How ML Enhances Predictive Analytics in Real Estate
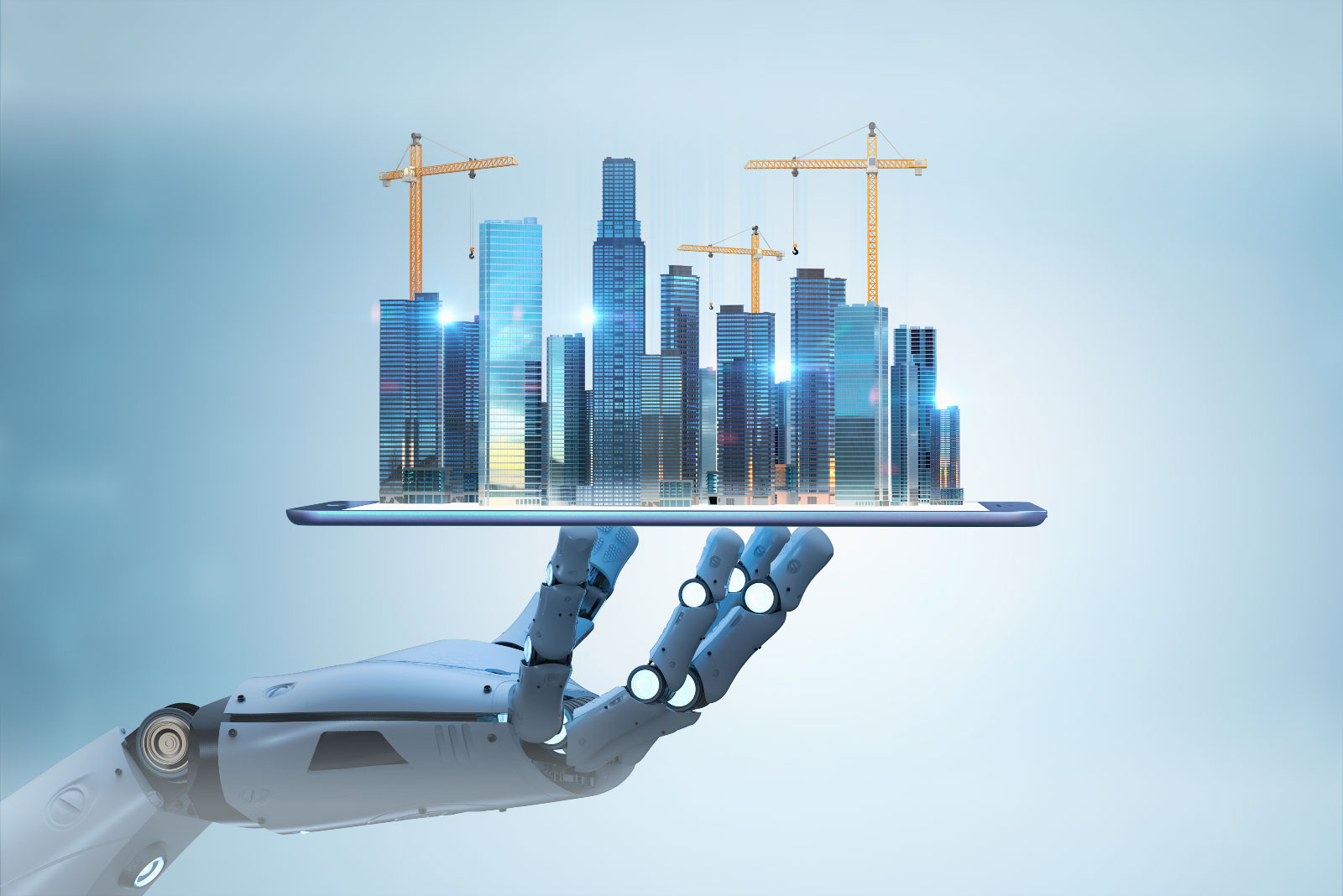
How Machine Learning Enhances Predictive Analytics in Real Estate: Transforming Property Markets with Data-Driven Insights
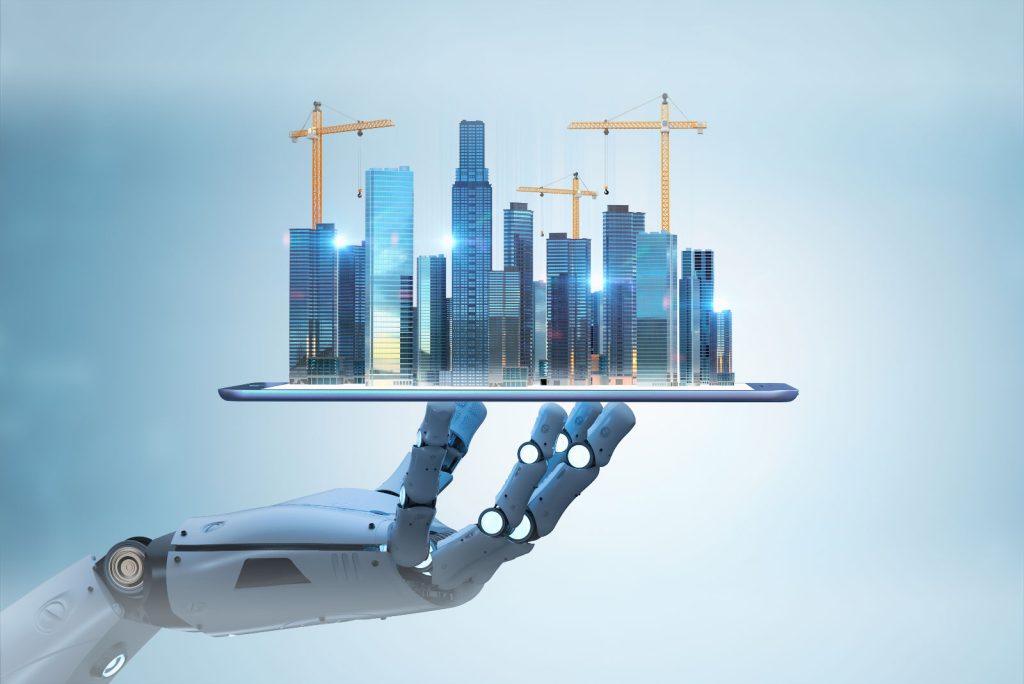
The real estate industry has long relied on intuition, experience, and traditional data analysis to make decisions. However, the emergence of Machine Learning (ML) is revolutionizing predictive analytics in real estate, enabling more accurate forecasts, better decision-making, and a deeper understanding of market trends. From predicting property values to optimizing investment strategies, ML is transforming the way real estate professionals operate. In this article, we’ll explore how ML enhances predictive analytics in real estate, the benefits it offers, and real-world examples that demonstrate its impact.
Introduction
Real estate markets are complex, with numerous factors influencing property values, demand, and investment potential. Traditionally, real estate professionals relied on historical data, market trends, and personal experience to make predictions. However, the rise of Machine Learning has introduced a new era of predictive analytics in real estate. By analyzing vast datasets, identifying patterns, and making data-driven predictions, ML is empowering real estate professionals to make more informed decisions and stay ahead of the competition.
Understanding Predictive Analytics in Real Estate
Predictive analytics in real estate involves using historical data, market trends, and statistical models to forecast future outcomes. This can include predicting property values, rental income, market demand, and investment returns. The goal of predictive analytics is to provide actionable insights that help real estate professionals make better decisions, mitigate risks, and optimize their strategies.
The Role of Machine Learning in Predictive Analytics
Machine Learning enhances predictive analytics by automating data analysis, uncovering hidden patterns, and making more accurate predictions. Here’s how ML is transforming predictive analytics in real estate:
Data Collection and Analysis
ML algorithms can process vast amounts of data from various sources, including property listings, transaction records, social media, and economic indicators. By analyzing this data, ML models can identify patterns and correlations that may not be apparent through traditional methods.
Property Valuation
ML models can predict property values with greater accuracy by analyzing factors such as location, property size, historical price trends, and nearby amenities. These models continuously learn from new data, improving their predictions over time. Companies like Zillow use ML to estimate property values with their “Zestimate” tool.
Market Trend Prediction
ML can analyze historical market data to predict future trends, such as changes in property prices, rental demand, and housing supply. This helps real estate professionals make informed decisions about when to buy, sell, or invest in properties.
Investment Risk Assessment
ML algorithms can assess the risk of real estate investments by analyzing factors such as market volatility, economic conditions, and property-specific data. This enables investors to make more informed decisions and minimize potential losses.
Customer Behavior Analysis
ML can analyze customer behavior patterns, such as search queries, preferences, and transaction history, to predict future actions. This allows real estate agents to tailor their marketing strategies and offer personalized recommendations to clients.
Key Benefits of ML in Real Estate
ML offers numerous benefits in predictive analytics for real estate:
Increased Accuracy
ML-driven predictive analytics provide more accurate forecasts compared to traditional methods. By analyzing vast amounts of data and learning from historical trends, ML models can make predictions that are more reliable and precise.
Time Efficiency
ML automates the data analysis process, allowing real estate professionals to quickly generate insights and make decisions without spending hours manually analyzing data. This saves time and resources, enabling professionals to focus on higher-level tasks.
Better Decision-Making
With more accurate predictions, real estate professionals can make better decisions regarding property investments, pricing strategies, and market positioning. This leads to increased profitability and reduced risk.
Enhanced Customer Experience
By leveraging ML to analyze customer behavior, real estate agents can offer personalized recommendations and services. This improves the customer experience, leading to higher satisfaction and increased client retention.
Competitive Advantage
Real estate professionals who adopt ML-driven predictive analytics gain a competitive edge by staying ahead of market trends, identifying opportunities early, and making data-driven decisions that others may overlook.
Real-World Applications of ML in Real Estate
Here are some real-world examples of how ML is enhancing predictive analytics in real estate:
Zillow’s Zestimate
Zillow’s Zestimate tool is a well-known example of ML in real estate. The platform uses ML algorithms to predict property values by analyzing data on property characteristics, location, and market trends. The Zestimate model continuously learns from new data, improving its accuracy over time. Zillow’s use of ML has made property valuations more accessible and reliable for buyers, sellers, and real estate agents alike. Read more
Redfin’s Predictive Analytics
Redfin uses ML to analyze market data and predict future property values, trends, and demand. The platform provides real-time insights to help buyers and sellers make informed decisions. Redfin’s ML-driven approach enhances the accuracy of its recommendations, giving users a clearer understanding of market conditions. Read more
Reonomy’s Commercial Real Estate Insights
Reonomy leverages ML to provide predictive analytics for commercial real estate. By analyzing data on property transactions, ownership, and market trends, Reonomy’s platform predicts property values, investment potential, and market opportunities. This helps investors and developers make data-driven decisions in the commercial real estate sector. Read more
HouseCanary’s Valuation Model
HouseCanary uses ML to create highly accurate property valuation models. The platform analyzes data on property characteristics, location, and market trends to predict property values and investment potential. HouseCanary’s ML-driven approach provides real estate professionals with valuable insights for making informed decisions. Read more
Compass’s AI-Powered Real Estate Platform
Compass uses ML to power its real estate platform, providing agents with predictive analytics on property values, market trends, and customer behavior. Compass’s AI-driven tools help agents stay ahead of the competition by offering personalized recommendations and data-driven insights. Read more
Challenges and Considerations
Data Quality and Availability
ML models rely on high-quality data to make accurate predictions. Incomplete or inaccurate data can lead to flawed predictions, so ensuring data quality and availability is crucial for successful ML implementation in real estate.
Algorithm Bias
ML algorithms can be biased if trained on biased data. This can result in inaccurate predictions and unfair outcomes. Addressing bias in ML models is essential for ensuring fairness and accuracy in predictive analytics.
Integration with Existing Systems
Implementing ML-driven predictive analytics requires integrating new technologies with existing real estate systems and processes. This can be challenging for companies with legacy systems that may not be compatible with modern ML tools.
Cost of Implementation
While ML offers significant benefits, implementing ML-driven predictive analytics can be costly. Real estate companies need to consider the upfront investment and ongoing maintenance costs when adopting these technologies.
Future Trends in ML-Driven Real Estate
AI-Powered Real Estate Agents
The future of real estate may see the rise of AI-powered agents who use ML to analyze data, predict market trends, and provide personalized recommendations to clients. These agents will be able to offer a more efficient and data-driven service, enhancing the customer experience.
Predictive Maintenance
ML will play a significant role in predictive maintenance, where algorithms analyze data from smart home devices and property sensors to predict maintenance needs. This will help property owners and managers address issues before they become costly problems.
Hyper-Personalized Marketing
As ML continues to advance, real estate professionals will be able to deliver hyper-personalized marketing campaigns that target specific buyer personas with tailored messages and offers. This will improve lead conversion rates and increase customer satisfaction.
Real-Time Market Insights
ML-driven predictive analytics will provide real-time market insights, allowing real estate professionals to make data-driven decisions instantly. This will reduce the time it takes to respond to market changes and capitalize on opportunities.
Smart Cities and Real Estate
ML will play a crucial role in the development of smart cities, where data-driven technologies optimize urban planning, infrastructure, and real estate development. Predictive analytics will help cities manage growth, enhance sustainability, and improve the quality of life for residents.
Conclusion
Machine Learning is transforming predictive analytics in real estate, providing professionals with more accurate forecasts, better decision-making tools, and a deeper understanding of market trends. From property valuation to investment strategies, ML is revolutionizing the way real estate operates. As the technology continues to advance, the potential for ML to enhance predictive analytics in real estate is limitless. By embracing ML-driven predictive analytics, real estate professionals can stay ahead of the competition and unlock new opportunities in the ever-evolving property market.
For more insights and resources on how ML is enhancing predictive analytics in real estate, visit TGI.