See How ML Enhances Customer Satisfaction in E-Commerce
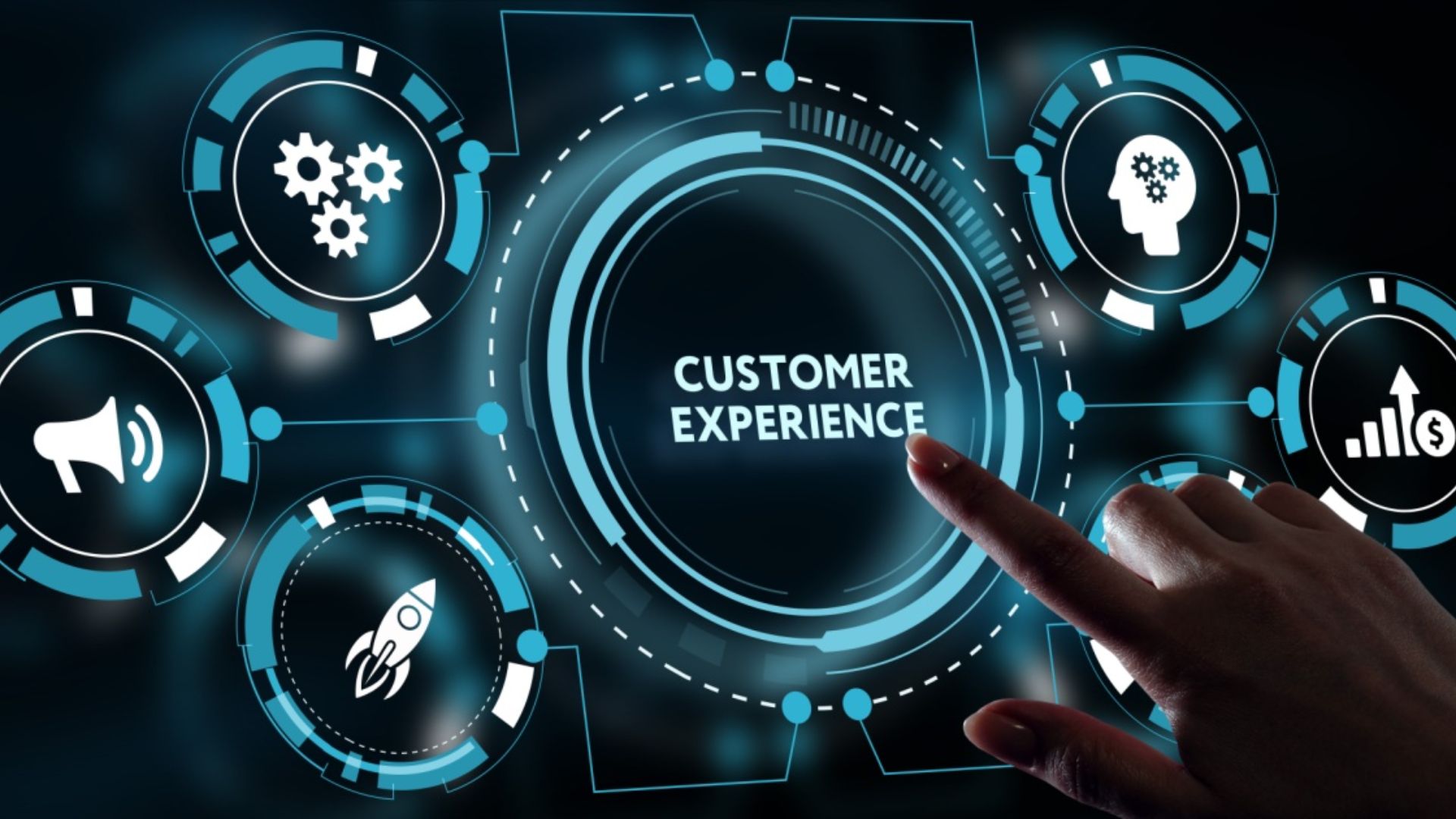
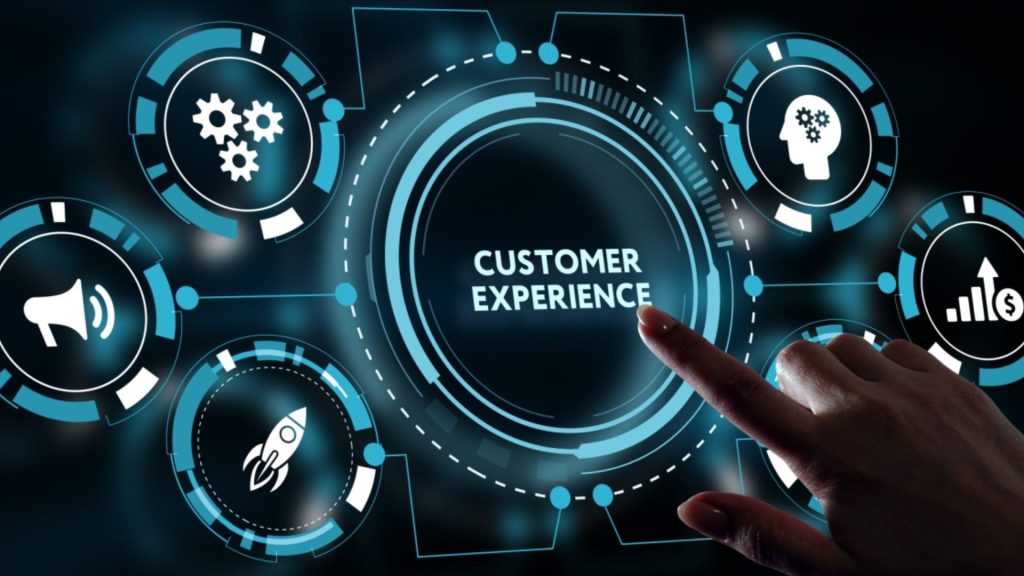
See How ML Enhances Customer Satisfaction in E-Commerce
#ML #Ecommerce #CustomerExperience
Introduction
In today’s competitive digital marketplace, customer satisfaction is paramount to building brand loyalty and driving long-term growth. Machine Learning (ML) offers e-commerce businesses the tools to understand customer preferences, personalize interactions, and streamline shopping experiences. By analyzing vast amounts of data—from browsing patterns and purchase histories to customer feedback—ML algorithms deliver insights that help retailers anticipate needs, improve product recommendations, and enhance overall satisfaction.
This article explores how ML is transforming e-commerce customer experiences, the benefits it provides, and the emerging trends that will shape the future of online retail.
Key Applications of ML in E-Commerce
ML-driven solutions address multiple facets of the e-commerce customer journey:
Personalized Product Recommendations
ML models analyze browsing history, purchase records, and user demographics to suggest products that align with individual tastes. Tailored recommendations increase conversion rates, basket sizes, and repeat visits.
Dynamic Pricing
By assessing market conditions, customer demand, and competitor prices, ML algorithms determine optimal price points in real-time. This ensures that pricing strategies remain competitive while meeting profitability goals.
Intelligent Search and Navigation
Natural Language Processing (NLP) and computer vision allow ML-driven search engines to understand user queries more accurately. Shoppers find relevant products faster, reducing frustration and improving satisfaction.
Inventory and Supply Chain Optimization
Predictive analytics help retailers maintain optimal stock levels, ensuring popular items are readily available. Timely deliveries and fewer stockouts lead to happier customers.
Proactive Customer Support
ML-powered chatbots and recommendation engines resolve common inquiries, guide customers through the sales funnel, and offer post-purchase support, all while reducing response times and improving service quality.
Benefits of ML for Customer Satisfaction
Integrating ML into e-commerce operations yields multiple advantages:
- Increased Personalization: ML tailors product recommendations, promotions, and content to individual customer preferences, fostering deeper engagement.
- Improved Convenience: Faster search results, intuitive navigation, and proactive support make online shopping more enjoyable and stress-free.
- Greater Loyalty and Retention: Meeting customer expectations leads to repeat business, positive reviews, and stronger brand loyalty.
- Data-Driven Decisions: Continuous analysis of customer data informs strategic decisions on product assortments, pricing, and marketing campaigns.
- Resource Efficiency: Automated processes reduce manual labor, cut operational costs, and free up staff to focus on high-value customer interactions.
Key ML Technologies in E-Commerce
Several ML technologies power enhanced customer experiences:
Collaborative Filtering
By identifying similarities among users or products, collaborative filtering suggests items popular with like-minded shoppers, refining recommendations over time.
Content-Based Filtering
ML algorithms analyze product attributes—such as category, brand, or style—to recommend items that share characteristics with a user’s previously liked items.
Natural Language Processing (NLP)
NLP enhances search functionality, enabling the platform to understand user queries in everyday language, interpret product reviews, and refine product descriptions.
Computer Vision
Image recognition allows visual search features, where customers upload images to find similar products, improving engagement and discovery.
Case Studies
Online Retailer A: Enhanced Recommendations
Retailer A implemented ML-driven recommendations, increasing sales from suggested products by 25%. Customers reported greater satisfaction due to discovering new, relevant items.
Marketplace B: Improved Search Experience
Marketplace B integrated NLP-based search, reducing search time by 15%. Shoppers found desired products faster and were more likely to complete purchases.
Brand C: Intelligent Chatbot Assistance
Brand C’s AI chatbot resolved common inquiries instantly, cutting response times by 40%. Customers appreciated the quick support, leading to higher satisfaction ratings.
Challenges and Considerations
While ML significantly improves the e-commerce experience, there are hurdles to consider:
Data Privacy and Security
Handling personal and payment data requires stringent privacy measures, encryption, and compliance with regulations like GDPR.
Ensuring Fairness and Bias Mitigation
ML models may inadvertently favor certain products or demographics. Regular auditing and bias mitigation strategies ensure equitable recommendations.
Model Interpretability
Understanding why certain items are recommended or priced ensures transparency, helping build user trust and improving acceptance.
Infrastructure and Scalability
Implementing ML solutions at scale requires robust infrastructure, including data pipelines, computing resources, and skilled talent.
Future Trends in ML-Driven E-Commerce
As ML evolves, the e-commerce landscape will continue to transform:
Voice Commerce
NLP-powered voice interfaces will allow shoppers to search, compare, and purchase using voice commands, simplifying the buying process.
Augmented and Virtual Reality (AR/VR)
ML-driven AR/VR experiences let customers visualize products in their environment, improving confidence and reducing return rates.
Context-Aware Experiences
Future ML models will adapt recommendations based on context—like location, time of day, or device type—further personalizing the shopping journey.
Hyper-Personalization
With more data and advanced ML techniques, recommendations will become even more granular, offering hyper-personalized experiences that anticipate user needs.
Conclusion
Machine Learning empowers e-commerce businesses to deliver personalized, efficient, and engaging customer experiences. By leveraging ML insights to drive product recommendations, pricing strategies, search enhancements, and proactive support, online retailers can build stronger relationships and boost satisfaction levels.
As ML technologies advance, e-commerce platforms that embrace data-driven innovations will set new standards for customer experience, paving the way for long-term success in the digital marketplace.