The Role of Machine Learning in Optimizing Financial Portfolios
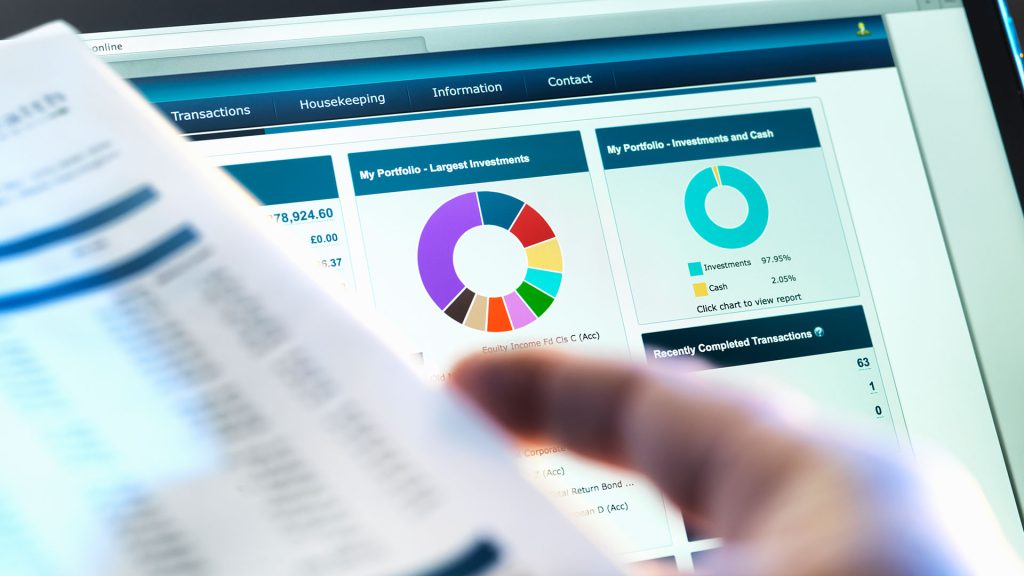
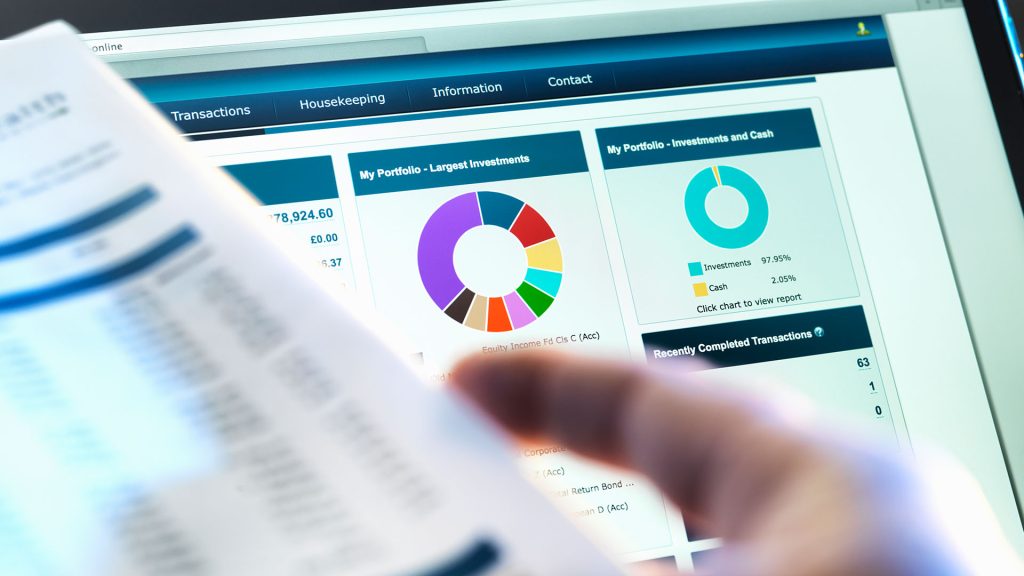
In the rapidly evolving world of finance, machine learning (ML) is emerging as a powerful tool for optimizing financial portfolios. By leveraging advanced algorithms and vast amounts of data, ML is transforming the way investment strategies are developed, tested, and implemented. This article explores the various ways ML is reshaping portfolio optimization, enhancing decision-making processes, and driving better investment outcomes.
Table of Contents
- Introduction to Machine Learning in Finance
- Benefits of ML in Portfolio Optimization
- Key Machine Learning Techniques in Portfolio Optimization
- Real-World Applications and Case Studies
- Challenges and Ethical Considerations
- Future Trends and Developments
- Conclusion
Introduction to Machine Learning in Finance
Machine learning, a subset of artificial intelligence, involves the use of algorithms and statistical models to perform specific tasks without explicit instructions. In finance, ML is employed to analyze vast datasets, identify patterns, and make predictions, which are crucial for portfolio management. By integrating ML into financial strategies, investors can enhance their decision-making processes and achieve more robust portfolio optimization.
Benefits of ML in Portfolio Optimization
Enhanced Data Analysis
ML algorithms can process and analyze large volumes of data at unprecedented speeds. This capability allows investors to consider a broader range of variables and datasets, including historical prices, economic indicators, and alternative data sources such as social media sentiment and news articles.
Example: Investment firms like Two Sigma use ML to analyze vast datasets to identify investment opportunities and optimize portfolios. Read more.
Risk Management
ML models can assess and manage risk more effectively by identifying patterns and correlations that traditional methods might overlook. These models can predict potential market downturns and adjust portfolios accordingly to minimize losses.
Example: JPMorgan’s LOXM trading algorithm uses ML to manage risk and improve trade execution. Read more.
Predictive Analytics
Predictive analytics, powered by ML, enables investors to forecast market trends and asset price movements. By anticipating changes in the market, investors can make proactive adjustments to their portfolios, optimizing returns and reducing risk.
Example: Goldman Sachs employs ML for predictive analytics in its trading and investment strategies. Read more.
Algorithmic Trading
Algorithmic trading involves using ML algorithms to execute trades based on predefined criteria. These algorithms can analyze market conditions in real-time and execute trades at optimal prices, significantly enhancing trading efficiency.
Example: Quantitative hedge funds like Renaissance Technologies utilize ML algorithms for high-frequency trading. Read more.
Key Machine Learning Techniques in Portfolio Optimization
Regression Analysis
Regression analysis is used to identify relationships between variables and predict future trends. In finance, it helps in forecasting asset prices and returns based on historical data.
Example: Analysts at BlackRock use regression models to forecast economic indicators and adjust portfolio allocations. Read more.
Clustering
Clustering involves grouping similar data points together. In portfolio optimization, clustering can identify groups of assets with similar characteristics, aiding in diversification and risk management.
Example: Betterment uses clustering algorithms to categorize assets and optimize portfolio diversification. Read more.
Neural Networks
Neural networks, modeled after the human brain, are capable of recognizing complex patterns and making accurate predictions. They are particularly useful in analyzing non-linear relationships in financial data.
Example: DeepMind, a subsidiary of Alphabet Inc., applies neural networks to model financial markets and develop investment strategies. Read more.
Reinforcement Learning
Reinforcement learning involves training algorithms to make decisions by rewarding them for desirable outcomes. In portfolio management, it can optimize investment strategies by continuously learning from market conditions.
Example: Financial firms like UBS are exploring reinforcement learning to enhance their trading algorithms. Read more.
Real-World Applications and Case Studies
BlackRock’s Aladdin
BlackRock, the world’s largest asset manager, uses an ML-powered platform called Aladdin to manage risk and optimize portfolios. Aladdin analyzes market data, forecasts economic conditions, and provides actionable insights to portfolio managers.
Example: Aladdin’s ML algorithms help BlackRock manage over $21 trillion in assets. Read more.
Betterment’s Robo-Advisors
Betterment, a leading robo-advisor, uses ML algorithms to provide personalized investment advice and portfolio management services. These algorithms assess an individual’s risk tolerance, financial goals, and market conditions to create optimized investment portfolios.
Example: Betterment manages over $22 billion in assets using ML-driven strategies. Read more.
Two Sigma’s Quantitative Research
Two Sigma, a quantitative hedge fund, employs ML to analyze market data and develop trading strategies. By leveraging advanced ML techniques, Two Sigma has consistently outperformed traditional hedge funds.
Example: Two Sigma’s ML models process petabytes of data daily to identify trading opportunities. Read more.
Challenges and Ethical Considerations
Data Quality and Availability
High-quality data is essential for accurate ML models. However, obtaining clean and comprehensive financial data can be challenging. Data availability and integrity issues can impact the performance of ML algorithms.
Model Interpretability
ML models, especially deep learning algorithms, can be complex and difficult to interpret. Ensuring that portfolio managers understand and trust these models is crucial for their successful implementation.
Ethical Concerns
The use of ML in finance raises ethical questions regarding transparency, fairness, and accountability. Ensuring that ML algorithms do not reinforce biases or make unethical investment decisions is a significant challenge.
Future Trends and Developments
As ML technology continues to evolve, its impact on financial portfolio optimization will likely grow. Future trends may include greater integration of alternative data sources, advancements in explainable AI, and the development of more sophisticated ML algorithms tailored to specific financial markets.
Conclusion
Machine learning is revolutionizing the field of portfolio optimization, offering new tools and techniques for enhancing investment strategies. By leveraging ML, investors can gain deeper insights, manage risks more effectively, and achieve better financial outcomes. As the technology continues to advance, its role in finance will only become more significant, paving the way for smarter, more efficient investment practices.
For more insights and updates on the latest in ML and finance, visit TGI.