AI’s Role in Enhancing Personalized Learning Experiences
AI’s Role in Enhancing Personalized Learning Experiences
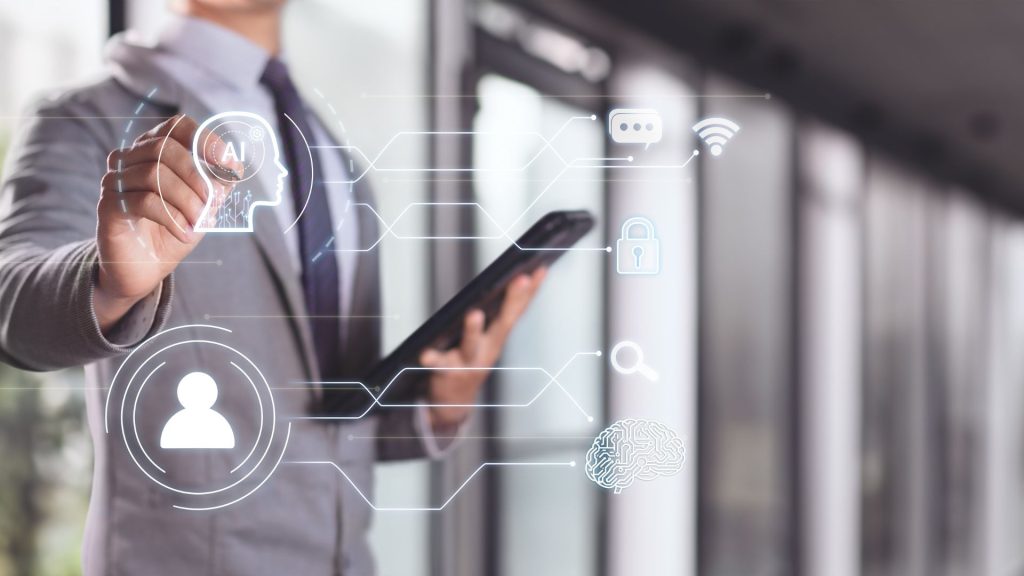
Introduction
Education is evolving rapidly, driven by technological advancements that have transformed traditional learning models into more dynamic and individualized experiences. One of the key technologies behind this transformation is Artificial Intelligence (AI). By leveraging AI, the education sector—often referred to as EdTech—is making significant strides in creating personalized learning experiences that cater to the unique needs, strengths, and weaknesses of each learner. AI-powered systems can analyze data, provide real-time feedback, and adapt content to optimize the learning process, making education more efficient and engaging. This article delves into AI’s role in enhancing personalized learning experiences, the technologies involved, benefits, challenges, and examples of AI in EdTech.
The Concept of Personalized Learning
What is Personalized Learning?
Personalized learning is an educational approach that tailors learning experiences to the individual learner’s needs, preferences, pace, and abilities. Unlike the traditional one-size-fits-all model, personalized learning ensures that each student progresses according to their learning style and preferences.
- Adaptive Content: Materials are adjusted based on student performance, ensuring they receive content that is neither too easy nor too challenging.
- Individualized Feedback: Learners receive feedback that is specific to their progress and areas of improvement.
- Student-Centered Approach: Focuses on the interests, strengths, and weaknesses of each student to foster a more engaging and effective learning experience.
AI Technologies Enabling Personalized Learning
Machine Learning Algorithms
Machine Learning (ML) is at the core of personalized learning, enabling systems to learn from student interactions and adjust content accordingly.
- Data Analysis: ML algorithms analyze student performance data to understand learning patterns and preferences.
- Predictive Analytics: Predicts which areas a student may struggle with in the future and provides tailored content to address those gaps.
- Adaptive Assessments: Creates assessments that adjust in real-time based on the learner’s responses, ensuring they are challenged at the appropriate level.
Natural Language Processing (NLP)
NLP is used to understand and process human language, making learning interactions more intuitive and personalized.
- Intelligent Tutoring Systems: NLP-powered systems interact with students using conversational language, providing explanations and answering questions in a way that mimics human tutors.
- Content Analysis: NLP helps in analyzing and summarizing learning materials, making content more digestible for students.
- Language Translation: Breaks language barriers by translating content into the learner’s preferred language.
Recommender Systems
AI-based recommender systems suggest content, activities, or courses based on the student’s learning history, interests, and performance.
- Learning Path Recommendations: Suggests personalized learning paths that align with the learner’s goals and needs.
- Course Recommendations: Proposes courses based on individual interests and career aspirations.
Intelligent Tutoring Systems (ITS)
ITS are AI-powered tools that provide personalized guidance and support to learners, replicating the role of a human tutor.
- Individualized Instruction: Adapts instructional content in real-time to match the student’s current knowledge level.
- Interactive Learning: Engages students through interactive problem-solving and immediate feedback.
Chatbots and Virtual Assistants
AI chatbots and virtual assistants support students by answering questions, providing reminders, and offering feedback on assignments.
- 24/7 Support: Available anytime to answer student questions, ensuring learning is not limited by classroom hours.
- Automated Feedback: Provides instant feedback on quizzes, essays, and other assignments.
Benefits of AI in Personalized Learning
Adaptive Learning
AI enables adaptive learning, where content is dynamically adjusted to meet the learner’s needs. This ensures that students receive appropriate challenges and can focus on areas where they need the most improvement.
- Real-Time Adjustments: Content adapts instantly based on the learner’s progress and performance.
- Targeted Interventions: AI identifies areas of difficulty and provides targeted interventions, helping students overcome obstacles more efficiently.
Enhanced Student Engagement
Personalized learning fosters greater engagement by catering to students’ interests and providing content that aligns with their individual learning styles.
- Gamification: AI-powered platforms use gamification techniques to make learning fun and motivating.
- Relevant Content: Tailored content is more relatable to students, making the learning experience more meaningful.
Efficient Use of Teacher Resources
AI helps educators focus on high-value activities by automating routine tasks, such as grading and administrative duties.
- Automated Grading: AI can assess quizzes and assignments, freeing up teachers’ time for personalized student interactions.
- Identifying Learning Gaps: AI tools highlight students who need additional support, allowing teachers to intervene more effectively.
Data-Driven Insights
AI provides data-driven insights into student performance, helping educators make informed decisions about teaching strategies and interventions.
- Performance Analytics: Teachers can track individual and class progress through dashboards that present data visually.
- Predictive Insights: Predicts which students are at risk of falling behind and suggests proactive measures.
Learning Without Boundaries
AI-powered personalized learning platforms enable students to learn at their own pace, anytime and anywhere, making education more flexible and accessible.
- Self-Paced Learning: Students can take as much time as they need to understand complex concepts, without feeling pressured by class schedules.
- Global Access: Learners from different parts of the world can access quality education without physical constraints.
Real-World Examples of AI in Personalized Learning
Duolingo
Duolingo is an AI-powered language learning platform that provides personalized language lessons to millions of users worldwide.
- Adaptive Learning Path: AI tracks the learner’s progress and adjusts lessons based on strengths and weaknesses.
- Gamified Experience: Duolingo uses gamification techniques like points, badges, and challenges to keep users motivated.
By personalizing the learning experience, Duolingo helps users achieve language proficiency at their own pace.
Coursera and edX
Online learning platforms Coursera and edX use AI to enhance the learning experience through personalized course recommendations and adaptive assessments.
- Course Recommendations: AI suggests courses based on the learner’s interests, previous courses, and career aspirations.
- Interactive Assessments: Adaptive quizzes adjust the difficulty level based on learner responses, ensuring that students are appropriately challenged.
These platforms make high-quality education accessible to a global audience while providing a personalized learning journey.
Khan Academy
Khan Academy uses AI to provide a personalized learning dashboard for each student, allowing them to learn at their own pace.
- Adaptive Exercises: AI recommends practice exercises tailored to the student’s learning level and progress.
- Teacher Support: Teachers receive insights into student progress, enabling them to offer targeted support where needed.
With AI, Khan Academy aims to close the educational gap by offering personalized support to learners across various subjects.
Carnegie Learning’s MATHia
MATHia is an AI-powered personalized math tutoring system designed by Carnegie Learning to provide individualized math instruction.
- Real-Time Feedback: Offers immediate feedback to students based on their responses, helping them learn from mistakes instantly.
- Tailored Problem-Solving: Adjusts math problems in real-time to meet the needs of individual students, ensuring they grasp concepts before moving forward.
MATHia’s AI-driven approach helps students master complex mathematical concepts in a structured and personalized manner.
Challenges and Considerations
Data Privacy and Security
AI-powered personalized learning platforms collect vast amounts of student data, raising concerns about privacy and security.
- Data Protection: Institutions must ensure that student data is protected from unauthorized access and breaches.
- Compliance with Regulations: Adherence to privacy regulations such as GDPR and FERPA is essential to safeguard student information.
Algorithmic Bias
AI algorithms may inadvertently incorporate biases present in the data, leading to unfair outcomes for certain student groups.
- Fairness and Equity: It’s crucial to ensure that AI models are trained on diverse datasets to avoid bias.
- Transparent Decision-Making: AI systems must be transparent in their decision-making processes to avoid unintended consequences.
Teacher Training and Adoption
Educators need to be trained to effectively use AI-powered tools and integrate them into their teaching practices.
- Professional Development: Providing teachers with training on how to leverage AI tools to enhance student learning.
- Resistance to Change: Addressing resistance from educators who may be skeptical about incorporating AI into the classroom.
Technical Challenges
Implementing AI in personalized learning comes with technical challenges, including infrastructure requirements and integration with existing systems.
- Scalability: AI systems must be scalable to accommodate large numbers of students without compromising performance.
- Integration: Seamless integration with existing learning management systems (LMS) is crucial for widespread adoption.
Ethical Considerations
The use of AI in personalized learning raises ethical questions about the role of technology in education.
- Human Oversight: Ensuring that AI is used to support, not replace, human educators.
- Student Autonomy: Balancing automation with student choice and autonomy in the learning process.
Future Trends in AI-Driven Personalized Learning
Emotion AI
Emotion AI, also known as affective computing, is expected to play a significant role in personalized learning by understanding and responding to student emotions.
- Emotion Detection: AI systems will be able to detect emotions such as frustration or confusion and adjust content accordingly.
- Adaptive Support: Providing emotional support and encouragement to help students overcome learning challenges.
AI-Driven Peer Learning
AI will facilitate peer learning by connecting students with similar learning needs and fostering collaborative problem-solving.
- Study Groups: AI will create virtual study groups based on shared interests and learning goals.
- Collaborative Learning Activities: Encouraging teamwork and group projects to enhance the learning experience.
Integration with Augmented Reality (AR) and Virtual Reality (VR)
AI-powered AR and VR technologies will enhance personalized learning by providing immersive and interactive experiences.
- Immersive Learning Environments: AR/VR will allow students to explore complex concepts in a hands-on virtual environment.
- Personalized Virtual Labs: AI will tailor virtual lab experiences to match the learner’s progress and areas of interest.
Lifelong Learning and Skill Development
AI will play a crucial role in supporting lifelong learning by providing personalized skill development opportunities throughout an individual’s career.
- Micro-Learning Modules: AI will recommend short, targeted learning modules to help learners acquire specific skills quickly.
- Career Pathways: Personalized learning paths will help individuals navigate career transitions and acquire the skills needed for new roles.
Greater Collaboration Between AI and Human Educators
The future of AI in education will see closer collaboration between AI systems and human educators to provide a more holistic learning experience.
- Teacher-AI Partnership: AI will assist educators in identifying areas where students need additional support, allowing teachers to focus on meaningful interactions.
- Augmented Teaching: AI tools will provide teachers with data-driven insights and resources to enhance their teaching strategies.
Conclusion
Artificial Intelligence is playing a transformative role in enhancing personalized learning experiences by providing tailored content, real-time feedback, and adaptive learning pathways. Through technologies such as machine learning, natural language processing, intelligent tutoring systems, and chatbots, AI is making education more engaging, efficient, and accessible. While there are challenges related to data privacy, algorithmic bias, and teacher adoption, the benefits of AI in personalized learning far outweigh the drawbacks. By addressing these challenges and embracing future trends such as emotion AI, AR/VR integration, and lifelong learning support, AI has the potential to reshape the education landscape and make personalized learning a reality for all learners, regardless of their background or location. The future of education is here, and it is personalized, adaptive, and powered by AI.