How ML Optimizes Energy Consumption in Industries
How ML Optimizes Energy Consumption in Industries
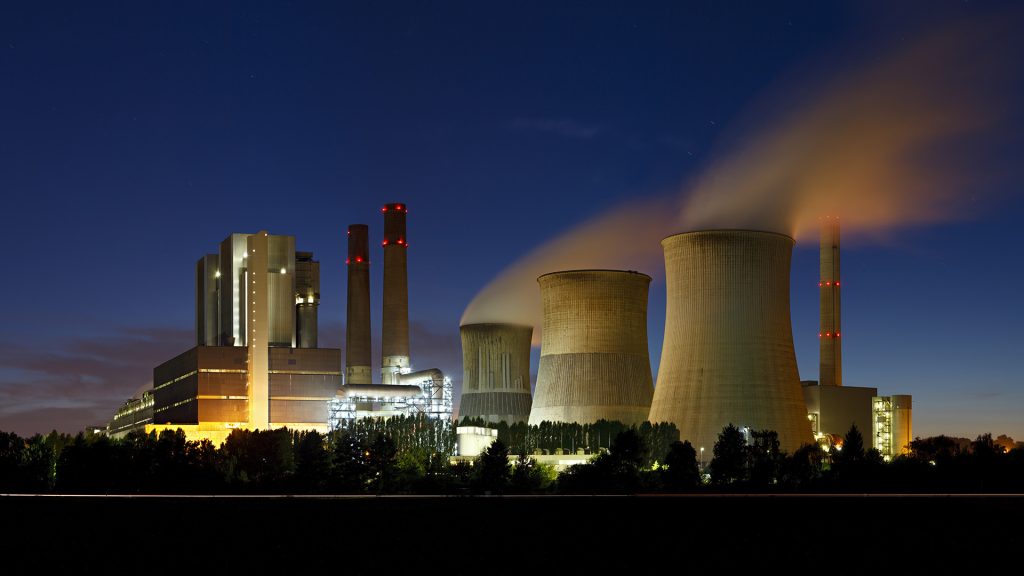
Machine Learning (ML) is revolutionizing how industries manage and optimize their energy consumption. By leveraging advanced algorithms and data analytics, ML can identify patterns, predict energy needs, and implement efficiency measures that reduce costs and environmental impact. This article explores the role of ML in optimizing energy consumption in industries, supported by real-world examples and detailed insights.
Table of Contents
- Introduction to Energy Consumption in Industries
- The Role of ML in Energy Optimization
- Key ML Techniques for Energy Optimization
- Applications of ML in Energy Optimization
- Real-World Examples of ML in Energy Optimization
- Challenges and Limitations
- Future Trends and Developments
- Conclusion
Introduction to Energy Consumption in Industries
Energy consumption in industries is a critical factor that affects operational costs and environmental sustainability. Industries consume a significant portion of global energy, making it essential to find ways to optimize energy usage and reduce waste. ML provides innovative solutions to these challenges by enhancing energy efficiency and promoting sustainable practices.
The Role of ML in Energy Optimization
Machine Learning is central to optimizing energy consumption in industries. By analyzing large datasets from various sources, ML models can identify patterns and inefficiencies, predict future energy needs, and suggest optimization strategies. These models continuously improve with more data, leading to more accurate and effective energy management solutions.
Key ML Techniques for Energy Optimization
Predictive Maintenance
Predictive maintenance uses ML algorithms to predict when equipment will fail or require maintenance. By analyzing historical data and real-time sensor information, ML can identify patterns indicating potential failures. This proactive approach allows industries to perform maintenance only when necessary, reducing downtime and energy waste associated with unexpected equipment failures.
Demand Forecasting
Demand forecasting involves using ML models to predict future energy needs based on historical data, weather patterns, and production schedules. Accurate demand forecasting helps industries manage energy resources more efficiently, reducing energy consumption during peak times and optimizing usage during off-peak periods.
Energy Management Systems
Energy Management Systems (EMS) equipped with ML capabilities can monitor, control, and optimize energy consumption in real-time. These systems use data from various sources, including sensors and meters, to provide actionable insights and automate energy-saving measures. ML algorithms can continuously analyze energy usage patterns, identify inefficiencies, and suggest improvements.
Applications of ML in Energy Optimization
Manufacturing
In manufacturing, ML can optimize energy consumption by monitoring production processes, identifying inefficiencies, and suggesting improvements. For example, ML algorithms can analyze data from production lines to identify patterns of energy waste and recommend adjustments to machinery settings or production schedules. This can lead to significant energy savings and reduced operational costs.
Example: Siemens uses ML to optimize energy consumption in its manufacturing facilities. By analyzing data from sensors and production systems, Siemens can identify inefficiencies and optimize machinery settings to reduce energy usage. This approach has led to significant energy savings and reduced operational costs. Siemens’ ML algorithms continuously monitor energy consumption and production data, providing real-time insights and actionable recommendations. By implementing these recommendations, Siemens has achieved substantial energy savings, demonstrating the potential of ML to drive efficiency in industrial settings. Read more.
Data Centers
Data centers are among the largest consumers of energy in the tech industry. ML can optimize energy usage in data centers by monitoring server loads, predicting demand, and dynamically allocating resources. For example, ML algorithms can predict when certain servers will be underutilized and adjust their power settings accordingly, reducing energy waste.
Example: Google uses ML to optimize energy consumption in its data centers. By analyzing historical data and real-time sensor information, Google’s ML algorithms can predict server loads and adjust cooling systems to operate more efficiently. This approach has resulted in a 40% reduction in energy consumption for cooling. Google’s data centers are among the most energy-efficient in the world, thanks to the use of ML. The algorithms continuously learn from data, allowing for ongoing improvements in efficiency and performance. This example highlights the significant impact that ML can have on reducing energy consumption in large-scale data centers. Read more.
Smart Grids
Smart grids use ML to manage and optimize the distribution of electricity. By analyzing data from smart meters, weather forecasts, and energy usage patterns, ML can predict demand and optimize the supply of electricity. This helps utilities balance load, reduce energy waste, and integrate renewable energy sources more effectively.
Example: IBM’s Watson uses ML to optimize energy distribution in smart grids. By analyzing data from various sources, Watson can predict energy demand and optimize the supply of electricity. This helps utilities balance load, reduce energy waste, and integrate renewable energy sources more effectively. Watson’s ML capabilities allow for real-time adjustments to energy distribution, ensuring that supply meets demand efficiently. This has led to improved reliability and reduced costs for utilities, showcasing the power of ML in transforming energy management in the utility sector. Read more.
HVAC Systems
Heating, Ventilation, and Air Conditioning (HVAC) systems are major energy consumers in buildings. ML can optimize HVAC systems by analyzing data from sensors, weather forecasts, and occupancy patterns. This allows for real-time adjustments to temperature settings and airflow, reducing energy consumption while maintaining comfort levels.
Example: Honeywell uses ML to optimize HVAC systems in commercial buildings. By analyzing data from sensors and weather forecasts, Honeywell’s ML algorithms can adjust temperature settings and airflow in real-time, reducing energy consumption while maintaining comfort levels. This approach has led to significant energy savings in commercial buildings. Honeywell’s ML-driven HVAC solutions provide continuous monitoring and adjustments, ensuring optimal performance and energy efficiency. The integration of ML in HVAC systems demonstrates the potential for substantial energy savings in building management. Read more.
Real-World Examples of ML in Energy Optimization
1. Siemens – Manufacturing Energy Optimization
Siemens uses ML to optimize energy consumption in its manufacturing facilities. By analyzing data from sensors and production systems, Siemens can identify inefficiencies and optimize machinery settings to reduce energy usage. This approach has led to significant energy savings and reduced operational costs. Siemens’ ML algorithms continuously monitor energy consumption and production data, providing real-time insights and actionable recommendations. By implementing these recommendations, Siemens has achieved substantial energy savings, demonstrating the potential of ML to drive efficiency in industrial settings. Read more.
2. Google – Data Center Energy Efficiency
Google uses ML to optimize energy consumption in its data centers. By analyzing historical data and real-time sensor information, Google’s ML algorithms can predict server loads and adjust cooling systems to operate more efficiently. This approach has resulted in a 40% reduction in energy consumption for cooling. Google’s data centers are among the most energy-efficient in the world, thanks to the use of ML. The algorithms continuously learn from data, allowing for ongoing improvements in efficiency and performance. This example highlights the significant impact that ML can have on reducing energy consumption in large-scale data centers. Read more.
3. IBM Watson – Smart Grid Management
IBM’s Watson uses ML to optimize energy distribution in smart grids. By analyzing data from various sources, Watson can predict energy demand and optimize the supply of electricity. This helps utilities balance load, reduce energy waste, and integrate renewable energy sources more effectively. Watson’s ML capabilities allow for real-time adjustments to energy distribution, ensuring that supply meets demand efficiently. This has led to improved reliability and reduced costs for utilities, showcasing the power of ML in transforming energy management in the utility sector. Read more.
4. Honeywell – HVAC System Optimization
Honeywell uses ML to optimize HVAC systems in commercial buildings. By analyzing data from sensors and weather forecasts, Honeywell’s ML algorithms can adjust temperature settings and airflow in real-time, reducing energy consumption while maintaining comfort levels. This approach has led to significant energy savings in commercial buildings. Honeywell’s ML-driven HVAC solutions provide continuous monitoring and adjustments, ensuring optimal performance and energy efficiency. The integration of ML in HVAC systems demonstrates the potential for substantial energy savings in building management. Read more.
5. Tesla – Manufacturing Energy Efficiency
Tesla uses ML to optimize energy consumption in its Gigafactories. By analyzing data from production processes and equipment, Tesla’s ML algorithms can identify inefficiencies and suggest improvements. This has led to significant energy savings and increased production efficiency. Tesla’s ML-driven energy management system continuously learns from data, allowing for ongoing optimization of energy usage. This example highlights the role of ML in driving efficiency and sustainability in advanced manufacturing facilities. Read more.
6. Enel – Renewable Energy Integration
Enel, a global energy company, uses ML to optimize the integration of renewable energy sources into the grid. By analyzing weather data, energy production, and consumption patterns, Enel’s ML algorithms can predict energy availability and demand, ensuring efficient use of renewable energy. This approach has improved the reliability and efficiency of renewable energy integration, reducing reliance on fossil fuels. Enel’s ML-driven energy management system supports the transition to a more sustainable energy future. Read more.
7. Schneider Electric – Industrial Energy Management
Schneider Electric uses ML to optimize energy consumption in industrial facilities. By analyzing data from sensors and production systems, Schneider Electric’s ML algorithms can identify inefficiencies and suggest improvements. This has led to significant energy savings and increased operational efficiency. Schneider Electric’s ML-driven energy management solutions provide real-time insights and actionable recommendations, helping industries achieve their energy efficiency goals. Read more.
8. Intel – Data Center Energy Optimization
Intel uses ML to optimize energy consumption in its data centers. By analyzing data from servers and cooling systems, Intel’s ML algorithms can predict demand and adjust energy usage in real-time. This approach has resulted in significant energy savings and improved operational efficiency. Intel’s ML-driven energy management system continuously learns from data, enabling ongoing optimization of energy usage. This example demonstrates the impact of ML on reducing energy consumption in high-performance computing environments. Read more.
9. General Electric – Smart Grid Optimization
General Electric (GE) uses ML to optimize the operation of smart grids. By analyzing data from smart meters, weather forecasts, and energy usage patterns, GE’s ML algorithms can predict demand and optimize energy distribution. This helps utilities balance load, reduce energy waste, and improve grid reliability. GE’s ML-driven smart grid solutions support the integration of renewable energy sources, enhancing the sustainability of the energy supply. Read more.
10. Microsoft – Campus Energy Management
Microsoft uses ML to optimize energy consumption across its campus buildings. By analyzing data from sensors, weather forecasts, and occupancy patterns, Microsoft’s ML algorithms can adjust lighting, heating, and cooling systems in real-time. This approach has led to significant energy savings and improved comfort levels for building occupants. Microsoft’s ML-driven energy management system provides continuous monitoring and adjustments, ensuring optimal performance and energy efficiency. Read more.
Challenges and Limitations
Data Quality and Availability
ML systems require large volumes of high-quality data to function effectively. Ensuring the availability and accuracy of data can be challenging, particularly in industries with outdated infrastructure or limited data collection capabilities.
Integration with Existing Systems
Integrating ML solutions with existing systems and processes can be complex and costly. Industries may need to invest in new technologies and infrastructure to support ML implementation.
Cost and Infrastructure
Implementing ML solutions for energy optimization can be expensive and require significant infrastructure investments. Smaller companies may face challenges in adopting these technologies due to financial constraints.
Future Trends and Developments
Enhanced Predictive Analytics
Advancements in predictive analytics will enable more accurate demand forecasting, equipment maintenance, and energy management, leading to greater efficiency and cost savings.
Integration with Renewable Energy
Future ML solutions will increasingly integrate with renewable energy sources, optimizing the use of sustainable energy and reducing reliance on fossil fuels.
Autonomous Energy Management Systems
Autonomous energy management systems powered by ML will provide real-time monitoring and optimization of energy usage, reducing human intervention and enhancing efficiency.
Conclusion
Machine Learning is transforming energy consumption optimization in industries by providing advanced tools for monitoring, prediction, and optimization. From manufacturing and data centers to smart grids and HVAC systems, ML is helping industries reduce energy consumption, lower costs, and promote sustainability. As technology continues to evolve, the role of ML in energy management will only grow, offering innovative solutions to global energy challenges.
For more insights and updates on the latest in ML and energy optimization, visit TGI.