Learn How ML is Improving Disease Prediction and Prevention
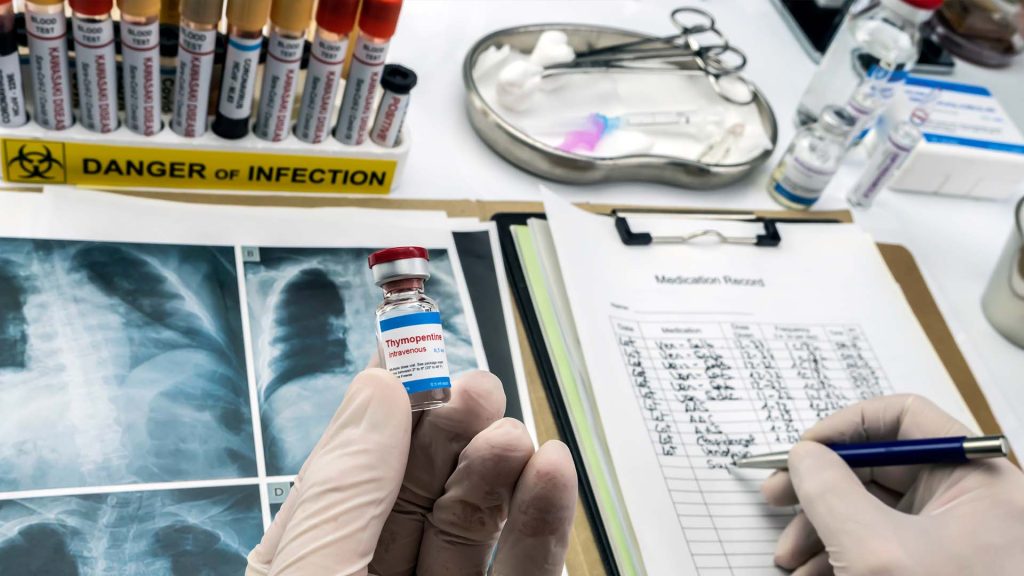
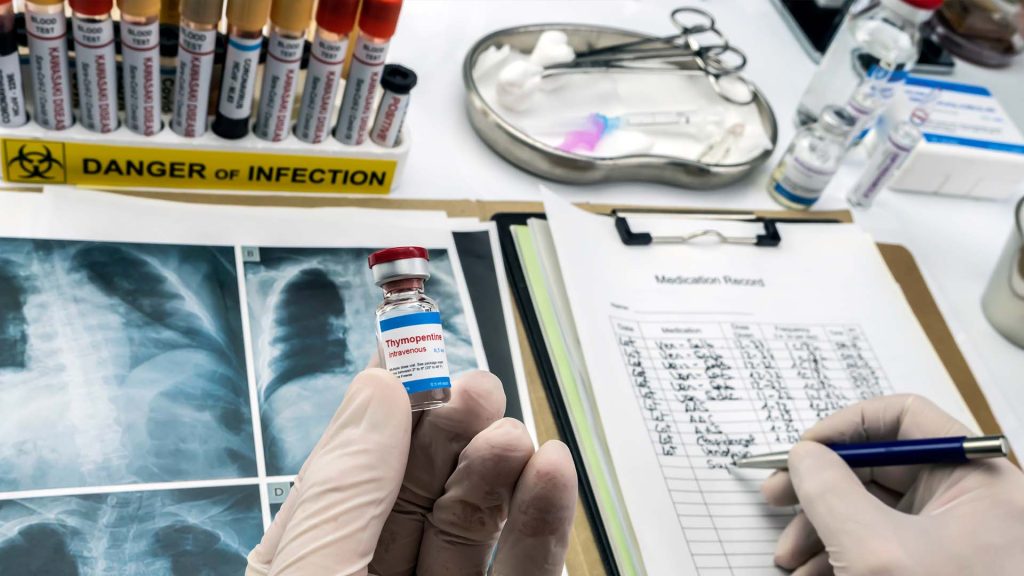
Machine Learning (ML) is transforming the healthcare industry by providing innovative solutions for disease prediction and prevention. This cutting-edge technology leverages vast amounts of data to identify patterns, predict outcomes, and provide actionable insights, ultimately improving patient care and health outcomes. In this article, we will explore how ML is being used to predict and prevent diseases, featuring 20 real-world examples that highlight the profound impact of ML in healthcare.
Introduction to Machine Learning in Healthcare
Machine Learning involves training algorithms to recognize patterns in data. These algorithms can then predict future outcomes based on historical data. In healthcare, ML models are trained using various types of data, including medical records, genetic information, and lifestyle data, to predict the likelihood of diseases and recommend preventive measures.
Benefits of Machine Learning in Disease Prediction and Prevention
- Early Detection: ML models can detect diseases at an early stage, even before symptoms appear, allowing for timely intervention.
- Personalized Medicine: ML enables the creation of personalized treatment plans based on an individual’s unique genetic makeup and health data.
- Improved Accuracy: ML algorithms can analyze large datasets with high accuracy, reducing the likelihood of misdiagnosis.
- Cost-Effective: By predicting diseases early, ML can help reduce healthcare costs associated with late-stage treatments and hospitalizations.
Real-World Examples of Machine Learning in Disease Prediction and Prevention
1. IBM Watson for Oncology
IBM Watson uses ML to analyze medical literature and patient data to provide oncologists with evidence-based treatment options. Watson for Oncology helps in predicting the best treatment plans for cancer patients by comparing their medical records with vast databases of clinical trials and medical journals.
2. Google’s DeepMind and Eye Diseases
Google’s DeepMind developed an ML system capable of diagnosing eye diseases by analyzing retinal scans. The system can detect conditions such as diabetic retinopathy and age-related macular degeneration with high accuracy.
3. PathAI and Pathology
PathAI uses ML to assist pathologists in diagnosing diseases from medical images. The platform improves the accuracy and efficiency of diagnosing cancer and other diseases by analyzing pathology slides.
4. Zebra Medical Vision
Zebra Medical Vision provides radiologists with ML tools that can detect a wide range of conditions from medical imaging, including liver disease, breast cancer, and cardiovascular conditions.
5. Tempus and Precision Medicine
Tempus uses ML to analyze clinical and molecular data to deliver personalized cancer care. The platform integrates data from various sources to provide insights into the most effective treatment plans for individual patients.
6. Health Catalyst and Predictive Analytics
Health Catalyst uses ML to provide healthcare organizations with predictive analytics solutions. The platform helps in predicting patient outcomes, identifying at-risk populations, and preventing hospital readmissions.
7. Medial EarlySign and Diabetes
Medial EarlySign uses ML to predict the onset of diabetes and its complications. The platform analyzes electronic health records to identify patients at high risk of developing diabetes and provides early intervention recommendations.
8. Aidoc and Medical Imaging
Aidoc uses ML to analyze medical imaging data in real-time, helping radiologists detect acute abnormalities and prioritize cases that require immediate attention.
9. Arterys and Cardiology
Arterys uses ML to provide cardiologists with tools for analyzing cardiac MRI images. The platform helps in diagnosing heart conditions and planning treatments with greater accuracy.
10. Berg Health and Drug Discovery
Berg Health uses ML to analyze biological data and identify new drug candidates. The platform helps in predicting the effectiveness of new drugs and accelerating the drug discovery process.
11. Qure.ai and Head Injuries
Qure.ai developed an ML system that can analyze head CT scans to detect abnormalities such as intracranial hemorrhages, fractures, and midline shifts, providing critical support in emergency settings.
12. Freenome and Cancer Detection
Freenome uses ML to detect early signs of cancer through a simple blood test. The platform analyzes cell-free DNA to identify cancer biomarkers, enabling early diagnosis and treatment.
13. Butterfly Network and Portable Ultrasound
Butterfly Network developed a portable ultrasound device that uses ML to assist clinicians in diagnosing various conditions, including cardiac, lung, and abdominal issues.
14. Kheiron Medical and Breast Cancer
Kheiron Medical uses ML to improve breast cancer detection in mammography. The platform helps radiologists identify cancerous lesions with higher accuracy, reducing the need for repeat exams.
15. Cardiologs and Arrhythmia Detection
Cardiologs uses ML to analyze ECG data and detect arrhythmias. The platform assists cardiologists in diagnosing and managing heart rhythm disorders more effectively.
16. Prognos and Disease Progression
Prognos uses ML to predict disease progression and treatment outcomes by analyzing diagnostic data. The platform helps healthcare providers make informed decisions about patient care.
17. Cleerly and Heart Disease
Cleerly uses ML to analyze coronary CT angiograms and assess heart disease risk. The platform provides detailed reports on coronary artery health, helping cardiologists plan preventive treatments.
18. Enlitic and Radiology
Enlitic uses ML to assist radiologists in interpreting medical images. The platform improves diagnostic accuracy for conditions such as lung cancer and fractures.
19. InsightRX and Pharmacology
InsightRX uses ML to personalize medication dosing for individual patients. The platform analyzes patient data to optimize drug therapy, improving treatment efficacy and reducing adverse effects.
20. Biofourmis and Remote Monitoring
Biofourmis uses ML to monitor patients remotely and predict adverse health events. The platform analyzes data from wearable devices to provide real-time insights into patient health and recommend preventive actions.
Challenges and Future Directions
While the benefits of ML in disease prediction and prevention are significant, there are also challenges that need to be addressed. These include:
- Data Privacy: Ensuring the privacy and security of patient data is paramount. Robust encryption and compliance with regulations such as GDPR and HIPAA are essential.
- Bias in Algorithms: ML models can inherit biases present in the training data, leading to unequal healthcare outcomes. Efforts must be made to ensure fairness and equity in ML algorithms.
- Integration with Healthcare Systems: Integrating ML solutions with existing healthcare systems and workflows can be complex. Collaboration between technology providers and healthcare organizations is necessary to overcome this challenge.
- Interpretability: Ensuring that ML models are interpretable and their predictions are understandable by healthcare providers is crucial for gaining trust and facilitating adoption.
Despite these challenges, the future of ML in healthcare looks promising. Continued advancements in ML algorithms, increased availability of healthcare data, and growing collaboration between technology and healthcare sectors will drive further innovations in disease prediction and prevention.
Conclusion
Machine Learning is revolutionizing the healthcare industry by improving disease prediction and prevention. From early detection of cancer to personalized treatment plans, ML is providing healthcare providers with powerful tools to enhance patient care. The 20 real-world examples highlighted in this article demonstrate the diverse applications of ML in healthcare and its potential to transform the way diseases are diagnosed and managed.
As ML technology continues to evolve, it will play an increasingly important role in predicting and preventing diseases, ultimately leading to better health outcomes for patients around the world. Embracing these innovations will be key to unlocking the full potential of ML in healthcare and achieving a future where diseases are detected early and prevented effectively.
For more information and updates on how ML is transforming healthcare, visit our website at tabsgi.com.