The Role of AI in Improving Environmental Sustainability
The Role of AI in Improving Environmental Sustainability
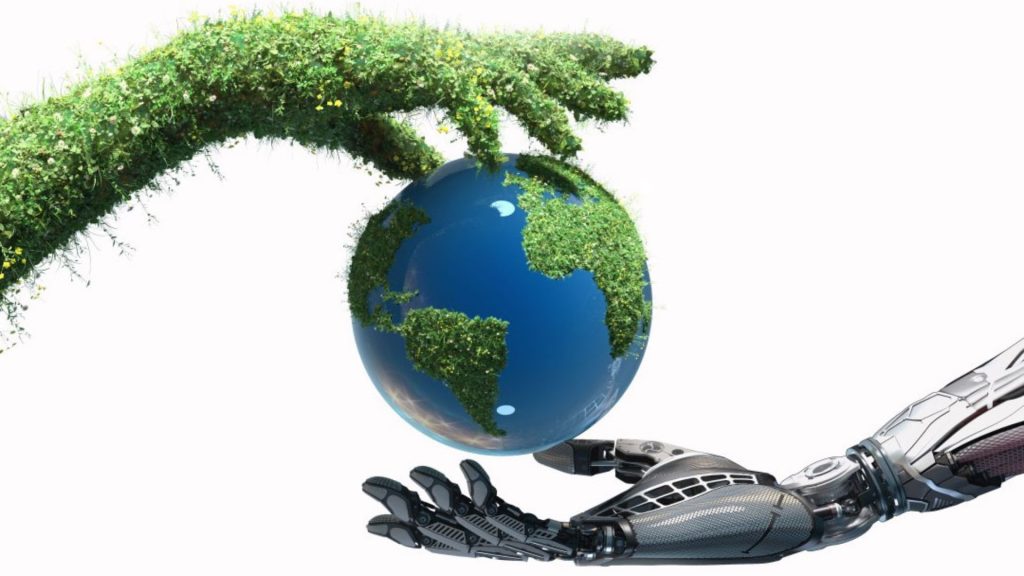
Introduction
Environmental sustainability is one of the most pressing challenges of our time, as societies and economies across the globe face the consequences of climate change, resource depletion, and pollution. Emerging technologies like Artificial Intelligence (AI) are offering promising solutions to address these challenges and improve environmental sustainability. AI’s ability to analyze large datasets, predict outcomes, and optimize processes has the potential to revolutionize how we tackle environmental issues. By enhancing energy efficiency, managing natural resources, reducing waste, and promoting sustainable practices, AI is playing a critical role in the fight for a greener future. This article explores how AI is improving environmental sustainability, the technologies involved, applications, benefits, challenges, and real-world examples.
AI Technologies and Techniques for Environmental Sustainability
Machine Learning and Predictive Analytics
Machine Learning (ML) and predictive analytics are key AI techniques used to support sustainability efforts:
- Data Analysis: ML algorithms analyze environmental data, such as temperature, emissions, and resource consumption, to identify patterns and predict trends.
- Predictive Maintenance: Predictive analytics is used to monitor equipment in energy and manufacturing sectors, ensuring they operate efficiently and reducing their environmental impact.
- Risk Assessment: ML models are used to predict natural disasters, such as floods and wildfires, helping to mitigate damage and reduce their impact on the environment.
Computer Vision for Environmental Monitoring
Computer vision, an AI technology that enables machines to interpret visual data, plays a significant role in environmental monitoring:
- Wildlife Monitoring: AI-powered drones and cameras monitor wildlife populations, track endangered species, and detect illegal poaching activities.
- Pollution Detection: Computer vision systems are used to identify pollutants in water bodies, detect oil spills, and monitor air quality.
- Forest Health Assessment: AI analyzes satellite images to monitor deforestation, identify diseased trees, and assess overall forest health.
Natural Language Processing (NLP) for Climate Research
NLP enables AI to process and analyze vast amounts of text data, making it a valuable tool for climate research:
- Research Analysis: NLP helps researchers analyze scientific literature on climate change, extract insights, and stay up-to-date with the latest findings.
- Public Awareness: NLP-based chatbots and virtual assistants help educate the public about sustainability practices and climate-related topics.
Optimization Algorithms for Energy Efficiency
AI optimization algorithms are used to improve energy efficiency in various sectors:
- Smart Grids: AI optimizes energy distribution within smart grids by predicting energy demand, managing supply, and integrating renewable energy sources.
- Building Automation: AI-powered building management systems control heating, ventilation, and lighting to reduce energy consumption and improve efficiency.
- Logistics Optimization: AI optimizes transportation routes to minimize fuel consumption, reducing greenhouse gas emissions in the logistics sector.
Reinforcement Learning for Resource Management
Reinforcement learning (RL) is used to manage resources and optimize sustainability efforts:
- Water Management: RL algorithms optimize water usage in agriculture, reducing waste and ensuring sustainable irrigation practices.
- Waste Reduction: AI models are used to optimize waste management processes, including sorting, recycling, and composting, to minimize landfill contributions.
AI Applications in Environmental Sustainability
Renewable Energy Optimization
AI is playing a significant role in optimizing the generation and distribution of renewable energy:
- Solar and Wind Energy Forecasting: AI models predict solar and wind energy output based on weather data, allowing energy operators to manage supply and demand more efficiently.
- Energy Storage Management: AI optimizes the use of energy storage systems by predicting energy generation and consumption patterns, reducing energy waste.
- Grid Integration: AI facilitates the integration of renewable energy sources into the grid by balancing fluctuations in energy production and consumption.
Climate Change Modeling and Prediction
AI is used to create climate models and predict the impacts of climate change:
- Climate Modeling: ML algorithms analyze historical climate data to create models that simulate future climate scenarios, helping researchers and policymakers make informed decisions.
- Disaster Prediction: AI predicts natural disasters, such as hurricanes, floods, and heatwaves, allowing governments to prepare and respond effectively.
Precision Agriculture
AI is revolutionizing agriculture by promoting sustainable farming practices:
- Crop Health Monitoring: AI-powered drones and sensors monitor crop health, detect diseases, and apply precise amounts of water and nutrients, reducing resource wastage.
- Irrigation Optimization: AI models optimize irrigation schedules based on weather data, soil moisture, and crop type, ensuring efficient water use.
- Yield Prediction: ML algorithms predict crop yields based on environmental conditions, helping farmers plan their harvests and make informed decisions.
Waste Management and Recycling
AI is improving waste management and recycling processes to reduce environmental pollution:
- Smart Waste Sorting: AI-powered robots sort recyclables from waste, increasing recycling efficiency and reducing contamination in recycling streams.
- Waste Collection Optimization: AI models optimize waste collection routes, reducing fuel consumption and minimizing the carbon footprint of waste collection vehicles.
Conservation Efforts
AI is supporting conservation initiatives aimed at preserving ecosystems and biodiversity:
- Wildlife Conservation: AI-powered cameras and drones monitor wildlife populations, track animal movement, and detect illegal activities such as poaching.
- Marine Conservation: AI analyzes underwater images and videos to monitor marine life and assess the health of coral reefs, helping to protect vulnerable ecosystems.
Benefits of AI in Environmental Sustainability
Data-Driven Decision Making
AI enables data-driven decision-making by analyzing large volumes of environmental data and providing actionable insights. This helps governments, organizations, and individuals make informed decisions to promote sustainability and mitigate environmental risks.
Improved Resource Efficiency
AI optimizes resource use, such as energy, water, and raw materials, reducing waste and minimizing the environmental impact. By promoting efficient resource management, AI contributes to sustainable practices in industries such as agriculture, energy, and manufacturing.
Real-Time Environmental Monitoring
AI systems provide real-time environmental monitoring, allowing for the early detection of pollution, deforestation, and other environmental issues. This enables timely interventions to mitigate damage and protect natural ecosystems.
Reduced Greenhouse Gas Emissions
By optimizing energy consumption, improving logistics, and promoting the use of renewable energy, AI helps reduce greenhouse gas emissions. This contributes to global efforts to combat climate change and achieve sustainability goals.
Cost Savings
AI-powered sustainability solutions lead to cost savings by improving efficiency and reducing waste. For example, AI-optimized energy systems can reduce electricity bills, while precision agriculture reduces costs associated with water and fertilizer use.
Enhanced Public Awareness and Engagement
AI-powered tools, such as chatbots and virtual assistants, help raise awareness about environmental sustainability and encourage individuals to adopt eco-friendly practices. By educating the public, AI promotes collective action towards a more sustainable future.
Real-World Examples of AI in Environmental Sustainability
Google’s Environmental Initiatives
Google uses AI to enhance its sustainability efforts across various domains:
- Carbon-Intelligent Computing: Google’s data centers use AI to shift workloads to times when renewable energy is most available, reducing their carbon footprint.
- AI for Wind Energy: Google’s DeepMind uses ML to predict wind energy output, allowing for better integration of wind power into the grid.
IBM’s Green Horizon Project
IBM’s Green Horizon project uses AI to address environmental challenges:
- Air Quality Monitoring: AI models analyze air quality data to provide real-time pollution forecasts, helping cities take proactive measures to improve air quality.
- Renewable Energy Forecasting: IBM’s AI tools predict solar and wind energy output, enabling more efficient use of renewable energy sources.
Microsoft’s AI for Earth Program
Microsoft’s AI for Earth program aims to address environmental challenges using AI:
- Land Use and Agriculture: AI tools analyze satellite imagery to monitor land use and promote sustainable agricultural practices.
- Biodiversity Conservation: AI models help track endangered species and assess ecosystem health, supporting conservation efforts.
Ocean Cleanup Project
The Ocean Cleanup project uses AI to address marine pollution:
- Trash Detection: AI-powered sensors detect plastic waste in the ocean, helping to guide cleanup efforts.
- Optimized Collection Routes: AI models optimize routes for collection vessels to maximize the amount of plastic waste removed from the ocean.
UN Global Pulse
The United Nations’ Global Pulse initiative uses AI to address environmental challenges:
- Disaster Response: AI models analyze satellite imagery to assess the impact of natural disasters and support response efforts.
- Environmental Monitoring: AI tools monitor environmental changes, such as deforestation and flooding, to inform policy and decision-making.
Challenges of Using AI for Environmental Sustainability
Data Quality and Availability
AI models rely on large volumes of high-quality data, but environmental data is often limited or inconsistent:
- Data Gaps: In some regions, there may be a lack of reliable environmental data, making it challenging to train AI models accurately.
- Data Privacy Concerns: Collecting environmental data from individuals and organizations can raise privacy concerns, limiting data availability.
High Energy Consumption of AI Models
Training large AI models requires significant computational resources, which can lead to increased energy consumption:
- Carbon Footprint: The energy used in training AI models can contribute to carbon emissions, potentially offsetting some of the environmental benefits of AI.
- Need for Green Computing: Developing energy-efficient AI models and using renewable energy for computation are essential to mitigate this challenge.
Algorithmic Bias
AI models can inherit biases from the data they are trained on, leading to unintended consequences:
- Bias in Environmental Predictions: Biased models may produce inaccurate predictions, affecting decision-making related to climate and resource management.
- Equity Concerns: AI-driven sustainability initiatives must consider the needs of vulnerable communities to avoid exacerbating inequalities.
Integration with Existing Systems
Integrating AI solutions into existing environmental management systems can be challenging:
- Compatibility Issues: Ensuring that AI models are compatible with existing infrastructure requires careful planning and investment.
- Technical Expertise: Implementing AI for environmental sustainability requires specialized knowledge, which may be lacking in certain regions or organizations.
Future Trends in AI for Environmental Sustainability
Federated Learning for Collaborative Sustainability
Federated learning allows AI models to be trained across multiple organizations without sharing raw data, promoting collaboration for sustainability efforts:
- Data Privacy: Federated learning ensures data privacy while enabling organizations to collaborate on building more accurate environmental models.
- Scalability: This approach supports large-scale data analysis without the need to centralize data, making it ideal for environmental applications.
AI and IoT Integration
The integration of AI with the Internet of Things (IoT) will enhance environmental monitoring and management:
- Smart Sensors: IoT sensors collect real-time environmental data, while AI analyzes this data to identify patterns and predict changes.
- Automated Interventions: AI-driven IoT systems will enable automated interventions, such as adjusting irrigation levels or activating pollution control measures.
AI-Driven Circular Economy
AI will play a crucial role in promoting a circular economy by optimizing resource use and minimizing waste:
- Product Lifecycle Management: AI models will optimize product lifecycles by predicting maintenance needs and recycling opportunities.
- Smart Manufacturing: AI will enable sustainable manufacturing practices by minimizing waste, optimizing energy use, and promoting the use of recycled materials.
AI for Sustainable Urban Planning
AI will be increasingly used to promote sustainability in urban areas:
- Traffic Management: AI models will optimize traffic flow, reducing congestion and emissions in cities.
- Green Infrastructure Planning: AI will help design green infrastructure, such as parks and green roofs, to enhance urban sustainability and improve quality of life.
Explainable AI (XAI) for Environmental Applications
Explainable AI (XAI) will improve transparency and trust in AI-driven sustainability initiatives:
- Informed Decision-Making: XAI will help stakeholders understand how AI models arrive at their predictions, enabling more informed decision-making.
- Regulatory Compliance: Explainable models are essential for meeting regulatory requirements and ensuring accountability in environmental management.
Conclusion
Artificial Intelligence is playing a pivotal role in improving environmental sustainability by enabling data-driven decision-making, optimizing resource use, and promoting efficient management of natural resources. AI technologies, such as machine learning, computer vision, and reinforcement learning, are being applied across various domains, including renewable energy optimization, climate modeling, precision agriculture, and waste management. Despite challenges related to data quality, energy consumption, and integration, the benefits of AI in sustainability are undeniable. As AI continues to evolve, future trends such as federated learning, AI-IoT integration, and AI-driven circular economy initiatives will further enhance the role of AI in creating a sustainable future. By harnessing the power of AI, we can address environmental challenges more effectively and work towards a greener, more sustainable planet for future generations.