Machine Learning for Business: Why Your Company Needs It Now
Machine Learning for Business: Why Your Company Needs It Now
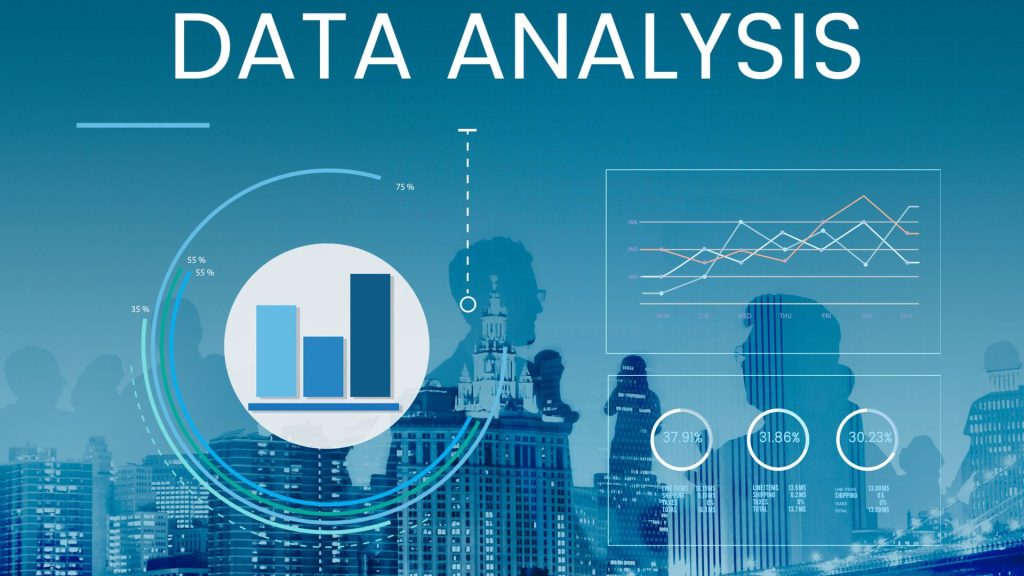
In the modern business landscape, machine learning (ML) is not just an advantage; it is a necessity. This transformative technology is reshaping industries by enabling smarter decision-making, enhancing customer experiences, and streamlining operations. Here, we explore the multifaceted benefits of machine learning and why your company should embrace it now.
Unpacking Machine Learning
What is Machine Learning?
Machine learning is a subset of artificial intelligence (AI) that enables systems to learn from data, identify patterns, and make decisions with minimal human intervention. ML models improve over time, adapting to new data without being explicitly programmed to do so.
The Business Benefits of Machine Learning
Enhanced Data Insights
One of the primary advantages of machine learning is its ability to sift through massive amounts of data and extract actionable insights. Businesses can use ML to uncover trends and patterns that are not obvious to human analysts, helping them to make more informed decisions.
Automation of Routine Tasks
Machine learning automates repetitive tasks, freeing up employees for higher-value work. This includes everything from customer service responses to inventory management, which can all be optimized with ML to reduce errors and increase efficiency.
Improved Customer Experiences
ML algorithms can personalize customer interactions based on their behavior and preferences, leading to more effective marketing and higher customer satisfaction. This personalization can range from product recommendations to customized marketing messages, all tailored to the individual customer’s needs.
Predictive Analytics for Forecasting
Machine learning excels at predictive analytics, which involves using historical data to predict future outcomes. Businesses use ML to forecast sales trends, demand spikes, and even potential supply chain disruptions, allowing them to plan more effectively and mitigate risks.
Implementing Machine Learning in Your Business
Starting Small
For businesses new to machine learning, it’s advisable to start small. Identify areas where ML can have an immediate impact, such as customer service or sales forecasting, and gradually expand as you see results.
Choosing the Right Tools and Partners
Selecting the right machine learning tools and partners is crucial. Look for platforms and consultants with expertise specific to your industry, and ensure they offer scalable solutions that can grow with your business.
Training and Development
Investing in training for your team is essential to fully leverage machine learning. This includes not only technical training but also training on how to interpret ML insights and integrate them into business strategies.
Overcoming Challenges
Data Privacy and Security
Implementing ML requires handling sensitive data. Ensuring the privacy and security of this data is paramount, and businesses must adhere to regulations and best practices to protect their information.
Managing Costs
While machine learning can be cost-effective in the long run, initial investments and ongoing maintenance can be significant. Careful planning and management are required to ensure that the costs are aligned with the expected benefits.
The Future of Machine Learning in Business
Looking ahead, machine learning is set to become even more integral to business operations. Advances in technology will make ML tools more accessible and affordable, enabling even small businesses to benefit from AI-driven insights and automation.
Machine learning offers a competitive edge that no modern business can afford to ignore. By automating processes, enhancing data analysis, and improving customer engagement, ML not only boosts efficiency but also drives innovation. The time to adopt machine learning is now, as it continues to be a key differentiator in the digital age.