See How ML is Transforming Personalized Health Recommendations
See How ML is Transforming Personalized Health Recommendations
#ML #Health #Tech
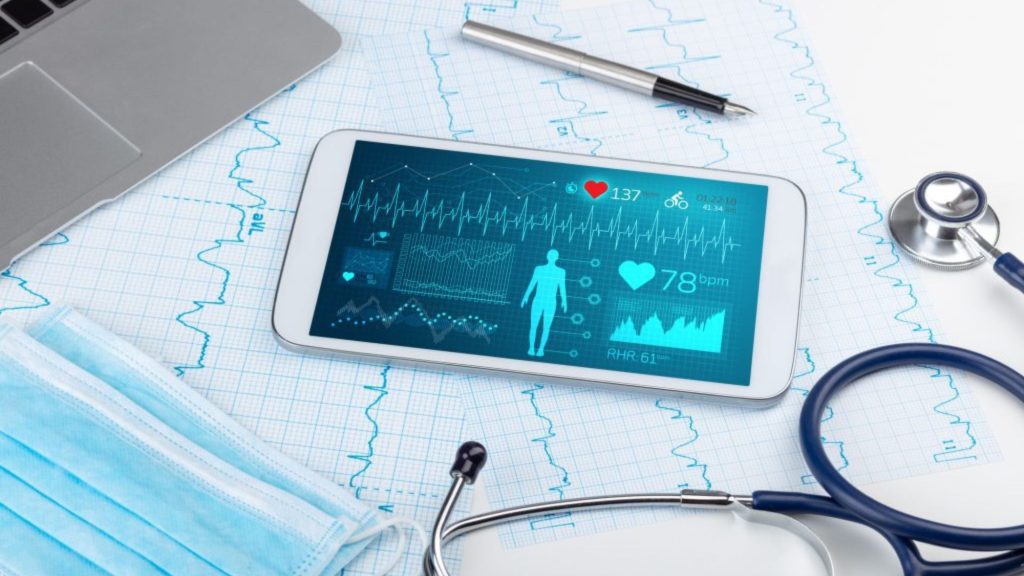
Introduction
The healthcare industry is experiencing a paradigm shift as advances in Machine Learning (ML) enable highly personalized and data-driven health recommendations. Rather than relying solely on generalized guidelines, ML leverages vast amounts of patient data—ranging from genetics and medical histories to lifestyle factors and wearable device readings—to provide individualized insights. This approach allows healthcare providers to tailor prevention strategies, treatment plans, and wellness recommendations, ultimately improving patient outcomes and overall health management.
This article explores how ML is transforming the delivery of personalized health recommendations, the benefits it offers, and the future trends shaping this innovative field.
Applications of ML in Personalized Health
ML technologies are being applied across various aspects of healthcare to enhance the precision and relevance of health recommendations:
Predictive Risk Assessment
ML algorithms analyze patient data to predict the likelihood of developing certain conditions. For example, models can forecast the risk of cardiovascular disease, prompting early interventions such as dietary adjustments, increased exercise, or preventive medications.
Genomic-Based Recommendations
By integrating genomic data, ML can help identify genetic predispositions to specific health issues. Personalized recommendations might include targeted screening schedules, tailored drug therapies, or lifestyle modifications to mitigate genetic risks.
Lifestyle and Behavioral Guidance
Wearable devices and health apps collect real-time data on activity levels, sleep patterns, and nutrition. ML models process these inputs to generate personalized coaching tips, stress management techniques, and fitness goals that evolve with the patient’s progress.
Medication Management
ML-driven tools analyze patient history, medication interactions, and side effects to recommend optimal dosing schedules or alternative treatments, improving medication adherence and minimizing adverse reactions.
Chronic Disease Management
For patients with chronic conditions like diabetes or hypertension, ML-enabled monitoring systems can adjust recommendations dynamically. Personalized alerts or reminders ensure that patients maintain better control over their conditions.
Benefits of ML in Personalized Health Recommendations
Integrating ML into personalized health recommendations offers numerous advantages:
- Improved Outcomes: Tailored advice increases the likelihood of positive health changes and better long-term results.
- Early Intervention: Predictive analytics enable proactive measures that can prevent disease progression and reduce healthcare costs.
- Enhanced Patient Engagement: Individualized recommendations motivate patients, making them more likely to adhere to suggested interventions.
- Efficient Care Delivery: Automated data analysis frees up healthcare professionals to focus on patient care rather than manual data processing.
- Scalable Solutions: ML-driven platforms can handle large patient populations, ensuring that personalized care remains accessible and affordable.
Key ML Technologies in Personalized Health
Several ML technologies are instrumental in shaping personalized health recommendations:
Natural Language Processing (NLP)
NLP extracts insights from patient records, clinician notes, and patient-reported outcomes, helping models understand context, symptoms, and patient histories.
Deep Learning
Complex neural networks identify patterns in large, unstructured datasets, such as imaging studies or genomic data, providing actionable recommendations and diagnostics.
Federated Learning
This approach trains models on decentralized data—like patient records stored at multiple hospitals—without sharing sensitive information. It enhances privacy and data security.
Reinforcement Learning
Reinforcement learning algorithms continually refine recommendations based on patient feedback, resulting in adaptive and responsive health guidance.
Case Studies
Hospital A: Personalized Nutrition Plans
By analyzing patient dietary logs and biomarkers, Hospital A’s ML platform generated personalized meal plans. Patients experienced a 15% improvement in metabolic indicators and higher adherence to dietary guidelines.
Clinic B: Early Diabetes Interventions
Clinic B used ML to identify high-risk prediabetic patients and provided targeted lifestyle recommendations. This proactive approach reduced diabetes onset rates by 20% over two years.
Health App C: Dynamic Workout Adjustments
App C’s ML-driven fitness coach adapted exercise routines based on user feedback, wearables data, and performance metrics. Users reported a 25% increase in workout consistency and improved cardiovascular health.
Challenges and Considerations
While ML offers significant benefits, there are challenges to address:
Data Privacy and Security
Handling sensitive health data requires stringent privacy measures, adherence to regulations like HIPAA, and robust cybersecurity protocols.
Data Quality and Bias
Models depend on accurate, representative data. Biased or incomplete datasets can lead to inequitable recommendations or reduced effectiveness.
Interpretability
Clinicians and patients need to understand how ML models make decisions. Ensuring transparency helps build trust and improve adoption of these technologies.
Integration into Healthcare Systems
Implementing ML solutions requires adapting workflows, training staff, and ensuring compatibility with existing electronic health records (EHRs) and IT infrastructure.
Future Trends in ML and Personalized Health
The future of ML in healthcare is poised for further innovation:
Multi-Omics Integration
Combining genomic, proteomic, metabolomic, and environmental data will offer holistic health insights, guiding truly personalized preventive care.
Real-Time Monitoring
Continuous data from wearable sensors and connected devices will enable ML models to adjust recommendations as patients’ conditions change throughout the day.
AI-Driven Clinical Decision Support
As ML models become more sophisticated, they will provide actionable insights to clinicians at the point of care, streamlining decision-making processes.
Global Accessibility
Scalable, cloud-based ML platforms will make personalized health recommendations more accessible worldwide, bridging gaps in care across diverse populations.
Conclusion
Machine Learning is revolutionizing how healthcare providers deliver personalized health recommendations. By leveraging patient data, ML models offer early interventions, tailored treatments, and ongoing support that improve patient outcomes and well-being. As these technologies evolve, overcoming challenges related to data privacy, integration, and interpretability will be critical in unlocking their full potential.
Embracing ML-driven personalized health recommendations represents a significant step towards a future where healthcare is proactive, patient-centric, and continuously improving.