AI and the Digital Transformation: How It’s Reshaping Industries
AI and the Digital Transformation: How It’s Reshaping Industries
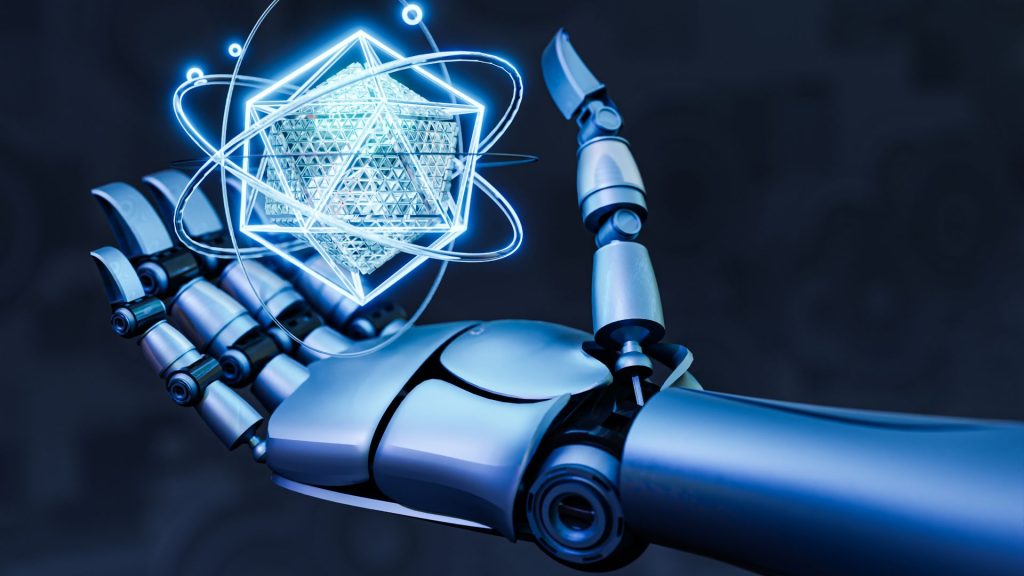
Introduction
Artificial Intelligence (AI) has emerged as one of the most transformative technologies of the 21st century. Its integration into various industries is driving a digital transformation that is reshaping the way businesses operate, compete, and deliver value to customers. From healthcare to finance, manufacturing to retail, AI is not just a buzzword but a fundamental force that is redefining the future of work, innovation, and economic growth.
This article delves into the profound impact of AI on digital transformation across industries. We will explore how AI is enabling businesses to automate processes, enhance decision-making, and create personalized customer experiences. Additionally, we will examine the challenges and opportunities that come with AI adoption, as well as the ethical considerations that must be addressed to ensure responsible AI deployment.
The Role of AI in Digital Transformation
Digital transformation refers to the integration of digital technologies into all aspects of a business, fundamentally changing how organizations operate and deliver value to customers. AI is at the heart of this transformation, acting as a catalyst that accelerates innovation and efficiency.
1. Automation and Efficiency
One of the most significant contributions of AI to digital transformation is automation. AI-powered systems can perform repetitive tasks with greater accuracy and speed than humans, freeing up employees to focus on more strategic and creative activities. For example, in manufacturing, AI-driven robots can assemble products with precision, while in customer service, chatbots can handle routine inquiries, reducing the need for human intervention.
Automation not only improves efficiency but also reduces operational costs. By automating processes, businesses can minimize errors, optimize resource allocation, and increase productivity. This is particularly important in industries such as logistics, where AI-powered systems can optimize supply chain operations, reducing delivery times and costs.
2. Data-Driven Decision Making
AI excels at analyzing vast amounts of data and extracting actionable insights. In the era of big data, businesses are inundated with information from various sources, including social media, sensors, and transaction records. AI algorithms can process this data in real-time, identifying patterns and trends that would be impossible for humans to detect.
This capability is transforming decision-making processes across industries. In finance, for example, AI-powered analytics tools can assess market trends and predict stock movements, enabling traders to make informed decisions. In healthcare, AI can analyze patient data to identify potential health risks and recommend personalized treatment plans.
3. Personalization and Customer Experience
AI is revolutionizing the way businesses interact with their customers. By leveraging AI, companies can deliver personalized experiences that cater to individual preferences and needs. For instance, e-commerce platforms use AI algorithms to recommend products based on a customer’s browsing history and purchase behavior. Similarly, streaming services like Netflix and Spotify use AI to curate content that aligns with users’ tastes.
Personalization not only enhances customer satisfaction but also drives customer loyalty and retention. By understanding and anticipating customer needs, businesses can create more meaningful and engaging interactions, ultimately leading to increased sales and revenue.
4. Innovation and New Business Models
AI is not just improving existing processes; it is also enabling the creation of entirely new business models. For example, the rise of AI-powered platforms like Uber and Airbnb has disrupted traditional industries by connecting service providers directly with consumers. These platforms leverage AI to match supply with demand, optimize pricing, and enhance user experiences.
In addition, AI is driving innovation in areas such as autonomous vehicles, smart cities, and personalized medicine. These advancements are not only creating new opportunities for businesses but also addressing some of the world’s most pressing challenges, such as climate change, urbanization, and healthcare access.
AI in Key Industries
The impact of AI on digital transformation is evident across various industries. Below, we explore how AI is reshaping some of the most prominent sectors.
1. Healthcare
AI is transforming healthcare by improving diagnostics, treatment, and patient care. AI-powered tools can analyze medical images, such as X-rays and MRIs, with remarkable accuracy, aiding in the early detection of diseases like cancer. Additionally, AI algorithms can predict patient outcomes and recommend personalized treatment plans based on individual health data.
Telemedicine, powered by AI, is also gaining traction, enabling patients to consult with healthcare providers remotely. This is particularly beneficial in rural or underserved areas where access to medical facilities is limited. Furthermore, AI is being used to streamline administrative tasks, such as scheduling appointments and managing medical records, allowing healthcare professionals to focus on patient care.
2. Finance
The financial industry is leveraging AI to enhance risk management, fraud detection, and customer service. AI algorithms can analyze transaction data in real-time to identify suspicious activities and prevent fraud. In addition, AI-powered chatbots are being used to provide customers with instant support, answering queries and assisting with transactions.
AI is also revolutionizing investment management. Robo-advisors, which use AI to create and manage investment portfolios, are becoming increasingly popular among investors. These platforms offer personalized investment advice based on an individual’s financial goals and risk tolerance, making wealth management more accessible and affordable.
3. Retail
In the retail sector, AI is driving a shift towards more personalized and efficient shopping experiences. AI-powered recommendation engines analyze customer data to suggest products that align with individual preferences, increasing the likelihood of purchase. Additionally, AI is being used to optimize inventory management, ensuring that popular items are always in stock while reducing excess inventory.
Retailers are also using AI to enhance the in-store experience. For example, smart mirrors in clothing stores can suggest outfits based on a customer’s style and body type, while AI-powered checkout systems can reduce wait times by automating the payment process.
4. Manufacturing
AI is revolutionizing manufacturing by enabling the creation of smart factories. These facilities use AI-powered sensors and machines to monitor and optimize production processes in real-time. Predictive maintenance, powered by AI, can detect equipment failures before they occur, reducing downtime and maintenance costs.
In addition, AI is being used to improve quality control. AI-powered vision systems can inspect products for defects with greater accuracy than human inspectors, ensuring that only high-quality products reach the market. Furthermore, AI is enabling the customization of products at scale, allowing manufacturers to meet the unique needs of individual customers.
5. Transportation and Logistics
AI is transforming the transportation and logistics industry by optimizing routes, reducing fuel consumption, and improving delivery times. AI-powered systems can analyze traffic data in real-time to identify the most efficient routes for delivery vehicles. In addition, AI is being used to predict demand for transportation services, enabling companies to allocate resources more effectively.
The rise of autonomous vehicles is another significant development in this sector. Self-driving trucks and delivery drones, powered by AI, have the potential to revolutionize the logistics industry by reducing labor costs and increasing efficiency. Furthermore, AI is being used to enhance the safety of transportation systems by predicting and preventing accidents.
6. Energy
AI is playing a crucial role in the transition to a more sustainable energy future. AI-powered systems can optimize the operation of renewable energy sources, such as wind and solar, by predicting weather patterns and adjusting energy production accordingly. In addition, AI is being used to improve energy efficiency in buildings and industrial facilities, reducing energy consumption and carbon emissions.
AI is also enabling the development of smart grids, which use real-time data to balance energy supply and demand. This not only improves the reliability of the energy system but also facilitates the integration of renewable energy sources into the grid.
Challenges and Opportunities
While the benefits of AI in digital transformation are undeniable, there are also challenges that must be addressed to fully realize its potential.
1. Data Privacy and Security
The widespread adoption of AI raises concerns about data privacy and security. AI systems rely on vast amounts of data to function effectively, and this data often includes sensitive information about individuals and organizations. Ensuring that this data is collected, stored, and used in a secure and ethical manner is a critical challenge.
Businesses must implement robust data protection measures, such as encryption and access controls, to safeguard sensitive information. In addition, they must comply with data privacy regulations, such as the General Data Protection Regulation (GDPR) in the European Union, to avoid legal and reputational risks.
2. Ethical Considerations
The use of AI also raises ethical questions, particularly in areas such as bias and fairness. AI algorithms are only as good as the data they are trained on, and if this data contains biases, the AI system may perpetuate or even amplify these biases. For example, AI-powered hiring tools may discriminate against certain groups if the training data is biased.
To address these concerns, businesses must ensure that their AI systems are transparent and accountable. This includes conducting regular audits of AI algorithms to identify and mitigate biases, as well as involving diverse teams in the development and deployment of AI systems.
3. Workforce Displacement
The automation of tasks through AI has the potential to displace workers, particularly in industries that rely heavily on routine and repetitive tasks. While AI can create new job opportunities, there is a risk that the benefits of AI will not be evenly distributed, leading to increased inequality.
To mitigate this risk, businesses must invest in reskilling and upskilling their workforce to prepare employees for the jobs of the future. Governments and educational institutions also have a role to play in ensuring that workers have access to the training and education they need to thrive in an AI-driven economy.
4. Regulation and Governance
The rapid advancement of AI technology has outpaced the development of regulatory frameworks, creating a need for clear and consistent guidelines. Governments and industry bodies must work together to establish standards and best practices for the ethical and responsible use of AI.
This includes addressing issues such as algorithmic transparency, accountability, and the use of AI in critical applications, such as healthcare and criminal justice. By creating a regulatory environment that fosters innovation while protecting the public interest, we can ensure that AI is used for the benefit of all.
The Future of AI and Digital Transformation
As AI continues to evolve, its impact on digital transformation will only grow. In the coming years, we can expect to see even more innovative applications of AI across industries, as well as the emergence of new technologies that build on the foundation of AI.
1. AI and the Internet of Things (IoT)
The integration of AI with the Internet of Things (IoT) is poised to create a new wave of innovation. IoT devices generate vast amounts of data, and AI can analyze this data to provide insights and automate processes. For example, in smart homes, AI-powered IoT devices can learn user preferences and adjust settings accordingly, creating a more comfortable and energy-efficient living environment.
In industrial settings, the combination of AI and IoT can enable predictive maintenance, optimize supply chains, and improve operational efficiency. This convergence of technologies has the potential to transform industries and create new business opportunities.
2. AI and 5G
The rollout of 5G networks is another development that will amplify the impact of AI. 5G offers faster data speeds and lower latency, enabling real-time communication between devices and AI systems. This will be particularly important in applications such as autonomous vehicles, where split-second decisions can mean the difference between life and death.
In addition, 5G will enable the widespread adoption of edge computing, where data is processed closer to the source rather than being sent to a centralized data center. This will allow AI algorithms to operate more efficiently and respond more quickly to changing conditions.
3. AI and Quantum Computing
Quantum computing is still in its early stages, but it has the potential to revolutionize AI by solving complex problems that are currently beyond the reach of classical computers. Quantum computers can process vast amounts of data simultaneously, enabling AI algorithms to perform tasks such as drug discovery, financial modeling, and climate prediction with unprecedented speed and accuracy.
While quantum computing is not yet widely available, ongoing research and development are bringing us closer to realizing its potential. As quantum computing matures, it will open up new possibilities for AI and digital transformation.
4. AI and Human Collaboration
The future of AI is not about replacing humans but augmenting human capabilities. AI has the potential to enhance human creativity, decision-making, and problem-solving by providing insights and automating routine tasks. For example, AI-powered tools can assist doctors in diagnosing diseases, help engineers design more efficient systems, and enable artists to create new forms of expression.
By fostering collaboration between humans and AI, we can unlock new levels of innovation and productivity. This requires a shift in mindset, where AI is seen as a tool that empowers humans rather than a threat to jobs and livelihoods.
Conclusion
AI is a driving force behind the digital transformation that is reshaping industries and redefining the way we live and work. From automation and data-driven decision-making to personalization and innovation, AI is enabling businesses to operate more efficiently, deliver better customer experiences, and create new opportunities for growth.
However, the adoption of AI also comes with challenges, including data privacy concerns, ethical considerations, and the potential for workforce displacement. To fully realize the benefits of AI, businesses, governments, and society as a whole must work together to address these challenges and ensure that AI is used responsibly and ethically.
As we look to the future, the integration of AI with other emerging technologies, such as IoT, 5G, and quantum computing, will open up new possibilities for innovation and transformation. By embracing AI and fostering collaboration between humans and machines, we can create a future that is more efficient, sustainable, and inclusive.