How AI is Improving Patient Data Analysis
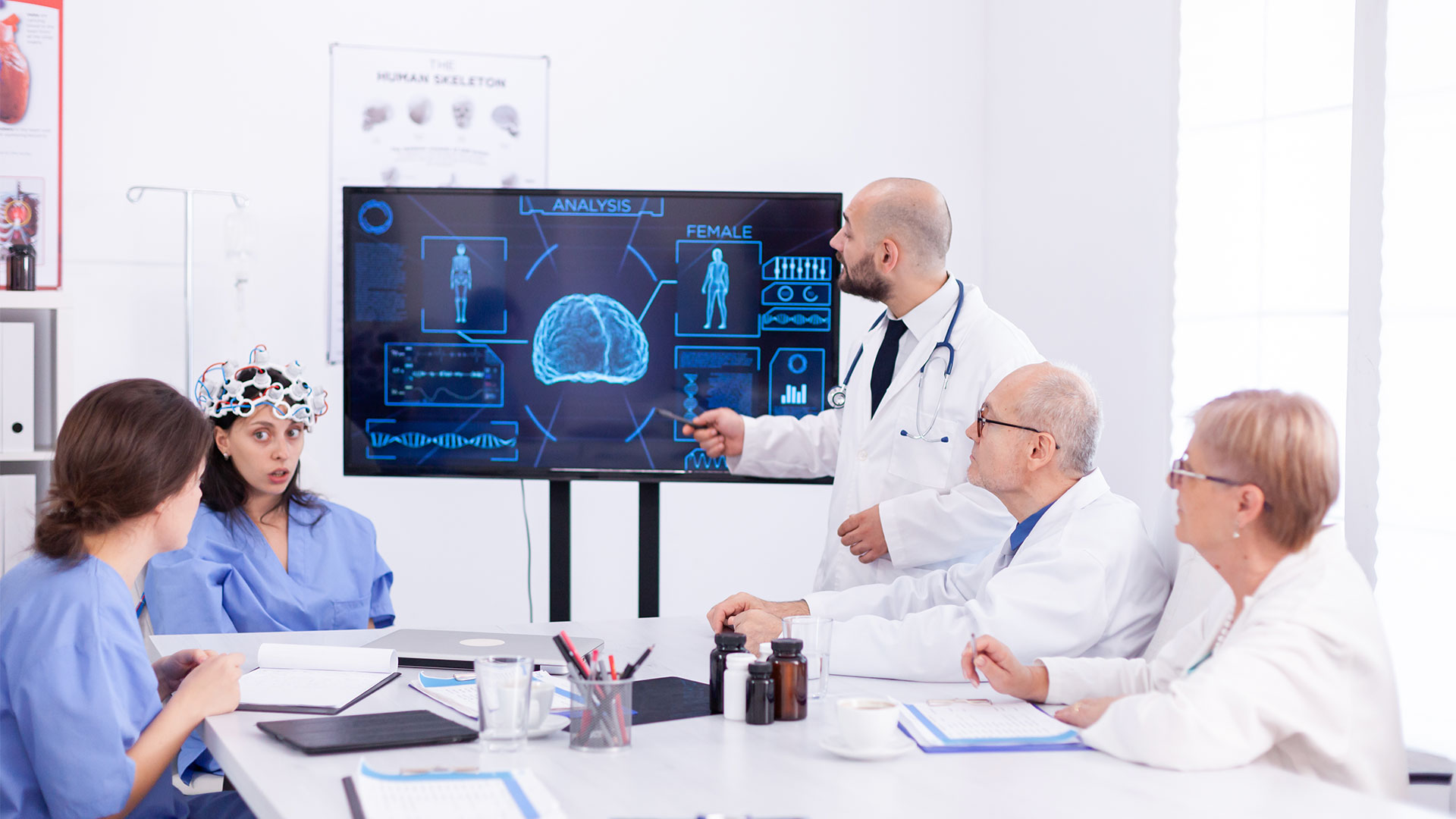
How AI is Improving Patient Data Analysis
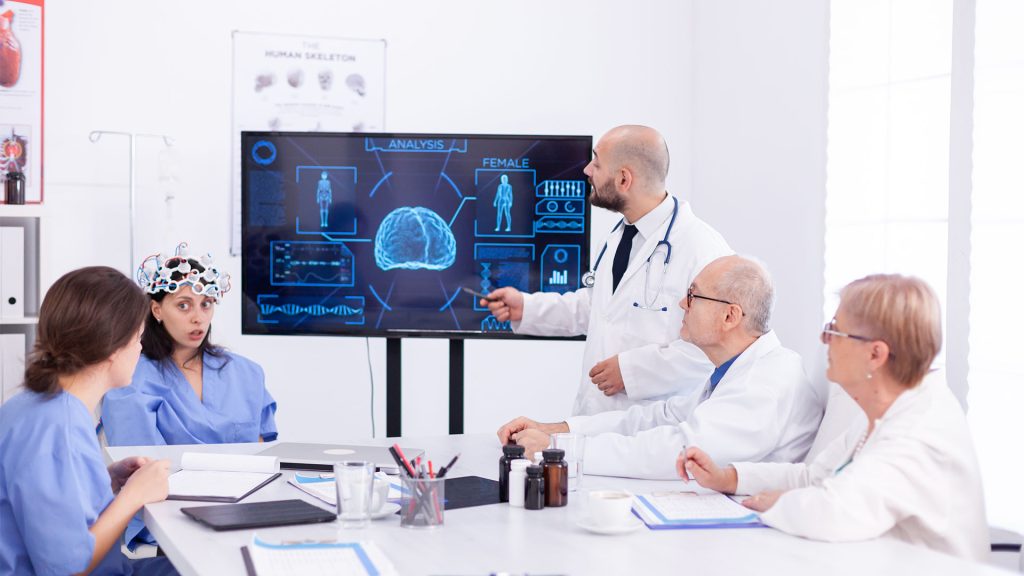
Artificial Intelligence (AI) is revolutionizing healthcare in numerous ways, and one of the most significant advancements is in patient data analysis. The ability to analyze vast amounts of data quickly and accurately is transforming how healthcare providers diagnose, treat, and manage patient care. This article explores how AI is improving patient data analysis, the benefits it brings, real-world applications, and the future of AI in healthcare.
Introduction
Patient data is one of the most valuable assets in healthcare. From electronic health records (EHRs) to imaging data and genomics, the sheer volume of information can be overwhelming for healthcare professionals. Traditional methods of data analysis often fall short in uncovering the complex patterns and insights needed for personalized medicine and effective treatment. AI is stepping in to bridge this gap, offering advanced analytics that can process and interpret vast datasets quickly and accurately.
Understanding AI in Patient Data Analysis
AI in healthcare involves using machine learning algorithms, natural language processing (NLP), and other AI techniques to analyze patient data. These technologies can identify patterns, predict outcomes, and provide recommendations that support clinical decision-making. AI-driven data analysis can be applied to various types of patient data, including structured data (e.g., lab results, vital signs) and unstructured data (e.g., physician notes, medical images).
Key Benefits of AI in Healthcare Data Analysis
AI offers several significant benefits in patient data analysis:
Improved Diagnostics
AI enhances diagnostic accuracy by analyzing patient data more comprehensively than traditional methods. For instance, AI algorithms can analyze medical images to detect early signs of diseases such as cancer, heart disease, or neurological disorders, often with greater accuracy than human experts. This leads to earlier interventions and better patient outcomes.
Personalized Treatment Plans
AI can analyze patient data to create personalized treatment plans based on individual characteristics, such as genetics, lifestyle, and medical history. This tailored approach to medicine improves treatment efficacy and reduces the risk of adverse effects.
Predictive Analytics
AI-powered predictive analytics can forecast patient outcomes, such as the likelihood of disease progression or hospital readmission. By identifying high-risk patients, healthcare providers can intervene early, potentially preventing complications and reducing healthcare costs.
Streamlined Operations
AI helps automate administrative tasks, such as data entry, billing, and scheduling, allowing healthcare professionals to focus more on patient care. Additionally, AI can optimize resource allocation, ensuring that medical staff and equipment are used efficiently.
Enhanced Research and Drug Development
AI can analyze vast datasets from clinical trials, research studies, and patient records to identify potential drug candidates, predict their effectiveness, and reduce the time it takes to bring new treatments to market. This accelerates innovation in drug development and personalized medicine.
Real-World Applications of AI in Patient Data Analysis
Here are some real-world examples of how AI is being used in patient data analysis:
Medical Imaging
AI is being used to analyze medical images, such as X-rays, MRIs, and CT scans, to detect diseases and conditions. For example, Google’s DeepMind has developed AI algorithms that can detect eye diseases from retinal scans with a high degree of accuracy, enabling early treatment and preventing vision loss. Read more
Electronic Health Records (EHRs)
AI is transforming how EHRs are managed and utilized. By using NLP, AI can extract relevant information from unstructured text, such as physician notes, and integrate it with structured data. This provides a more comprehensive view of a patient’s health and improves decision-making. IBM’s Watson Health is one such platform that leverages AI to analyze EHRs and assist clinicians in providing personalized care. Read more
Genomic Data Analysis
AI is playing a critical role in analyzing genomic data, enabling precision medicine. By identifying genetic mutations and predicting their impact on health, AI helps tailor treatments to individual patients. Companies like Tempus are using AI to analyze genomic data and develop personalized cancer treatment plans. Read more
Predictive Healthcare Models
AI-driven predictive models are being used to forecast patient outcomes, such as the likelihood of hospital readmissions or disease progression. For example, the Mayo Clinic uses AI to predict which patients are at risk of developing complications after surgery, allowing for timely interventions. Read more
Remote Patient Monitoring
AI-powered devices and wearables collect real-time data from patients, such as heart rate, blood pressure, and glucose levels. This data is analyzed to detect anomalies and provide early warnings to healthcare providers, enabling proactive care management. Fitbit and Apple Watch are examples of consumer devices that incorporate AI for health monitoring. Read more Read more
Challenges and Considerations
Data Privacy and Security
Handling sensitive patient data requires stringent data privacy and security measures. AI systems must comply with regulations such as HIPAA to protect patient information from breaches and unauthorized access.
Data Quality and Integration
AI’s effectiveness depends on the quality of the data it analyzes. Inconsistent, incomplete, or biased data can lead to inaccurate predictions and recommendations. Integrating data from multiple sources, such as EHRs, wearables, and lab systems, can also be challenging.
Ethical Concerns
The use of AI in healthcare raises ethical questions, such as bias in algorithms, transparency in decision-making, and the potential for AI to replace human judgment. Ensuring that AI is used responsibly and ethically is crucial for maintaining trust in the technology.
Adoption and Implementation
Integrating AI into existing healthcare systems requires significant investment in technology and training. Healthcare providers must be willing to adopt new workflows and processes to fully benefit from AI-driven data analysis.
Future Trends in AI-Driven Healthcare
Explainable AI
As AI becomes more integrated into healthcare, there is a growing demand for explainable AI—systems that provide clear, understandable reasons for their decisions. This transparency will be essential for gaining the trust of healthcare professionals and patients.
AI-Powered Virtual Assistants
AI-driven virtual assistants, such as chatbots, are increasingly being used to interact with patients, answer questions, and provide medical advice. These assistants can analyze patient data and provide recommendations based on the latest medical guidelines.
Advanced Predictive Analytics
AI’s predictive capabilities will continue to improve, enabling even more accurate forecasts of patient outcomes. This will lead to more proactive care management and better health outcomes.
Integration with Telemedicine
AI will play a crucial role in enhancing telemedicine by analyzing patient data remotely and providing real-time insights to healthcare providers. This will improve access to care, especially in remote or underserved areas.
Conclusion
AI is revolutionizing patient data analysis by providing healthcare providers with powerful tools to interpret complex datasets, predict outcomes, and personalize treatments. From improving diagnostics to enhancing patient care and streamlining operations, AI is transforming healthcare in profound ways. As technology continues to advance, the potential for AI to improve patient data analysis and overall healthcare delivery is limitless.
For more insights and resources on how AI is improving patient data analysis, visit TGI.