in real-time, enabling the company to respond quickly to changes in consumer demand. This helps H&M to optimize stock levels and reduce overstock and stockouts.
Read more15. Adidas: Supply Chain Optimization
Adidas leverages ML to optimize its supply chain and inventory management. By analyzing sales data and market trends, Adidas can forecast demand and manage stock levels more efficiently.
Read more16. Carrefour: Inventory Forecasting
Carrefour uses ML to forecast inventory needs and manage stock levels across its stores. By predicting demand accurately, Carrefour ensures that products are available for customers while minimizing waste.
Read more17. Lidl: Automated Stock Management
Lidl employs ML to automate stock management in its stores. The system forecasts demand, optimizes inventory levels, and ensures that products are always available for customers.
Read more18. IKEA: Inventory Optimization
IKEA uses ML to optimize inventory levels across its global supply chain. By forecasting demand and managing stock levels, IKEA ensures that products are available when and where customers need them.
Read more19. Tesco: Real-Time Inventory Management
Tesco employs ML to manage inventory in real-time, ensuring that products are always available for customers. By analyzing sales data and predicting demand, Tesco optimizes stock levels and reduces waste.
Read more20. JD.com: Smart Supply Chain
JD.com leverages ML in its smart supply chain to optimize inventory management. The system forecasts demand, automates replenishment, and ensures efficient stock management, leading to improved customer satisfaction and reduced operational costs.
Read moreHow to Use ML and AI for Inventory Management in Logistics
Integrating ML and AI into inventory management involves several steps, from data collection and preprocessing to model development and implementation. Here’s a detailed guide on how companies can leverage ML and AI to optimize their inventory management processes:
1. Data Collection
The first step in utilizing ML for inventory management is gathering relevant data. This includes:
- Sales Data: Historical sales records provide insights into demand patterns.
- Customer Data: Information on customer preferences and behaviors helps predict future demand.
- Inventory Data: Current stock levels, lead times, and storage information are crucial for inventory management.
- External Data: Factors such as weather conditions, economic indicators, and market trends can impact demand and supply.
2. Data Preprocessing
Once the data is collected, it needs to be cleaned and prepared for analysis. This involves:
- Data Cleaning: Removing duplicates, handling missing values, and correcting errors in the data.
- Normalization: Standardizing data to ensure consistency and comparability.
- Feature Engineering: Creating new features that can help improve the performance of ML models.
3. Model Development
With the prepared data, companies can develop ML models tailored to their inventory management needs. Key models include:
- Demand Forecasting Models: Predict future demand based on historical sales data, customer behavior, and external factors. Techniques like time series analysis and regression models are commonly used.
- Inventory Optimization Models: Determine optimal stock levels, reorder points, and safety stock to minimize costs and avoid stockouts. Techniques include linear programming and simulation models.
- Anomaly Detection Models: Identify unusual patterns or anomalies in inventory data that could indicate issues like fraud, theft, or data entry errors. Clustering and outlier detection algorithms are used here.
4. Implementation
Implementing ML models in inventory management involves integrating them into existing systems and processes:
- Automated Replenishment Systems: Use ML models to automatically reorder stock based on predicted demand and current inventory levels.
- Real-Time Monitoring: Continuously monitor inventory levels and supply chain performance using ML algorithms to detect and respond to issues promptly.
- Decision Support Systems: Provide managers with data-driven insights and recommendations to make informed decisions about inventory management.
5. Evaluation and Improvement
After implementation, it’s essential to evaluate the performance of ML models and make continuous improvements:
- Performance Metrics: Track key metrics like forecast accuracy, stockout rates, and inventory turnover to assess the effectiveness of ML models.
- Feedback Loops: Use feedback from inventory managers and other stakeholders to refine models and improve accuracy.
- Continuous Learning: Regularly update models with new data to ensure they adapt to changing conditions and maintain their effectiveness.
Conclusion
Machine Learning is transforming inventory management in the logistics industry by enhancing efficiency, reducing costs, and improving overall performance. The examples provided illustrate the wide-ranging applications of ML in optimizing inventory across different sectors, showcasing its potential to drive significant advancements. As ML technology continues to evolve, its role in reshaping inventory management and logistics will only grow, paving the way for a more efficient and responsive supply chain.
Further Reading and Resources
For those interested in exploring this topic further, here are some additional resources and articles that delve deeper into the impact of ML on inventory management in logistics:
- “The Future of Supply Chain with AI and ML” by Deloitte
- “How AI and ML Are Revolutionizing Supply Chain Management” by McKinsey & Company
- “The Impact of Machine Learning on Inventory Management” by Harvard Business Review
- “AI in Logistics: Transforming the Supply Chain” by PwC
- “Leveraging AI for Smarter Inventory Management” by Forbes
By integrating ML into inventory management, logistics companies can achieve unprecedented levels of efficiency, accuracy, and responsiveness, leading to a more streamlined and effective supply chain. #ML #Logistics #SupplyChain