How Machine Learning Optimizes Warehouse Management Systems
How Machine Learning Optimizes Warehouse Management Systems
#ML #WarehouseManagement #Logistics
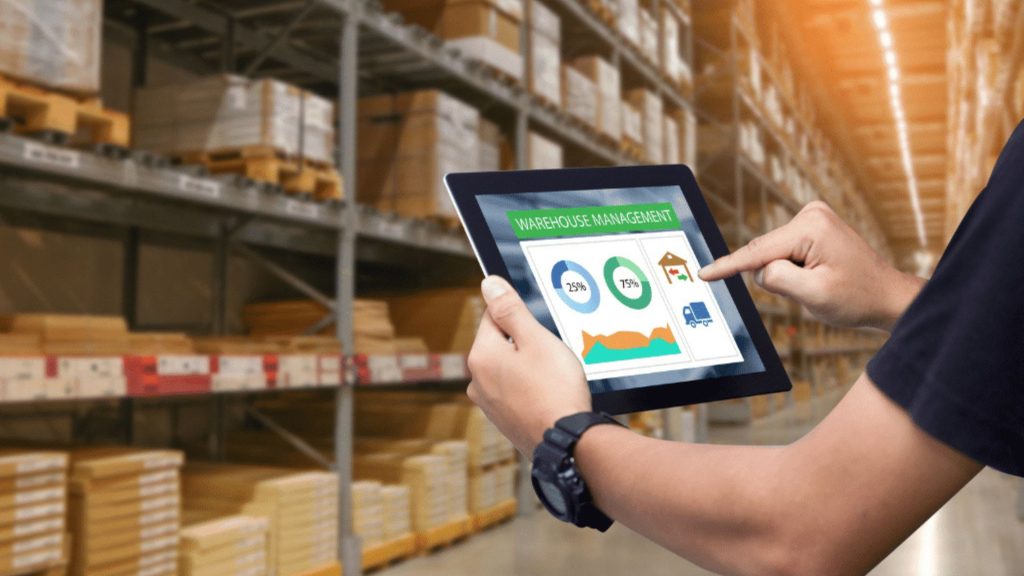
Introduction
In today’s fast-paced global economy, the efficiency of warehouse management systems (WMS) plays a pivotal role in the success of supply chain operations. With the advent of e-commerce and the increasing demand for faster delivery times, warehouses are under immense pressure to optimize their operations. Enter Machine Learning (ML), a subset of artificial intelligence (AI) that enables systems to learn and improve from experience without being explicitly programmed. ML is revolutionizing warehouse management by providing intelligent solutions to complex logistical challenges.
This article delves into how Machine Learning is optimizing warehouse management systems, transforming logistics, and paving the way for more efficient and responsive supply chains.
The Challenges in Warehouse Management
Warehouses are dynamic environments where numerous processes occur simultaneously. Managing these processes efficiently is critical but fraught with challenges, including:
- Inventory Management: Keeping track of stock levels, avoiding overstocking or stockouts, and managing perishable goods.
- Order Fulfillment: Ensuring orders are picked, packed, and shipped accurately and promptly.
- Labor Management: Scheduling staff effectively to meet fluctuating demand while controlling labor costs.
- Space Optimization: Utilizing warehouse space efficiently to maximize storage capacity and facilitate easy access to goods.
Traditional methods often fall short in addressing these challenges due to their inability to process vast amounts of data and adapt to changing conditions in real-time.
Role of Machine Learning in Warehouse Management
Machine Learning algorithms can analyze large datasets to identify patterns and make predictions, enabling warehouses to operate more efficiently. Key applications include:
Predictive Analytics
ML models can predict future inventory needs by analyzing historical sales data, seasonal trends, and market indicators. This helps in maintaining optimal stock levels and reducing holding costs.
Demand Forecasting
Accurate demand forecasting allows warehouses to anticipate customer needs and adjust their operations accordingly. ML algorithms consider various factors like past sales, promotions, and external events to forecast demand.
Inventory Optimization
By predicting demand, ML helps in optimizing inventory levels, reducing excess stock, and minimizing the risk of stockouts. It ensures the right products are available at the right time.
Route Optimization
For order picking and deliveries, ML algorithms can determine the most efficient routes, reducing travel time and fuel consumption, and improving delivery times.
Labor Scheduling
ML can forecast labor requirements based on predicted workload, helping managers schedule staff more effectively and control labor costs.
ML Techniques Applied in Warehouse Management
Various ML techniques are employed to address specific warehouse management challenges:
Supervised Learning
Used for predictive tasks where the algorithm learns from labeled datasets. Applications include demand forecasting and inventory management.
Unsupervised Learning
Helps in identifying patterns or groupings within data without pre-labeled outcomes. Useful in customer segmentation and detecting anomalies in warehouse operations.
Reinforcement Learning
Algorithms learn optimal actions through trial and error to maximize a reward function. Applied in robotic process automation and dynamic routing.
Deep Learning
Utilizes neural networks with multiple layers to model complex patterns. Used in image recognition for sorting and quality control processes.
Case Studies
Company A: Improved Inventory Turnover
By implementing ML-driven demand forecasting, Company A reduced excess inventory by 20% and improved inventory turnover rates, leading to significant cost savings.
Company B: Enhanced Picking Efficiency
Company B utilized ML algorithms for route optimization within the warehouse, resulting in a 15% reduction in order picking time and improved delivery times.
Company C: Optimized Space Utilization
By analyzing storage patterns, ML helped Company C reorganize its warehouse layout, maximizing space utilization by 25% and improving accessibility to high-turnover items.
Benefits of ML in Warehouse Management
The integration of ML into warehouse management systems offers numerous benefits:
- Cost Reduction: Optimized inventory levels and efficient operations reduce carrying costs and labor expenses.
- Improved Efficiency: Automated processes and intelligent decision-making streamline operations.
- Better Customer Satisfaction: Accurate demand forecasting and timely deliveries enhance customer experience.
- Real-Time Decision Making: ML algorithms process data quickly, allowing for immediate responses to changing conditions.
Challenges and Considerations
While ML offers significant advantages, there are challenges to consider:
Data Quality and Quantity
ML algorithms require large amounts of high-quality data. Inaccurate or insufficient data can lead to poor predictions and decisions.
Integration with Existing Systems
Incorporating ML into existing WMS requires compatibility and may involve significant changes to current processes.
Security and Privacy Concerns
Protecting sensitive data used in ML models is crucial to prevent unauthorized access and data breaches.
Workforce Adaptation
Employees may need training to work alongside new technologies, and there may be resistance to change.
Future Trends
The future of warehouse management is set to be shaped by further advancements in ML and related technologies:
AI and Robotics
Integration of AI-powered robots for tasks like picking, packing, and sorting will increase efficiency and reduce human error.
IoT and Data Collection
The Internet of Things (IoT) will enhance data collection through connected devices, providing richer datasets for ML algorithms.
Autonomous Vehicles and Drones
Use of autonomous vehicles and drones for internal transport and inventory checks will further streamline warehouse operations.
Conclusion
Machine Learning is transforming warehouse management systems by providing intelligent solutions to complex logistical challenges. By leveraging ML, warehouses can operate more efficiently, reduce costs, and improve customer satisfaction. As technology continues to evolve, the integration of ML into warehouse operations will become increasingly essential for businesses aiming to stay competitive in the global market.
Embracing ML technologies is not just an option but a necessity for modern warehouses seeking to optimize their operations and adapt to the ever-changing demands of the logistics industry.