How AI is Transforming Telehealth Services
How AI is Transforming Telehealth Services
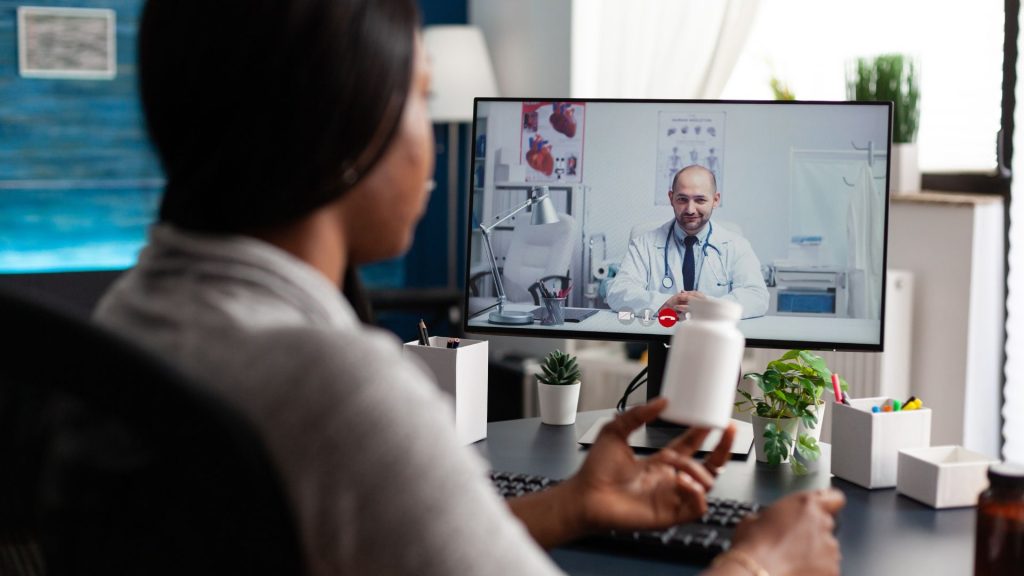
Introduction
The healthcare industry is undergoing a significant transformation driven by technological advancements. Among these, Artificial Intelligence (AI) stands out as a game-changer, especially in the realm of telehealth services. Telehealth, the delivery of healthcare services through digital communication technologies, has gained prominence due to its potential to increase accessibility, reduce costs, and improve patient outcomes. AI enhances telehealth by providing intelligent tools that facilitate remote diagnosis, personalized care, predictive analytics, and more. This article explores how AI is transforming telehealth services, the technologies involved, benefits, challenges, and real-world examples of AI-powered telehealth solutions.
The Rise of Telehealth
What is Telehealth?
Telehealth refers to the use of digital information and communication technologies to access healthcare services remotely. It includes:
- Virtual Consultations: Remote interactions between patients and healthcare providers via video conferencing.
- Remote Patient Monitoring: Tracking patient health data through wearable devices and sensors.
- Mobile Health (mHealth): Health services and information delivered via mobile devices.
- Health Education: Providing medical information and support remotely.
The Impact of COVID-19
The COVID-19 pandemic accelerated the adoption of telehealth services as social distancing measures necessitated remote healthcare delivery. Telehealth became essential for:
- Reducing Exposure: Minimizing the risk of virus transmission in healthcare settings.
- Continuity of Care: Ensuring patients receive ongoing medical attention despite restrictions.
- Resource Optimization: Allocating medical resources efficiently during the crisis.
The Role of AI in Telehealth
AI enhances telehealth by providing intelligent systems that augment healthcare delivery. Key areas where AI is making an impact include:
Virtual Assistants and Chatbots
AI-powered virtual assistants interact with patients to provide information, schedule appointments, and offer preliminary assessments.
- 24/7 Availability: Providing round-the-clock support to patients.
- Symptom Checking: Assessing patient symptoms and recommending next steps.
- Personalized Guidance: Offering tailored health advice based on patient data.
Remote Diagnosis and Monitoring
AI algorithms analyze data from remote monitoring devices to detect anomalies and predict health issues.
- Predictive Analytics: Forecasting health events like heart attacks or strokes.
- Anomaly Detection: Identifying irregularities in vital signs or patient behavior.
- Automated Alerts: Notifying healthcare providers of critical patient conditions.
Medical Imaging and Diagnostics
AI enhances the analysis of medical images remotely, aiding in diagnosis.
- Image Interpretation: Analyzing X-rays, MRIs, and CT scans with high accuracy.
- Tele-radiology: Enabling remote radiologists to collaborate and diagnose.
- Early Detection: Identifying diseases like cancer at initial stages.
Personalized Treatment Plans
AI systems develop customized treatment plans based on individual patient data.
- Genomic Analysis: Tailoring treatments based on genetic profiles.
- Medication Management: Optimizing drug dosages and combinations.
- Lifestyle Recommendations: Providing personalized advice on diet and exercise.
Natural Language Processing (NLP)
NLP allows AI to understand and process human language, enhancing telehealth interactions.
- Speech Recognition: Transcribing patient-provider conversations accurately.
- Language Translation: Bridging communication gaps between patients and providers.
- Sentiment Analysis: Assessing patient emotions and mental health.
Real-World Examples of AI in Telehealth
Babylon Health
Babylon Health is a UK-based company offering AI-powered telehealth services:
- Symptom Checker: An AI chatbot assesses symptoms and provides medical advice.
- Virtual Consultations: Patients can schedule video appointments with doctors.
- Health Monitoring: Tracks health metrics and offers personalized recommendations.
Babylon Health has partnered with healthcare providers worldwide, including the UK’s National Health Service (NHS), to enhance access to medical services.
Teladoc Health
Teladoc Health integrates AI into its telehealth platform:
- AI Triage: Guides patients to the appropriate level of care based on symptom assessment.
- Data Analytics: Uses AI to analyze patient data for better clinical decision-making.
- Remote Monitoring: Provides AI-driven monitoring for chronic conditions like diabetes and hypertension.
Teladoc’s AI capabilities have improved patient outcomes and reduced healthcare costs for organizations using their services.
HealthTap
HealthTap offers virtual healthcare services enhanced by AI:
- Dr. A.I.: An AI-powered virtual assistant that provides personalized health information.
- Global Medical Network: Connects patients with doctors worldwide for virtual consultations.
- AI-Powered Health Education: Delivers tailored health tips and educational content.
HealthTap’s AI solutions aim to make healthcare accessible and affordable by leveraging technology.
Ping An Good Doctor
China’s Ping An Good Doctor uses AI to enhance telehealth services:
- AI-Assisted Diagnosis: Provides preliminary diagnoses and treatment suggestions.
- Medical AI System: Analyzes patient data to support doctors in clinical decisions.
- Health Management: Offers personalized health plans based on AI analysis.
With over 300 million users, Ping An Good Doctor demonstrates the scalability of AI in telehealth.
Benefits of AI in Telehealth
Increased Accessibility
AI-powered telehealth services reach patients in remote or underserved areas, providing access to medical care regardless of geographical limitations.
Enhanced Efficiency
Automation of routine tasks and intelligent triaging reduce the workload on healthcare professionals, allowing them to focus on complex cases.
Improved Patient Outcomes
Personalized care plans and early detection of health issues lead to better patient outcomes and quality of life.
Cost Reduction
Telehealth reduces the need for physical infrastructure and lowers operational costs, making healthcare more affordable.
Data-Driven Insights
AI analyzes large datasets to provide actionable insights for healthcare providers, supporting evidence-based decision-making.
Challenges and Ethical Considerations
Data Privacy and Security
Handling sensitive medical data requires robust security measures:
- Compliance: Adhering to regulations like HIPAA and GDPR.
- Encryption: Protecting data during transmission and storage.
- Access Controls: Limiting data access to authorized personnel.
Algorithmic Bias
AI systems may inadvertently perpetuate biases present in training data:
- Fairness: Ensuring equitable treatment across different demographics.
- Transparency: Making AI decision-making processes understandable.
- Inclusive Data: Using diverse datasets to train AI models.
Regulatory Hurdles
Integrating AI into healthcare faces regulatory challenges:
- Approval Processes: Gaining regulatory approval for AI applications can be time-consuming.
- Standardization: Lack of industry-wide standards for AI in telehealth.
- Liability Issues: Determining responsibility in cases of AI-related errors.
Technical Limitations
Technical challenges can hinder the effectiveness of AI in telehealth:
- Connectivity Issues: Reliable internet access is necessary for telehealth services.
- Integration with Existing Systems: Ensuring compatibility with current healthcare infrastructure.
- User Adoption: Patients and providers may be hesitant to adopt new technologies.
Ethical Considerations
Ethical issues arise in AI-driven telehealth:
- Patient Autonomy: Ensuring patients retain control over their healthcare decisions.
- Consent: Obtaining informed consent for AI use in medical care.
- Human Oversight: Balancing automation with human expertise to prevent over-reliance on AI.
Future Prospects of AI in Telehealth
Advancements in AI Technology
Continued advancements in AI will enhance telehealth capabilities:
- Enhanced AI Algorithms: More accurate and reliable AI models for diagnosis and treatment.
- Edge Computing: Processing data locally on devices, reducing latency and improving real-time analysis.
- Integration with IoT: Connecting more health devices for comprehensive patient monitoring.
Personalized Medicine
AI will facilitate greater personalization in telehealth:
- Genomic Data Integration: Using genetic information to tailor treatments.
- Behavioral Insights: Analyzing lifestyle data for holistic care plans.
Global Collaboration
AI-powered telehealth can connect healthcare providers worldwide:
- Knowledge Sharing: Facilitating collaboration between medical professionals across borders.
- Remote Expertise: Providing access to specialists regardless of location.
Regulatory Evolution
Anticipated changes in regulations will support AI integration:
- Updated Guidelines: Establishing standards for AI use in telehealth.
- Incentives: Governments may offer incentives for adopting AI technologies.
Enhanced Patient Engagement
AI will empower patients to take an active role in their healthcare:
- Interactive Platforms: Engaging patients through AI-driven applications.
- Educational Resources: Providing personalized health education.
Strategies for Successful Implementation
Investing in Infrastructure
Building robust digital infrastructure is essential:
- High-Speed Internet: Ensuring reliable connectivity for telehealth services.
- Secure Data Systems: Implementing advanced cybersecurity measures.
Training Healthcare Professionals
Educating providers on AI tools enhances adoption:
- Technical Training: Familiarizing staff with AI applications.
- Ethical Awareness: Understanding ethical considerations in AI use.
Patient Education
Informing patients about AI benefits encourages engagement:
- Transparency: Explaining how AI impacts their care.
- Support Resources: Providing assistance in using telehealth platforms.
Collaboration with Tech Companies
Partnering with technology firms brings expertise:
- Custom Solutions: Developing AI tools tailored to specific healthcare needs.
- Innovation: Staying at the forefront of technological advancements.
Compliance and Ethics Framework
Establishing clear policies ensures responsible AI use:
- Data Governance: Implementing policies for data management and privacy.
- Ethical Guidelines: Defining acceptable AI practices in patient care.
Conclusion
Artificial Intelligence is profoundly transforming telehealth services by enhancing accessibility, efficiency, and personalization in healthcare delivery. Through AI-powered virtual assistants, remote monitoring, advanced diagnostics, and personalized treatment plans, telehealth is becoming more effective and patient-centric. While challenges such as data privacy, algorithmic bias, and regulatory hurdles exist, addressing these issues is crucial for the successful integration of AI into telehealth. As technology continues to evolve, AI will play an increasingly vital role in shaping the future of healthcare, making quality medical services accessible to all, regardless of location. Embracing AI in telehealth represents not just an innovation but a necessary step toward a more connected and efficient healthcare system.