How Machine Learning Enhances Predictive Maintenance in Transportation
How Machine Learning Enhances Predictive Maintenance in Transportation
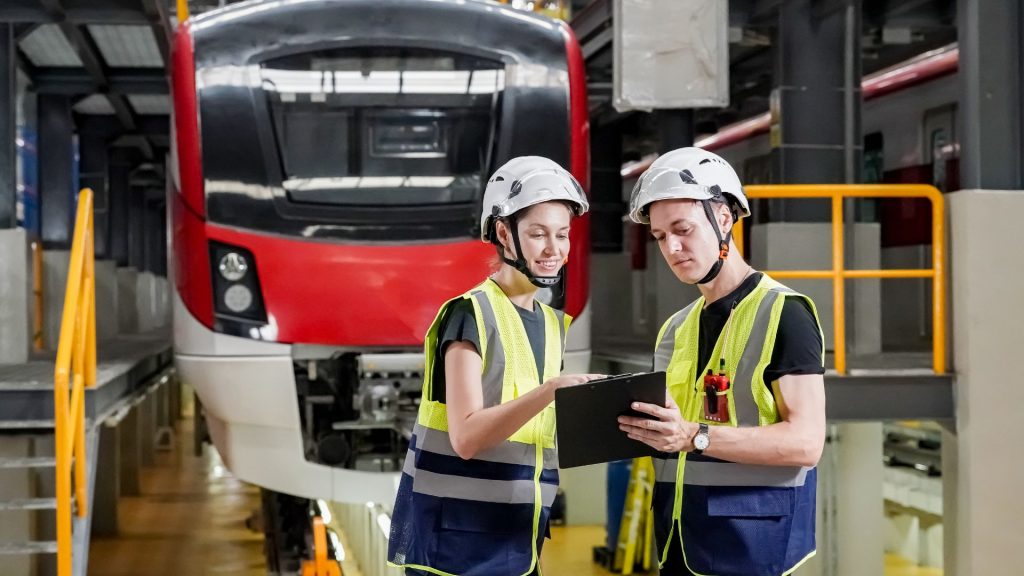
Introduction
The transportation industry is the backbone of the global economy, ensuring the smooth movement of goods and people across the world. As fleets and infrastructure age, maintenance becomes a critical aspect to ensure safety, reduce downtime, and maintain operational efficiency. Traditional maintenance strategies, such as reactive and scheduled maintenance, often lead to unexpected failures or unnecessary servicing. Machine Learning (ML) is revolutionizing maintenance strategies in transportation by enabling predictive maintenance. Predictive maintenance uses ML algorithms to predict when maintenance is required, thereby minimizing unplanned downtime, reducing costs, and improving safety. This article explores how ML enhances predictive maintenance in transportation, the techniques involved, applications, benefits, challenges, and real-world examples.
The Importance of Predictive Maintenance in Transportation
Challenges with Traditional Maintenance Strategies
Traditional maintenance approaches often fall short when it comes to efficiency and cost-effectiveness:
- Reactive Maintenance: Involves repairing equipment only after it has failed, leading to unexpected downtime, costly repairs, and potential safety hazards.
- Scheduled Maintenance: Maintenance performed at regular intervals, regardless of actual equipment condition, often results in unnecessary servicing and increased costs.
- Downtime Costs: Both reactive and scheduled maintenance can result in operational disruptions and revenue losses due to unplanned downtime.
The Predictive Maintenance Advantage
Predictive maintenance uses data-driven insights to determine the optimal time for maintenance:
- Condition-Based Maintenance: Equipment is serviced based on its current condition rather than a predetermined schedule, reducing unnecessary maintenance.
- Failure Prevention: Predictive maintenance helps identify potential failures before they occur, minimizing the risk of costly breakdowns and improving safety.
- Increased Asset Lifespan: By addressing issues proactively, predictive maintenance helps extend the lifespan of assets and reduces the need for replacements.
Machine Learning Techniques for Predictive Maintenance
Supervised Learning for Fault Detection
Supervised learning algorithms are used to predict potential faults based on historical data:
- Classification Models: ML models like Support Vector Machines (SVM) and Random Forests are used to classify the condition of components as “healthy” or “faulty” based on sensor data.
- Regression Models: Regression algorithms predict the remaining useful life (RUL) of components, allowing maintenance to be scheduled before a failure occurs.
- Neural Networks: Deep learning models are trained on historical maintenance data to identify patterns associated with faults, enhancing prediction accuracy.
Unsupervised Learning for Anomaly Detection
Unsupervised learning techniques are used to detect anomalies that indicate potential issues:
- Clustering Algorithms: Algorithms like K-Means and DBSCAN group similar data points, identifying outliers that may indicate abnormal equipment behavior.
- Autoencoders: Deep learning models are used to detect deviations from normal operating conditions, helping identify issues before they escalate.
Time Series Analysis
Time series analysis is essential for predictive maintenance in transportation:
- Recurrent Neural Networks (RNNs): RNNs, including Long Short-Term Memory (LSTM) networks, are used to analyze time-series data from sensors, predicting future equipment performance and potential failures.
- ARIMA Models: AutoRegressive Integrated Moving Average (ARIMA) models are used to analyze historical sensor data to predict future maintenance needs.
Reinforcement Learning for Maintenance Optimization
Reinforcement learning (RL) is used to optimize maintenance strategies:
- Optimal Maintenance Scheduling: RL algorithms learn optimal maintenance schedules that minimize downtime and costs while ensuring asset reliability.
- Dynamic Decision-Making: RL models adapt maintenance strategies based on real-time data, ensuring the most effective maintenance actions are taken.
Data Sources for ML-Based Predictive Maintenance
Sensor Data
Sensors are critical for collecting data used in ML-based predictive maintenance:
- Vibration Sensors: Measure vibrations in engines, wheels, and other mechanical components to identify early signs of wear or damage.
- Temperature Sensors: Monitor temperatures of critical components, such as brakes and engines, to detect overheating and potential failures.
- Pressure Sensors: Track pressure levels in hydraulic systems to ensure they remain within safe operating ranges.
Telematics Data
Telematics systems collect data on vehicle performance and usage:
- Engine Performance Metrics: Collect data on fuel consumption, engine speed, and other parameters that affect vehicle health.
- Vehicle Location and Usage: Monitor vehicle location, mileage, and driving conditions to provide context for maintenance predictions.
Historical Maintenance Records
Historical maintenance records are used to train ML models:
- Maintenance Logs: Records of past maintenance activities, including part replacements and repairs, are used to identify patterns and predict future maintenance needs.
- Failure History: Data on past failures is used to train models to recognize early warning signs of similar failures in the future.
Benefits of ML-Enhanced Predictive Maintenance in Transportation
Reduced Downtime
ML-based predictive maintenance minimizes unplanned downtime by identifying issues before they lead to equipment failure. This ensures that vehicles are serviced only when necessary, reducing the time spent out of operation.
Cost Savings
Predictive maintenance reduces maintenance costs by optimizing the timing of maintenance activities. By avoiding unnecessary maintenance and preventing costly breakdowns, transportation companies can save on labor, parts, and repair expenses.
Improved Safety
By predicting and preventing potential failures, ML enhances the safety of transportation operations. This is particularly important for public transportation and freight services, where equipment failures can lead to accidents and significant financial losses.
Increased Asset Lifespan
Proactive maintenance helps extend the lifespan of transportation assets, such as vehicles, engines, and critical components. By addressing issues early, predictive maintenance prevents wear and tear from escalating, preserving the overall health of assets.
Optimized Maintenance Scheduling
ML algorithms optimize maintenance schedules to minimize disruptions to operations. Maintenance activities can be planned during periods of low demand, reducing the impact on fleet availability and improving overall efficiency.
Data-Driven Decision-Making
ML-based predictive maintenance relies on data-driven insights, enabling transportation companies to make informed decisions about fleet management, resource allocation, and maintenance priorities. This helps improve operational efficiency and reduce costs.
Real-World Applications of ML in Predictive Maintenance
Deutsche Bahn (German Railways)
Deutsche Bahn has implemented ML-based predictive maintenance to improve the reliability of its rail services:
- Sensor Monitoring: Sensors installed on trains monitor critical components such as wheels, brakes, and engines. ML algorithms analyze this data to predict maintenance needs and prevent breakdowns.
- Reduced Downtime: Predictive maintenance has reduced the number of unexpected failures, minimizing service disruptions and improving passenger satisfaction.
Delta Airlines
Delta Airlines uses ML to enhance predictive maintenance for its fleet of aircraft:
- Engine Health Monitoring: ML models analyze engine performance data to detect anomalies and predict maintenance needs, ensuring engines are serviced before issues lead to in-flight failures.
- Optimized Maintenance Scheduling: Predictive maintenance has enabled Delta to optimize maintenance schedules, reducing aircraft downtime and improving operational efficiency.
UPS (United Parcel Service)
UPS has adopted ML-based predictive maintenance to improve the reliability of its delivery fleet:
- Telematics Data Analysis: ML algorithms analyze telematics data, including engine performance and driving conditions, to predict maintenance needs for delivery vehicles.
- Reduced Maintenance Costs: By predicting and addressing issues early, UPS has reduced maintenance costs and improved fleet availability for deliveries.
Ford Motor Company
Ford has implemented ML-driven predictive maintenance solutions for its connected vehicles:
- Connected Vehicle Platform: Ford’s connected vehicles collect data on engine performance, fuel consumption, and driving behavior. ML models analyze this data to predict maintenance needs and send alerts to vehicle owners.
- Customer Satisfaction: Predictive maintenance helps Ford customers maintain their vehicles proactively, reducing the likelihood of unexpected breakdowns and improving customer satisfaction.
Challenges of Using ML for Predictive Maintenance in Transportation
Data Quality and Availability
The accuracy of ML models depends on the quality and availability of data:
- Incomplete Data: Missing or incomplete sensor data can lead to inaccurate predictions and affect the reliability of predictive maintenance models.
- Data Consistency: Variability in data quality due to sensor malfunctions or data transmission issues can affect model performance.
Integration with Legacy Systems
Integrating ML solutions with existing maintenance systems can be challenging:
- Compatibility Issues: Ensuring that ML models are compatible with legacy systems may require significant adjustments and investment.
- Data Integration: Combining data from multiple sources, such as sensors, telematics, and maintenance logs, requires careful data integration and management.
High Initial Costs
Implementing ML-based predictive maintenance requires significant investment in hardware, software, and expertise:
- Sensor Installation: Installing sensors on existing assets can be costly, particularly for large fleets.
- Computational Resources: Training ML models requires powerful computational resources, which may be costly for small and medium-sized transportation companies.
Model Interpretability
Many ML models, especially deep learning models, are often considered “black boxes,” making it difficult to understand how they arrive at their predictions:
- Explainability: Providing transparency in ML predictions is crucial for gaining the trust of maintenance teams and decision-makers.
- Trust Issues: Lack of interpretability may lead to skepticism about the accuracy and reliability of predictive maintenance models.
Future Trends in ML-Enhanced Predictive Maintenance
Federated Learning for Collaborative Maintenance
Federated learning enables ML models to be trained across multiple organizations without sharing raw data:
- Data Privacy: Federated learning ensures data privacy while enabling collaboration between transportation companies to build more accurate predictive maintenance models.
- Improved Model Accuracy: Collaborative learning across large datasets can improve the accuracy of maintenance predictions for different types of vehicles and equipment.
Edge Computing for Real-Time Maintenance Predictions
Edge computing processes data closer to the source, enabling real-time maintenance predictions:
- Reduced Latency: Edge computing reduces latency by processing sensor data locally, allowing for faster predictions and immediate maintenance actions.
- Scalability: Edge computing enables predictive maintenance solutions to be scaled across large fleets without relying on centralized cloud servers.
Integration with Digital Twins
Digital twins, virtual replicas of physical assets, will enhance predictive maintenance:
- Virtual Simulation: Digital twins allow maintenance teams to simulate different maintenance scenarios and assess their impact on asset health.
- Improved Predictions: ML models integrated with digital twins can provide more accurate predictions by incorporating real-time data from both physical and virtual assets.
AI-Driven Autonomous Maintenance
AI-driven autonomous maintenance systems will take predictive maintenance to the next level:
- Automated Repairs: AI-powered robots and drones will be used to perform maintenance tasks autonomously, reducing the need for human intervention.
- Self-Learning Systems: ML models will continuously learn from new data, enabling autonomous maintenance systems to adapt and improve over time.
Explainable AI (XAI) for Predictive Maintenance
Explainable AI (XAI) will improve transparency and trust in ML-driven predictive maintenance:
- Informed Decision-Making: XAI will help maintenance teams understand how ML models make predictions, enabling more informed decision-making.
- Regulatory Compliance: Explainable models are essential for meeting regulatory requirements and ensuring accountability in maintenance operations.
Conclusion
Machine Learning is transforming predictive maintenance in transportation by enabling data-driven insights, reducing downtime, and improving safety. Through the use of supervised and unsupervised learning, time series analysis, and reinforcement learning, ML helps predict equipment failures, optimize maintenance schedules, and extend the lifespan of transportation assets. While challenges such as data quality, integration, and cost exist, the benefits of ML in predictive maintenance are clear. As ML continues to evolve, future trends such as federated learning, edge computing, digital twins, and autonomous maintenance will further enhance the capabilities of predictive maintenance, ensuring the reliability, efficiency, and sustainability of transportation operations. By embracing ML, transportation companies can achieve greater operational efficiency, reduce costs, and improve the safety and reliability of their services.