How ML Enhances Real-Time Data Analytics
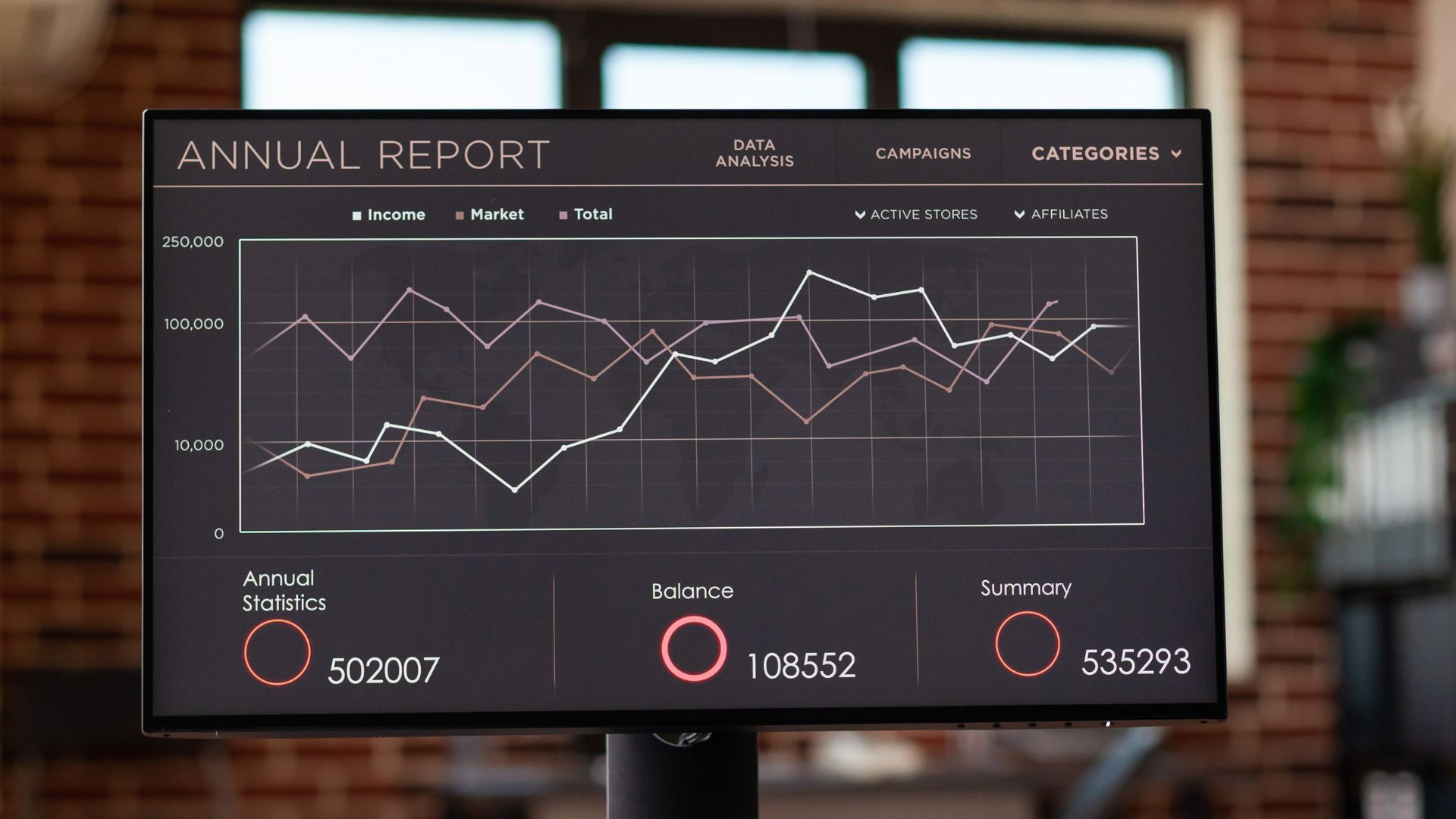
How ML Enhances Real-Time Data Analytics
#ML #DataAnalytics #Business
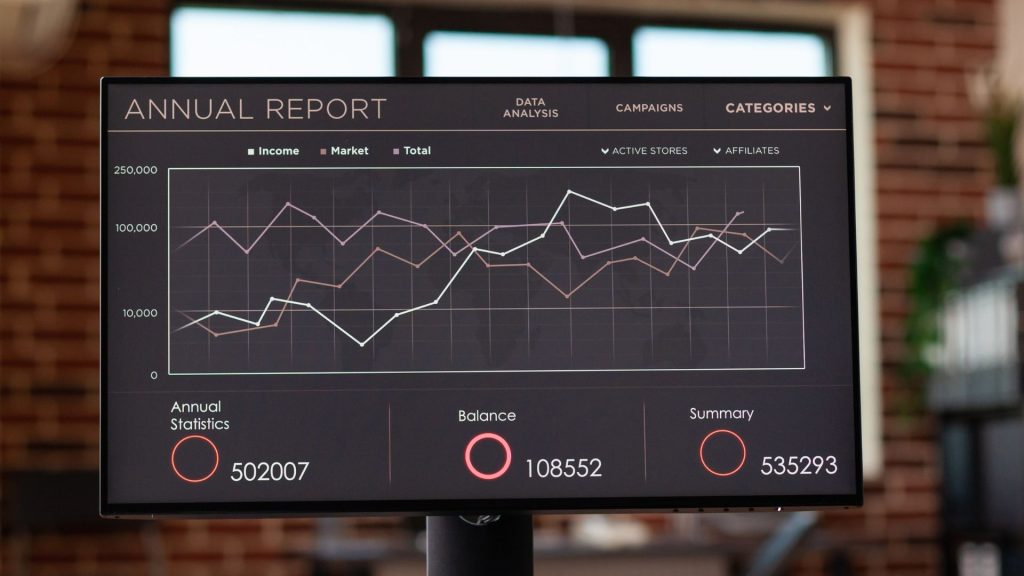
Introduction
In today’s fast-paced business environment, real-time data analytics has become a critical tool for organizations aiming to make swift and informed decisions. Machine Learning (ML), a subset of artificial intelligence, is revolutionizing real-time data analytics by enabling systems to learn from data patterns and make predictions without explicit programming. ML algorithms process vast amounts of data at high speeds, uncovering insights that were previously unattainable.
This article explores how Machine Learning enhances real-time data analytics, the benefits it offers to businesses, and the future trends shaping this dynamic field.
The Importance of Real-Time Data Analytics
Real-time data analytics involves processing and analyzing data as it becomes available, allowing businesses to react promptly to new information. This capability is essential for:
- Improving Customer Experience: Responding to customer behaviors and preferences in real-time enhances satisfaction and loyalty.
- Optimizing Operations: Immediate insights enable organizations to adjust processes for efficiency and cost savings.
- Risk Management: Detecting anomalies or potential issues as they occur helps mitigate risks promptly.
- Competitive Advantage: Swift decision-making based on current data positions businesses ahead of competitors.
However, handling and making sense of the vast streams of real-time data is a significant challenge that ML is uniquely positioned to address.
Role of Machine Learning in Real-Time Data Analytics
Machine Learning enhances real-time data analytics through several key functions:
Real-Time Data Processing
ML algorithms can process and analyze data streams in real-time, identifying patterns and trends as they emerge. This immediate analysis is crucial for time-sensitive decision-making.
Predictive Analytics
By learning from historical and current data, ML models predict future events or behaviors, allowing businesses to anticipate needs and proactively address potential issues.
Anomaly Detection
ML systems can detect deviations from normal patterns in real-time, which is essential for fraud detection, network security, and quality control.
Automated Decision-Making
ML enables systems to make autonomous decisions based on data analysis, reducing the need for human intervention and speeding up response times.
Natural Language Processing (NLP)
NLP allows ML systems to analyze unstructured data like social media feeds or customer reviews in real-time, providing insights into public sentiment and market trends.
Benefits of ML in Real-Time Data Analytics
Integrating ML into real-time data analytics offers numerous advantages:
- Enhanced Accuracy: ML models improve over time, increasing the precision of analytics and predictions.
- Scalability: ML systems can handle growing data volumes without compromising performance.
- Cost Efficiency: Automation reduces the need for manual data processing, lowering operational costs.
- Personalization: Real-time insights enable businesses to tailor products and services to individual customer needs.
- Competitive Edge: Faster access to insights allows businesses to seize opportunities and adapt to market changes swiftly.
Key ML Technologies in Real-Time Analytics
Several Machine Learning technologies are instrumental in enhancing real-time data analytics:
Stream Processing Frameworks
Tools like Apache Kafka and Apache Flink enable the processing of real-time data streams, allowing ML models to analyze data on the fly.
Deep Learning
Deep learning models can handle complex data structures, making them suitable for tasks like image recognition and natural language processing in real-time.
Reinforcement Learning
This approach allows models to learn optimal actions through trial and error, which is valuable for dynamic environments where conditions change rapidly.
Edge Computing
Processing data at the edge of the network reduces latency, enabling real-time analytics closer to the data source, which is crucial for Internet of Things (IoT) applications.
Case Studies
Company J: Enhancing Customer Experience
Company J used ML to analyze customer interactions in real-time, allowing for immediate personalization of services. This led to a 25% increase in customer satisfaction scores.
Company K: Optimizing Supply Chain
By implementing ML-driven real-time analytics, Company K improved supply chain efficiency by 30%, reducing delays and cutting costs.
Company L: Fraud Detection
Company L deployed ML models to monitor transactions in real-time, detecting fraudulent activities immediately and reducing financial losses by 40%.
Challenges and Considerations
While ML offers significant benefits, there are challenges to address:
Data Quality and Volume
Real-time analytics require high-quality data streams. Managing and processing large volumes of data can be resource-intensive.
Latency Issues
Ensuring low latency in data processing is critical. Network delays or processing bottlenecks can hinder real-time capabilities.
Integration Complexity
Integrating ML systems with existing infrastructure can be complex, requiring specialized skills and potentially significant investments.
Security and Privacy
Real-time data often includes sensitive information. Protecting this data from breaches and ensuring compliance with regulations is essential.
Future Trends
The intersection of ML and real-time data analytics is evolving, with emerging trends including:
Artificial Intelligence of Things (AIoT)
Combining AI with IoT devices enhances real-time data processing at the edge, enabling smarter and more responsive systems.
Automated Machine Learning (AutoML)
AutoML simplifies the creation of ML models, making real-time analytics more accessible to businesses without extensive ML expertise.
Real-Time Natural Language Processing
Advancements in NLP will allow for real-time analysis of unstructured textual data, such as social media posts and customer feedback.
Quantum Computing
Quantum computing holds the potential to process data at unprecedented speeds, significantly enhancing real-time analytics capabilities.
Conclusion
Machine Learning is dramatically enhancing real-time data analytics, enabling businesses to process and analyze data instantly and make informed decisions promptly. The integration of ML into real-time analytics systems offers significant benefits, including improved accuracy, efficiency, and competitive advantage. As technology continues to advance, embracing ML in real-time data analytics will be essential for businesses aiming to thrive in a data-driven world.
Investing in ML technologies today prepares organizations for the future, where real-time insights are the cornerstone of successful business strategies.